伍德里奇计量经济学绪论共54页
《计量经济学导论》考研伍德里奇考研复习笔记二
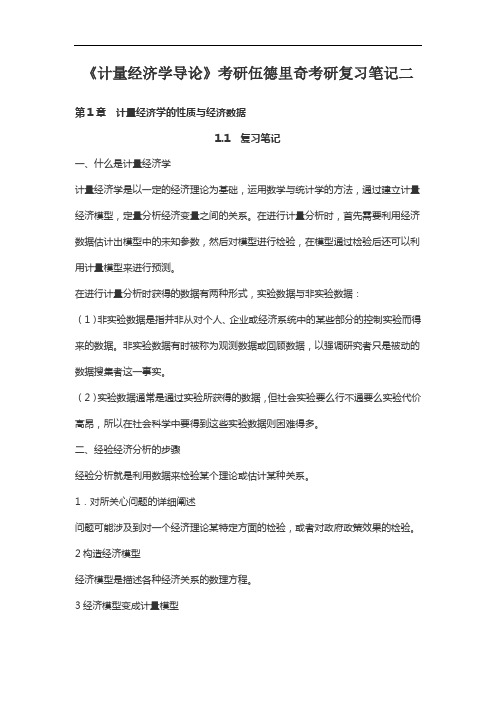
《计量经济学导论》考研伍德里奇考研复习笔记二第1章计量经济学的性质与经济数据1.1 复习笔记一、什么是计量经济学计量经济学是以一定的经济理论为基础,运用数学与统计学的方法,通过建立计量经济模型,定量分析经济变量之间的关系。
在进行计量分析时,首先需要利用经济数据估计出模型中的未知参数,然后对模型进行检验,在模型通过检验后还可以利用计量模型来进行预测。
在进行计量分析时获得的数据有两种形式,实验数据与非实验数据:(1)非实验数据是指并非从对个人、企业或经济系统中的某些部分的控制实验而得来的数据。
非实验数据有时被称为观测数据或回顾数据,以强调研究者只是被动的数据搜集者这一事实。
(2)实验数据通常是通过实验所获得的数据,但社会实验要么行不通要么实验代价高昂,所以在社会科学中要得到这些实验数据则困难得多。
二、经验经济分析的步骤经验分析就是利用数据来检验某个理论或估计某种关系。
1.对所关心问题的详细阐述问题可能涉及到对一个经济理论某特定方面的检验,或者对政府政策效果的检验。
2构造经济模型经济模型是描述各种经济关系的数理方程。
3经济模型变成计量模型先了解一下计量模型和经济模型有何关系。
与经济分析不同,在进行计量经济分析之前,必须明确函数的形式,并且计量经济模型通常都带有不确定的误差项。
通过设定一个特定的计量经济模型,我们就知道经济变量之间具体的数学关系,这样就解决了经济模型中内在的不确定性。
在多数情况下,计量经济分析是从对一个计量经济模型的设定开始的,而没有考虑模型构造的细节。
一旦设定了一个计量模型,所关心的各种假设便可用未知参数来表述。
4搜集相关变量的数据5用计量方法来估计计量模型中的参数,并规范地检验所关心的假设在某些情况下,计量模型还用于对理论的检验或对政策影响的研究。
三、经济数据的结构1横截面数据(1)横截面数据集,是指在给定时点对个人、家庭、企业、城市、州、国家或一系列其他单位采集的样本所构成的数据集。
伍德里奇计量经济学绪论共54页文档
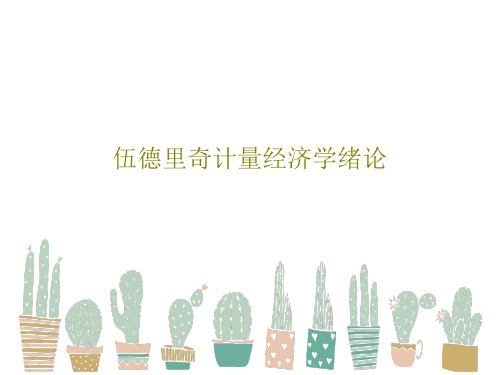
