Incentive-based modeling and inference of attacker intent, objectives, and strategies
以智能网联汽车为载体的汽车强国战略顶层设计

052专题研究 以智能网联汽车为载体的汽车强国战略顶层设计以智能网联汽车为载体的汽车强国战略顶层设计Strategic Analysis on Establishing an AutomobilePower in China Based on Intelligent &Connected Vehicles边明远,李克强(清华大学汽车工程系,北京 100084)Bian Mingyuan, Li Keqiang(Department of Automotive Engineering, Tsinghua University, Beijing 100084, China)摘要:智能网联汽车是以信息技术为代表的新科技变革趋势下产生的一种新兴产业形态。
由于体现为跨行业多领域新技术的深度交叉融合,智能网联汽车的产业化发展必定能带动相关行业的协同进步,从而推动中国制造业的转型升级,实现我国由汽车大国向汽车强国的转变。
本文阐述了建设汽车强国的本质内涵以及发展智能网联汽车对建设汽车强国的意义,分析了中国发展智能网联汽车具备的基础和面临的问题,并提出了以智能网联汽车为载体的建设汽车强国战略的顶层设计方案。
关键词:智能网联汽车;汽车强国;产业转型升级;顶层设计中图分类号:F420 文献标识码:AAbstract: The intelligent and connected vehicle (ICV), a trendy and promising industry form, has evolved with the development of information technology. Benefiting from the disciplinary crossing and integration of emerging technologies of various industries and fields, related industries will be witnessing a collaborative growth through the industrialization of ICVs. China, as the most productive nation in terms of automobiles in the world, desires to promote industrial transformation and upgradation, thus becoming one of the most powerful nations in the automobile industry. In this study, the connotation and significance of establishing an automobile power is illustrated, the current conditions and future challenges in the development of ICV that China faces are analyzed, and a top-level strate-gic design is proposed.Keywords: intelligent & connected vehicle (ICV); automobile power; industrial transformation and upgrading; top-level design一、前言当前,全球汽车产业正处于深度变革时期,作为汽车与信息技术两大产业创新融合的代表,智能网联汽车是新一轮科技革命和产业变革背景下的新生事物。
嵌入误分类代价和拒识代价的二元分类算法
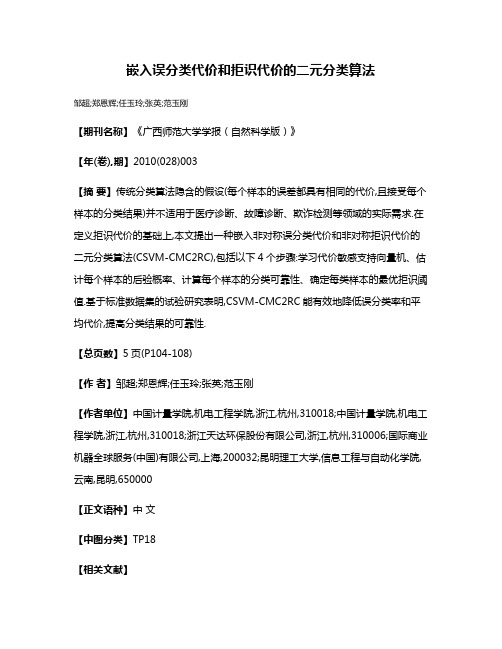
嵌入误分类代价和拒识代价的二元分类算法
邹超;郑恩辉;任玉玲;张英;范玉刚
【期刊名称】《广西师范大学学报(自然科学版)》
【年(卷),期】2010(028)003
【摘要】传统分类算法隐含的假设(每个样本的误差都具有相同的代价,且接受每个样本的分类结果)并不适用于医疗诊断、故障诊断、欺诈检测等领域的实际需求.在定义拒识代价的基础上,本文提出一种嵌入非对称误分类代价和非对称拒识代价的二元分类算法(CSVM-CMC2RC),包括以下4个步骤:学习代价敏感支持向量机、估计每个样本的后验概率、计算每个样本的分类可靠性、确定每类样本的最优拒识阈值.基于标准数据集的试验研究表明,CSVM-CMC2RC能有效地降低误分类率和平均代价,提高分类结果的可靠性.