46、法律有权打破平静。——马·格林 47、在一千磅法律里,没有一盎司仁 爱。— —英国
48、法律一多,公正就少。——托·富 勒 49、犯罪总是以惩罚相补偿;只有处 罚才能 使犯罪 得到偿 还。— —达雷 尔
50、弱者就是财富 ❖ 丰富你的人生
71、既然我已经踏上这条道路,那么,任何东西都不应妨碍我沿着这条路走下去。——康德 72、家庭成为快乐的种子在外也不致成为障碍物但在旅行之际却是夜间的伴侣。——西塞罗 73、坚持意志伟大的事业需要始终不渝的精神。——伏尔泰 74、路漫漫其修道远,吾将上下而求索。——屈原 75、内外相应,言行相称。——韩非
伍德里奇计量经济学导论
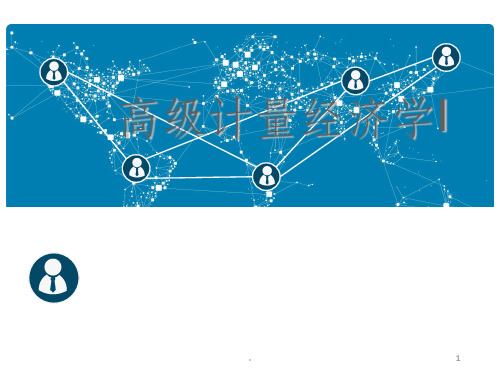
(3)因此,给定收入X的值Xi,可得消费支出Y的条件均值(conditional mean)或条件期望(conditional expectation): E(Y|X=Xi)
(4)该例中: E(Y | X=80)=65
.
描出散点图发现:随着收入的增加,消费“平均地说”也在增加,且Y的 条件均值均落在一根正斜率的直线上。这条直线称为总体回归线。
. E(y|x) = 0 + 1x
x1=5
x2 =10
.
34
对于所研究的经济问题,通常总体回归直线 E(Yi|Xi) = 0 + 1Xi 是观测不到
的。可以通过收集样本来对总体(真实的)回归直线做出估计。
样本回归模型: Yˆi ˆ0ˆ1Xi
或: Yi ˆ0ˆ1Xiei
② y = 0 + 1 x + u
u 为误差项或扰动项,它代表了除了x之外可以影响y的因素。
l 线性回归的含义: y 和x 之间并不一定存在线性关系,但是,只 要通过转换可以使y的转换形式和x的转换形式存在相对于参数的 线性关系,该模型即称为线性模型。
.
19 19
Ø 总体回归函数的随机设定
l 对于某一个家庭,如何描述可支配收入和消费支出的关系?
l 等式右边的变量被称为解释变量(Explanaiory Variable)或自 变量(Independent Variable)、右边变量、回归元,协变量,或控制变量。
l 等式y = b0 + b1x + u只有一个非常数回归元。我们称之为简单回归模型, 两
变量回归模型或双变量回归模型.
.