【总页数】5页(P104-108)
【作者】邹超;郑恩辉;任玉玲;张英;范玉刚
【作者单位】中国计量学院,机电工程学院,浙江,杭州,310018;中国计量学院,机电工程学院,浙江,杭州,310018;浙江天达环保股份有限公司,浙江,杭州,310006;国际商业机器全球服务(中国)有限公司,上海,200032;昆明理工大学,信息工程与自动化学院,云南,昆明,650000
【正文语种】中文
【中图分类】TP18
【相关文献】
1.基于支持向量机的嵌入拒识代价的手写字符识别研究 [J], 黄口;刘思颂;孔瑞
2.动态误分类代价下代价敏感属性选择分治算法 [J], 黄伟婷;赵红
3.嵌入拒识代价的投票式极限学习机 [J], 徐鑫
4.代价敏感属性中动态误分类代价下Bayes判别函数探究 [J], 牛军霞
5.代价敏感属性中动态误分类代价下的Fisher判别函数 [J], 牛军霞
因版权原因,仅展示原文概要,查看原文内容请购买。
高维时空数据的建模与统计推断, 英文
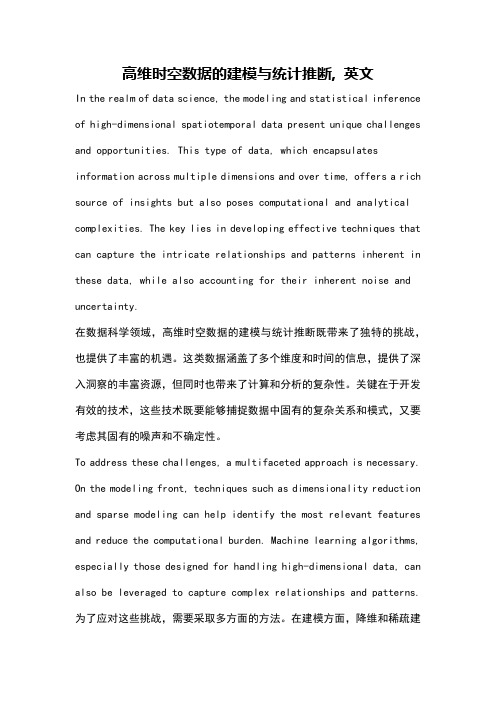
高维时空数据的建模与统计推断, 英文In the realm of data science, the modeling and statistical inference of high-dimensional spatiotemporal data present unique challenges and opportunities. This type of data, which encapsulates information across multiple dimensions and over time, offers a rich source of insights but also poses computational and analytical complexities. The key lies in developing effective techniques that can capture the intricate relationships and patterns inherent in these data, while also accounting for their inherent noise and uncertainty.在数据科学领域,高维时空数据的建模与统计推断既带来了独特的挑战,也提供了丰富的机遇。
这类数据涵盖了多个维度和时间的信息,提供了深入洞察的丰富资源,但同时也带来了计算和分析的复杂性。
关键在于开发有效的技术,这些技术既要能够捕捉数据中固有的复杂关系和模式,又要考虑其固有的噪声和不确定性。
To address these challenges, a multifaceted approach is necessary. On the modeling front, techniques such as dimensionality reduction and sparse modeling can help identify the most relevant features and reduce the computational burden. Machine learning algorithms, especially those designed for handling high-dimensional data, can also be leveraged to capture complex relationships and patterns.为了应对这些挑战,需要采取多方面的方法。
基于混合核函数支持向量机和遗传算法的人脸识别

基于混合核函数支持向量机和遗传算法的人脸识别任彧;梅盛鑫【摘要】提出了一种基于混合核函数支持向量机和遗传算法的识别方法,用于人脸识别.该方法结合了支持向量机的学习性能和遗传算法的寻优性能,与传统的方法相比,具有速度快、误差少、效率高的特点,在实验中能够较精确地对人脸进行识别.%This paper proposes a recognition method, which is based on mixed kernel function support vector machine and genetic algorithm, for human face recognition. The method combines the learning performance of support vector machine with the searching performance of genetic algorithm. Compared with traditional methods, this face recognition method has the features of faster, less error and more efficient, in experiment the meth is more accurate in recognising human faces.【期刊名称】《计算机应用与软件》【年(卷),期】2011(028)004【总页数】4页(P260-263)【关键词】混合核函数;支持向量机;遗传算法;人脸识别【作者】任彧;梅盛鑫【作者单位】杭州电子科技大学软件与智能技术研究所,浙江,杭州,310018;杭州电子科技大学软件与智能技术研究所,浙江,杭州,310018【正文语种】中文人脸识别作为模式识别的一个重要分支,在智能监控、虚拟现实、医学检测、人机交互等方面有着广泛的应用。
《2024年面向深度学习的多模态融合技术研究综述》范文

《面向深度学习的多模态融合技术研究综述》篇一一、引言随着信息技术的飞速发展,多模态数据在各个领域的应用越来越广泛。
多模态融合技术作为处理多模态数据的重要手段,其研究价值和应用前景日益凸显。
深度学习技术的崛起为多模态融合技术提供了新的思路和方法。
本文旨在综述面向深度学习的多模态融合技术的研究现状、主要方法及挑战,以期为相关研究提供参考。
二、多模态数据与多模态融合技术多模态数据是指来自不同感官或不同数据源的信息,如文本、图像、音频、视频等。
多模态融合技术是将这些不同模态的数据进行有效融合,以提取更多有用信息,提高数据处理和问题解决的能力。
多模态融合技术可以应用于许多领域,如智能问答、虚拟助手、多媒体分析等。
三、面向深度学习的多模态融合技术研究现状面向深度学习的多模态融合技术主要通过深度学习模型对不同模态的数据进行特征提取和融合。
目前,该领域的研究主要集中在以下几个方面:1. 数据预处理:在多模态数据融合前,需要对数据进行预处理,包括数据清洗、标准化、特征提取等。
预处理的效果对后续的融合过程具有重要影响。
2. 特征提取与表示:利用深度学习模型对不同模态的数据进行特征提取和表示学习。
这一过程需要设计合适的模型结构,以适应不同模态数据的特性。
3. 融合策略:多模态数据的融合策略是研究的重点。
目前,常见的融合策略包括早期融合、中期融合和晚期融合。
早期融合在数据输入阶段进行融合,中期融合在特征提取过程中进行融合,晚期融合则是在决策层进行融合。
4. 模型训练与优化:针对多模态融合任务,需要设计合适的模型训练方法和优化策略。
这包括损失函数的选择、训练过程的调整等。
四、主要方法及挑战1. 主要方法:目前,面向深度学习的多模态融合技术主要采用基于深度学习的模型进行特征提取和融合。
其中,卷积神经网络(CNN)和循环神经网络(RNN)是应用最广泛的模型。
此外,还有一些新兴的模型和方法,如Transformer、图神经网络等。
研判分析工作流程
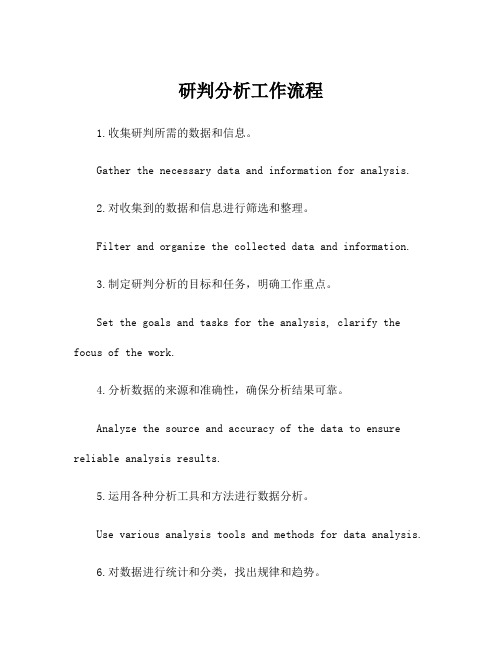
研判分析工作流程1.收集研判所需的数据和信息。
Gather the necessary data and information for analysis.2.对收集到的数据和信息进行筛选和整理。
Filter and organize the collected data and information.3.制定研判分析的目标和任务,明确工作重点。
Set the goals and tasks for the analysis, clarify the focus of the work.4.分析数据的来源和准确性,确保分析结果可靠。
Analyze the source and accuracy of the data to ensure reliable analysis results.5.运用各种分析工具和方法进行数据分析。
Use various analysis tools and methods for data analysis.6.对数据进行统计和分类,找出规律和趋势。
Statistics and classification of data to find patterns and trends.7.研究相关领域的发展动态和新趋势,辅助分析工作。
Study the development trends and new dynamics in the relevant fields to assist in the analysis.8.利用专业知识和经验进行研判分析。
Utilize professional knowledge and experience for analysis.9.结合实际情况,制定合理的分析方向和方法。
Combine the actual situation to develop a reasonable analysis direction and method.10.对不同来源的数据进行交叉对比,寻找数据之间的关联。
语义分析的一些方法

语义分析的一些方法语义分析的一些方法(上篇)•5040语义分析,本文指运用各种机器学习方法,挖掘与学习文本、图片等的深层次概念。
wikipedia上的解释:In machine learning, semantic analysis of a corpus is the task of building structures that approximate concepts from a large set of documents(or images)。
工作这几年,陆陆续续实践过一些项目,有搜索广告,社交广告,微博广告,品牌广告,内容广告等。
要使我们广告平台效益最大化,首先需要理解用户,Context(将展示广告的上下文)和广告,才能将最合适的广告展示给用户。
而这其中,就离不开对用户,对上下文,对广告的语义分析,由此催生了一些子项目,例如文本语义分析,图片语义理解,语义索引,短串语义关联,用户广告语义匹配等。
接下来我将写一写我所认识的语义分析的一些方法,虽说我们在做的时候,效果导向居多,方法理论理解也许并不深入,不过权当个人知识点总结,有任何不当之处请指正,谢谢。
本文主要由以下四部分组成:文本基本处理,文本语义分析,图片语义分析,语义分析小结。
先讲述文本处理的基本方法,这构成了语义分析的基础。
接着分文本和图片两节讲述各自语义分析的一些方法,值得注意的是,虽说分为两节,但文本和图片在语义分析方法上有很多共通与关联。
最后我们简单介绍下语义分析在广点通“用户广告匹配”上的应用,并展望一下未来的语义分析方法。
1 文本基本处理在讲文本语义分析之前,我们先说下文本基本处理,因为它构成了语义分析的基础。
而文本处理有很多方面,考虑到本文主题,这里只介绍中文分词以及Term Weighting。
1.1 中文分词拿到一段文本后,通常情况下,首先要做分词。
分词的方法一般有如下几种:•基于字符串匹配的分词方法。
此方法按照不同的扫描方式,逐个查找词库进行分词。
嵌入时延神经网络的高斯混合模型说话人辨认

(col no t n cne n E gnei ,otesui rt, af g20 9 , h a Shoo fr i Si cad nie n S u a nv sy N nn 10 6C i ) fI ma o e rg h t ei i n
d g sst m eifr ain o efaur es a d tr u h t eta so m ai no h e tr e tri m a steb - iet t o m t ft e t est, n h o g h n f r to ftefau ev co t ke y hei n o h r h p te i o d p n e c a xmu l l o dn e s o e e s n b e GM M n oh ss fi e e d n et t n h ma i m  ̄ei o e d r a o a l. h m r a dTDNN r rie s oeb a et n da wh l y a a
摘要 :提 出了一种 在高斯混合模型中嵌入时延神经 网络的方法。它集成 了作为判别性方法 的时延神经网络和作 为生
成性 方 法 的 高 斯混 合 模 型 各 自的优 点 。 时延 神 经 网络 挖 掘 了特 征 向量 集 的 时 间信 息 , 并且 通 过 时 延 网 络 的变 换 使 需 要 假 设 变 量独 立 的 最 大 似 然概 率f ) 法 更 为 合 理 。 以最 大 似 然 概 率 为准 则 ,把 它 们 作为 一 个 整 体 来 进 行 训练 。训 ML方
Ex e i n w a ep o os d s se i r v s c u a yr t g i s a e i e GM M t l S p rme t s s ho t t h r p e y t m h t mp o e c r c a ea an t s l a b n a l NR t a i m a wi am x mu h
- 1、下载文档前请自行甄别文档内容的完整性,平台不提供额外的编辑、内容补充、找答案等附加服务。
- 2、"仅部分预览"的文档,不可在线预览部分如存在完整性等问题,可反馈申请退款(可完整预览的文档不适用该条件!)。
- 3、如文档侵犯您的权益,请联系客服反馈,我们会尽快为您处理(人工客服工作时间:9:00-18:30)。
Incentive-Based Modeling and Inference of Attacker Intent,Objectives,and StrategiesPENG LIU and WANYU ZANGPennsylvania State UniversityandMENG YUMonmouth UniversityAlthough the ability to model and infer attacker intent,objectives,and strategies(AIOS)may dramatically advance the literature of risk assessment,harm prediction,and predictive or proactive cyber defense,existing AIOS inference techniques are ad hoc and system or application specific. In this paper,we present a general incentive-based method to model AIOS and a game-theoretic approach to inferring AIOS.On one hand,we found that the concept of incentives can unify a large variety of attacker intents;the concept of utilities can integrate incentives and costs in such a way that attacker objectives can be practically modeled.On the other hand,we developed a game-theoretic AIOS formalization which can capture the inherent interdependency between AIOS and defender objectives and strategies in such a way that AIOS can be automatically inferred.Finally, we use a specific case study to show how attack strategies can be inferred in real-world attack–defense scenarios.Categories and Subject Descriptors:C.2.0[Computer-Communication Networks]:Security and ProtectionGeneral Terms:Security,TheoryAdditional Key Words and Phrases:Attacker intent and strategy modeling,attack strategy infer-ence,game theory1.INTRODUCTIONThe ability to model and infer attacker intent,objectives,and strategies(AIOS) may dramatically advance the state of the art of computer security for several reasons.First,for many“very difficult to prevent”attacks such as DDoS,given the specification of a system protected by a set of specific security mechanisms, This work was supported by DARPA and AFRL,AFMC,USAF,under award number F20602-02-1-0216,and by Department of Energy Early Career PI Award.Authors’addresses:P.Liu and W.Zang,School of Information Sciences and Technology, Pennsylvania State University,University Park,PA16802;email:pliu@;M.Yu,De-partment of Computer Science,Monmouth University,West Long Branch,NJ07764. Permission to make digital or hard copies of part or all of this work for personal or classroom use is granted without fee provided that copies are not made or distributed for profit or direct commercial advantage and that copies show this notice on thefirst page or initial screen of a display along with the full citation.Copyrights for components of this work owned by others than ACM must be honored.Abstracting with credit is permitted.To copy otherwise,to republish,to post on servers, to redistribute to lists,or to use any component of this work in other works requires prior specific permission and/or a fee.Permissions may be requested from Publications Dept.,ACM,Inc.,1515 Broadway,New York,NY10036USA,fax:+1(212)869-0481,or permissions@.C 2005ACM1094-9224/05/0200-0078$5.00ACM Transactions on Information and System Security,Vol.8,No.1,February2005,Pages78–118.Incentive-Based Modeling and Inference of AIOS•79 this ability could tell us which kind of strategies are more likely to be taken by the attacker than the others,even before such an attack happens.Such AIOS inferences may lead to more precise risk assessment and harm prediction.Second,AIOS modeling and inference could be more beneficial during run time.A big security challenge in countering a multiphase,well-planned,care-fully hidden attack from either malicious insiders or outside attackers is“how to make correct proactive(especially predictive)real-time defense decisions during an earlier stage of the attack in such a way that much less harm will be caused without consuming a lot of resources?”Although many proactive de-fense techniques are developed such as sandboxing[Malkhi and Reiter2000] and isolation[Liu et al.2000],making the right proactive defense decisions in real time is very difficult primarily due to the fact that intrusion detection during the early stage of an attack can lead to many false alarms,which could make these proactive defense actions very expensive in terms of both resources and denial of service.Although alert correlation techniques[Cuppens and Miege2002;Ning et al. 2002]may reduce the number of false alarms by correlating a set of alerts into an attack scenario(i.e.,steps involved in an attack)and may even tell which kind of attack actions may follow a given action[Debar and Wespi2001], they are limited in supporting proactive intrusion response in two aspects.(1) When many types of(subsequences of)legitimate actions may follow a given suspicious action,alert correlation can do nothing except for waiting until a more complete attack scenario emerges.However,intrusion response at this moment could be“too late.”(2)When many types of attack actions may follow a given(preparation)action,alert correlation cannot tell which actions are more likely to be taken by the attacker next.As a result,since taking proactive defense actions for each of the attack actions can be too expensive,the response may have to wait until it is clear what attack actions will happen next—perhaps during a later stage of the attack.However,late intrusion response usually means more harm.By contrast,with the ability to model and infer AIOS,given any suspicious action,we can predict the harm that could be caused;then we can make better and affordable proactive intrusion response decisions based on the corresponding risk,the corresponding cost(e.g.,due to the possibility of false alarms),and the attack action inferences.Moreover,the intrusion response time is substantially shortened.However,with a focus on attack characteristics[Landwehr et al.1994]and attack effects[Browne et al.2001;Zou et al.2002],existing AIOS inference tech-niques are ad hoc and system or application specific[Gordon and Loeb2001; Syverson1997].To systematically model and infer AIOS,we need to distin-guish AIOS from both attack actions and attack effects.Since the same attack action can be issued by two attackers with very different intents and objectives, AIOS cannot be directly inferred from the characteristics of attacks.Although the attacker achieves his or her intents and objectives through attacks and their effects,the mapping from attack actions and/or effects to attacker intents and/or objectives is usually not one-to-one but one-to-many,and more inter-estingly,the(average)cardinality of this mapping can be much larger than the mapping from attacker intents and/or objectives to attack actions and/or ACM Transactions on Information and System Security,Vol.8,No.1,February2005.80•P.Liu et al.effects.This asymmetry nature indicates that in many cases using AIOS mod-els to predict attack actions can be more precise than using the set of actions already taken by the attacker based on either their effects or the causal rela-tionship between them and some other attack actions.1As a result,although a variety of attack taxonomies and attribute databases have been developed, people’s ability to model and infer AIOS,to predict attacks,and to do proactive intrusion response is still very limited.Nevertheless,a good understanding of attacks is the foundation of practical AIOS modeling and inference.In this paper,we present a systematic incentive-based method to model AIOS and a game-theoretic approach to inferring AIOS.On one hand,we found that the concept of incentives can unify a large variety of attacker intents;the con-cept of utilities can integrate incentives and costs in such a way that attacker objectives can be practically modeled.On the other hand,we developed a game-theoretic AIOS formalization which can capture the inherent interdependency between AIOS and defender objectives and strategies in such a way that AIOS can be automatically inferred.Finally,we use a specific case study to show how attack strategies can be inferred in real-world attack–defense scenarios.The proposed framework,in some sense,is an economics-based framework since it is based on economic incentives,utilities,and payoffs.The rest of the paper is organized as follows.In Section2,we discuss the related work.Section3presents a conceptual,incentive-based framework for AIOS modeling.In Section4,we present a game-theoretic formalization of this framework.Section5addresses show to infer AIOS.In Section6,we use a specific case study to show how attack strategies can be inferred in real-world attack–defense scenarios.In Section7,we mention several future research issues.2.RELATED WORKThe use of game theory in modeling attackers and defenders has been addressed in several other research.In Syverson[1997],Syverson talks about“good”nodes fighting“evil”nodes in a network and suggests using stochastic games for rea-soning and analysis.In Lye and Wing[2002],Lye and Wing precisely formalize this idea using a general-sum stochastic game model and give a concrete ex-ample in detail where the attacker is attacking a simple enterprise network that provides some Internet services such as web and FTP.A set of specific states regarding this example are identified,state-transition probabilities are assumed,and the Nash equilibrium or best-response strategies for the players are computed.In Browne[2000],Browne describes how static games can be used to an-alyze attacks involving complicated and heterogeneous military networks.In his example,a defense team has to defend a network of three hosts against an attacking team’s worms.The defense team can choose either to run a worm 1To illustrate,consider a large space of strategies the attacker may take according to his or her intent and objectives where each strategy is simply a sequence of actions.An attack action may belong to many strategies,and the consequences of the action could satisfy the preconditions of many other actions,but each strategy usually contains only a small number of actions.ACM Transactions on Information and System Security,Vol.8,No.1,February2005.Incentive-Based Modeling and Inference of AIOS•81 detector or not.Depending on the combined attack and defense actions,each outcome has different costs.In Burke[1999],Burke studies the use of repeated games with incomplete information to model attackers and defenders in in-formation warfare.In Hespanha and Bohacek[2001],Hespanha and Bohacek discuss zero-sum routing games where an adversary(or attacker)tries to in-tersect data packets in a computer network.The designer of the network has to find routing policies that avoid links that are under the attacker’s surveillance. In Xu and Lee[2003],Xu and Lee use game-theoretical framework to analyze the performance of their proposed DDoS defense system and to guide its design and performance tuning accordingly.Our work is different from the above game theoretic attacker modeling works in several aspects.First,these works focus on specific attack–defense scenarios, while our work focuses on general AIOS modeling.Second,these works focus on specific types of game models,for example,static games,repeated games, or stochastic games;while our work focuses on the fundamental characteris-tics of AIOS,and game models are only one possible formalization of our AIOS framework.In addition,our AIOS framework shows the inherent relationship between AIOS and the different types of game models,and identifies the condi-tions under which a specific type of game models will be feasible and desirable. Third,our work systematically identifies the properties of a good AIOS for-malization.These properties not only can be used to evaluate the merits and limitations of game-theoretic AIOS models,but also can motivate new AIOS models that can improve the above game theory models or even go beyond standard game-theoretic models.In Gordon and Loeb[2001],information security is used as a response to game theoretic competitor analysis systems(CAS)for the purpose of protecting a firm’s valuable business data from its competitors.Although understanding and predicting the behavior of competitors are key aspects of competitor analysis, the behaviors CAS want to predict are not cyber attacks.Moreover,security is what our game theoretic system wants to model while security is used in Gordon and Loeb[2001]to protect a game-theoretic system.The computational complexity of game-theoretic analysis is investigated in several research.For example,Conitzer and Sandholm[2002]show that both determining whether a pure strategy Bayes–Nash equilibrium exists and de-termining whether a pure strategy Nash equilibrium exists in a stochastic (Markov)game are NP-hard.Moreover,Koller and Milch[2001]show that some specific knowledge representations,in certain settings,can dramatically speed up equilibriumfinding.The marriage of economics and information security has attracted a lot of in-terests recently(a lot of related work can be found at the economics and security resource page maintained by Ross Anderson at /∼rja14 /econsec.html).However,these work focuses on the economics perspective of security(e.g.,security market,security insurance),while our approach is to apply economics concepts to model and infer AIOS.In recent years,it is found that economic mechanism design theory[Clarke 1971;Groves1973;Vickrey1961]can be very valuable in solving a variety of Internet computing problems such as routing,packet scheduling,and web ACM Transactions on Information and System Security,Vol.8,No.1,February2005.82•P.Liu et al.work topology.caching[Feigenbaum et al.2002;Nisan and Ronan2001;Wellman and Walsh2001].Although when market-based mechanisms are used to defend against at-tackers[Wang and Reiter2003],the AIOS are incentive based,which is consis-tent with our framework,market-based computing does not imply an in-depthAIOS model.Finally,it should be noticed that AIOS modeling and inference are very differ-ent from intrusion detection[Lunt1993;McHugh2001;Mukherjee et al.1994].Intrusion detection is based on the characteristics of attacks,while AIOS mod-eling is based on the characteristics of attackers.Intrusion detection focuses onthe attacks that have already happened,while AIOS inference focuses on theattacks that may happen in the future.3.AN INCENTIVE-BASED FRAMEWORK FOR AIOS MODELINGIn this section,we present an incentive-based conceptual model of attackerintent,objectives,and strategies.Our model is quite abstract.To make ourpresentation more tangible,we willfirst present the following example,whichwill be used throughout the paper to illustrate our concepts.Example1.In recent years,Internet distributed denial-of-service(DDoS)attacks have increased in frequency,severity,and sophistication and becomea major security threat.When a DDoS attack is launched,a large number ofhosts(called zombies)“controlled”by the attackerflood a high volume of pack-ets toward the target(called the victim)to downgrade its service performancesignificantly or make it unable to deliver any service.In this example,we would model the intent and objectives and infer thestrategies of the attackers that enforce brute-force DDoS attacks.(Althoughsome DDoS attacks with clear signatures,such as SYNflooding,can be effec-tively countered,most DDoS attacks without clear signatures,such as brute-force DDoS attacks,are very difficult to defend against since it is not clear whichpackets are DDoS packets and which are not.)An example scenario is shownin Figure1where many zombies(i.e.,a subset of source hosts{S0,...,S64})are flooding a couple of web sites(i.e.,the victims)using normal HTTP requests.Here,Rx.y denotes a router;the bandwidth of each type of links is marked;andthe web sites may stay on different subnets.ACM Transactions on Information and System Security,Vol.8,No.1,February2005.Incentive-Based Modeling and Inference of AIOS•83 Although our modeling and inference framework can handle almost every DDoS defense mechanism,to make this example more tangible,we select pushback[Ioannidis and Bellovin2002],a popular technique,as the security mechanism.Pushback uses aggregates,that is,a collection of packets from one or moreflows that have some properties in common,to identify and rate limit the packets that are most likely to cause congestion or DoS.Pushback is a coordinated defense mechanism that typically involves multiple routers.To il-lustrate,consider Figure1again,when router R1.0detects a congestion caused by a set of aggregates,R1.0will not only rate-limit these aggregates,but also request adjacent upstream routers(e.g.,R2.1)to rate-limit the corresponding aggregates via some pushback messages.The effectiveness of pushback can be largely captured by four bandwidth parameters associated with the incoming link to the victims(i.e.,the link that connects R1.0and R0.0):(a)B N,the total bandwidth of this link;(b)B ao,the (amount of)bandwidth occupied by the DoS packets;(c)B lo,the bandwidth occupied by the legitimate packets;(d)B lw,the bandwidth that the legitimate users would occupy if there are no attacks.For example,pushback is effective if after being enforced B ao can become smaller and B lo can become larger.We build our AIOS models on top of the relationships between the attacker and a computer system(i.e.,the defender).In our model,the computer sys-tem can be any kind(e.g.,a network system,a distributed system,a database system).We call it the system for short.For example,in Example1the sys-tem consists of every router on a path from a zombie to a victim.The attacker issues attacks to the system.Each attack is a sequence of attack actions associ-ated with the system.For example,an action can be the sending of a message, the submission of a transaction,the execution of a piece of code,and so on.An attack will cause some effects on the system,that is,transforming the system from one state to another state.For example,in Example1the main attack effects are that many legitimate packets could not reach the victims.Part of the system is a set of specific security mechanisms.A mechanism can be a piece of software or hardware(e.g.,afirewall,an access controller,an IDS).A mechanism usually involves a sequence of defense actions associated with the system when being activated.For example,in Example1a router sending out a pushback message is a defense action,and this action can trigger the receiving router(s)to take further defense actions.A security mechanism is activated when an event arrives which causes a set of specific conditions to be satisfied.Many of these conditions are associated with the effects of an attack action in reactive defense,or the prediction of an incoming attack action in proactive defense.For example,in Example1a packet arriving at a router is an event.When there is no congestion at the router,this event will not activate any security mechanism.However,when this event leads to“the detection of a congestion”(i.e.,the condition),pushback will be activated.And it is clear that whether this condition can be satisfied is dependent upon the accumulated effects of the previous DoS packets arriving at the router.Finally,a defense posture of the system is defined by the set of security mechanisms and the ways they are activated.For example,in Example1,pushback may be configured ACM Transactions on Information and System Security,Vol.8,No.1,February2005.84•P.Liu et al.to stay at various defense postures based on such parameters as congestion thresholds and target drop rate,which we will explain in Section3.3shortly.The attacker-system relation has several unique characteristics(or proper-ties)that are important in illustrating the principles of our attack strategy inference framework.These properties are as follows.—Intentional Attack Property.Attacks are typically not random.They are planned by the attacker based on some intent and objectives.—Strategy-Interdependency Property.Whether an attack can succeed is depen-dent on how the system is protected.Whether a security mechanism is effec-tive is dependent on how the system is attacked.In other words,the capacity of either an attack or a defense posture should be measured in a relative way.We will define the notion of strategy shortly.And we will use concrete attack and defense strategies derived from Example1to illustrate this property shortly in Section3.3.—Uncertainty Property.The attacker usually has incomplete information or knowledge about the system,and vice versa.For example,in Example1the attacker usually has uncertainty about how Pushback is configured when he or she enforces a DDoS attack.3.1Incentive-Based Attacker Intent ModelingDifferent attackers usually have different intents even when they issue the same attack.For example,some attackers attack the system to show off their hacking capacity,some hackers attack the system to remind the administrator of a securityflaw,cyber terrorists attack our cyberspace for creating damage, business competitors may attack each other’s information systems to increase their market shares,just to name a few.It is clear that investigating the char-acteristics of each kind of intents involves a lot of effort and complexity,and such complexity actually prevents us from building a general,robust connec-tion between attacker intents and attack actions.This connection is necessary to do almost every kind of attacker behavior inference.We focus on building general yet simple intent models.In particular,we believe that the concept of economic“incentives”can be used to model attacker intent in a general way.In our model,the attacker’s intent is simply to maximize his or her incentives.In other words,the attacker is motivated by the possibility of gaining some incentives.Most,if not all,kinds of intents can be modeled as incentives such as the amount of profit earned,the amount of terror caused, and the amount of satisfaction because of a nice show-off.For an example,in Example1the incentives for the attacker can be the amount of DoS suffered by the legitimate users.For another example,the incentives for an attacker that enforces a worm attack can be the amount of network resources consumed by the worm’s scanning packets plus the amount of DoS caused on certain type of services.We may use economics theory to classify incentives into such categories as money,emotional reward,and fame.To infer attacker intents,we need to be able to compare one incentive with another.Incentives can be compared with each other either qualitatively or ACM Transactions on Information and System Security,Vol.8,No.1,February2005.Incentive-Based Modeling and Inference of AIOS•85 quantitatively.Incentives can be quantified in several ways.For example,prof-its can be quantified by such monetary units as dollars.For another exam-ple,in Example1,the attacker’s incentives can be quantified by two metrics: (a)B ao/B N,which indicates the absolute impact of the DDoS attack;and(b)B lo/B lw,which indicates the relative availability impact of the attack.Accord-ingly,the attacker’s intent is to maximize B ao/B N but minimize B lo/B lw.One critical issue in measuring and comparing incentives is that under different value systems,different comparison results may be obtained.For example,dif-ferent types of people value such incentives as time,fame,and differently.As a result,very misleading attacker strategy inferences could be produced if we use our value system to evaluate the attacker’s incentives.After an attack is enforced,the incentives(e.g.,money,fame)earned by the attacker are dependent on the effects of the attack,which are typically captured by the degradation of a specific set of security measurements that the system cares about.Each such measurement is associated with a specific security met-ric.Some widely used categories of security metrics include but not limited to confidentiality,integrity,availability(against denial-of-service),nonrepudia-tion,and authentication.For example,in Example1the major security metrics of the system are(a)B lo,which indicates the absolute availability provided by the system;and(b)B lo/B lw,which indicates the relative availability provided by the system.In our model,we call the set of security metrics that a system wants to protect the metric vector of the system.(Note that different systems may have different metric vectors.)For example,the metric vector for the system in Example1can be simply defined as B lo,B lo/B lw .At time t,the measurements associated with the system’s metric vector are called the security vector of the system at time t,denoted by V s t.As a result,assume an attack starts at time t1 and ends at t2,then the incentives earned by the attacker(via the attack)maybe measured by degradation(V s t1,V s t2),which basically computes the distance be-tween the two security vectors.For example,in Example1assume the securityvector is V s t1= 1000Mbps,100% before the attack and V s t2= 50Mbps,5%after the attack,then degradation(V s t1,V s t2)= −950Mbps,−95% .The above discussion indicates the following property of AIOS inference:—Attack Effect Property.Effects of attacks usually yield more insights about at-tacker intent and objectives than attack actions.For example,in Example1,a DoS packet indicates almost nothing about the attacker’s intent which canonly be seen after some DoS effects are caused.3.2Incentive-Based Attacker Objective ModelingIn real world,many attackers face a set of constraints when issuing an attack, for example,an attacker may have limited resources;a malicious insider may worry about the risk of being arrested and put into jail.However,our intent model assumes no constraints.To model attacker motivations in a more realistic way,we incorporate constraints in our attack objective model.In particular,we classify constraints into two categories:cost constraints and noncost constraints.(a)Cost constraints are constraints on things that the attacker can“buy”or “trade”such as hardware,software,Internet connection,and time.Such things ACM Transactions on Information and System Security,Vol.8,No.1,February2005.86•P.Liu et al.are typically used to measure the cost of an attack.In addition,risk is typically a cost constraint.(b)Noncost constraints are constraints on things that the attacker cannot buy such as religion-based constraints and top secret attacking tools that the attacker may never be able to“buy.”The cost of an attack is not only dependent on the resources needed to en-force the attack,but also dependent on the risk for the attacker to be traced back,arrested,and punished.Based on the relationship between incentives and costs,we classify attackers into two categories:(a)rational attackers have concerns about the costs(and risk)associated with their attacks.That is,when the same incentive can be obtained by two attacks with different costs,ratio-nal attackers will pick the one with a lower cost.(b)Irrational attackers have no concerns about the costs associated with their attacks.They only want to maximize the incentives.Given a set of(cost)constraints,inferring the attack actions of an irrational attacker is not so difficult a task since we need only tofind out“what are the most rewarding attack actions in the eyes of the attacker without violating the constraints?”By contrast,we found that inferring the attack actions of a rational attacker is more challenging.In this paper,we will focus on how to model and infer the IOS of rational attackers.In our model,an attacker’s objective is to maximize his or her utilities through an attack without violating the set of cost and noncost constraints associated with the attacker.The utilities earned by an attacker indicate a distance be-tween the incentives earned by the attacker and the cost of the attack.The dis-tance can be defined in several ways,for example,utilities=incentives−cost, utilities=incentives.Note that the cost of an attack can be measured by a set of cost values which captures both attacking resources and risk.To illustrate,let us revisit Example1.The attacker’s total incentives may be measured byαB ao/B N+(1−α)(1−B lo/B lw),whereαdetermines how the attacker weighs the two aspects of the impact of the DDoS attack.The attack’s costs in this example are not much,though the attacker needs a computer and Internet access to“prepare”the zombies and the needed controls.The cost will become larger when the risk of being traced back is included.Let us assume the cost is a constant numberη.Then the attacker’s utilities can be measured by αB ao/B N+(1−α)(1−B lo/B lw)−η,and the attacker’s objective can be quantified as MaxαB ao/B N+(1−α)(1−B lo/B lw).3.3Incentive-Based Attacker Strategy ModelingStrategies are taken to achieve objectives.The strategy-interdependency prop-erty indicates that part of a good attacker strategy model should be the defense strategy model because otherwise we will build our AIOS models on top of the assumption that the system never changes its defense posture,which is too restrictive.See that whenever the system’s defense posture is changed,the defense strategy is changed.In our model,attack strategies are defined based on the“battles”between the attacker and the system.Each attack triggers a battle which usually involves multiple phases.(For example,many worm-based attacks involve such phases ACM Transactions on Information and System Security,Vol.8,No.1,February2005.。