Ø 回归分析的目的
a. 函数形式:可以是线性或非线性的。
《计量经济学导论》考研伍德里奇版考研复习笔记
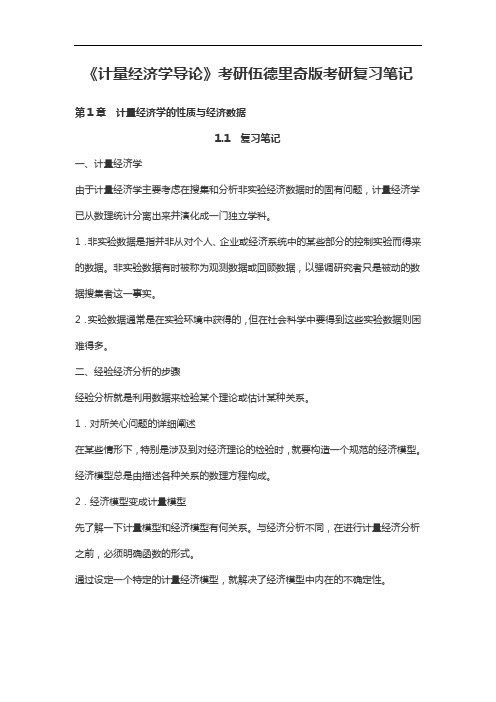
《计量经济学导论》考研伍德里奇版考研复习笔记第1章计量经济学的性质与经济数据1.1 复习笔记一、计量经济学由于计量经济学主要考虑在搜集和分析非实验经济数据时的固有问题,计量经济学已从数理统计分离出来并演化成一门独立学科。
1.非实验数据是指并非从对个人、企业或经济系统中的某些部分的控制实验而得来的数据。
非实验数据有时被称为观测数据或回顾数据,以强调研究者只是被动的数据搜集者这一事实。
2.实验数据通常是在实验环境中获得的,但在社会科学中要得到这些实验数据则困难得多。
二、经验经济分析的步骤经验分析就是利用数据来检验某个理论或估计某种关系。
1.对所关心问题的详细阐述在某些情形下,特别是涉及到对经济理论的检验时,就要构造一个规范的经济模型。
经济模型总是由描述各种关系的数理方程构成。
2.经济模型变成计量模型先了解一下计量模型和经济模型有何关系。
与经济分析不同,在进行计量经济分析之前,必须明确函数的形式。
通过设定一个特定的计量经济模型,就解决了经济模型中内在的不确定性。
在多数情况下,计量经济分析是从对一个计量经济模型的设定开始的,而没有考虑模型构造的细节。
一旦设定了一个计量模型,所关心的各种假设便可用未知参数来表述。
3.搜集相关变量的数据4.用计量方法来估计计量模型中的参数,并规范地检验所关心的假设在某些情况下,计量模型还用于对理论的检验或对政策影响的研究。
三、经济数据的结构1.横截面数据(1)横截面数据集,就是在给定时点对个人、家庭、企业、城市、州、国家或一系列其他单位采集的样本所构成的数据集。
有时,所有单位的数据并非完全对应于同一时间段。
在一个纯粹的横截面分析中,应该忽略数据搜集中细小的时间差别。
(2)横截面数据的重要特征①假定它们是从样本背后的总体中通过随机抽样而得到的。
当抽取的样本(特别是地理上的样本)相对总体而言太大时,可能会导致另一种偏离随机抽样的情况。
这种情形中潜在的问题是,总体不够大,所以不能合理地假定观测值是独立抽取的。
伍德里奇《计量经济学导论--现代观点》1
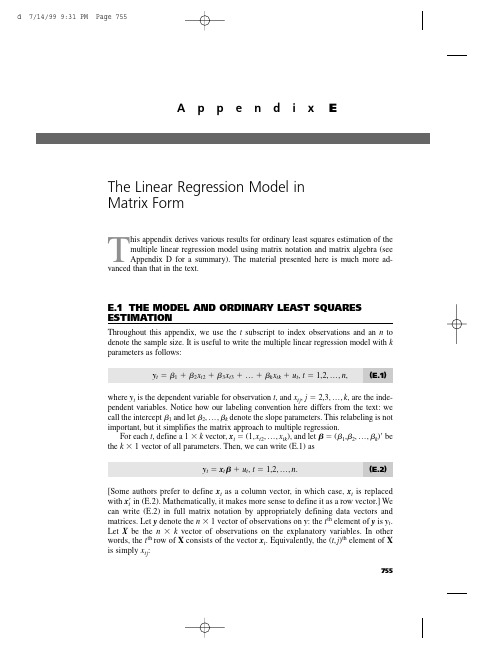
T his appendix derives various results for ordinary least squares estimation of themultiple linear regression model using matrix notation and matrix algebra (see Appendix D for a summary). The material presented here is much more ad-vanced than that in the text.E.1THE MODEL AND ORDINARY LEAST SQUARES ESTIMATIONThroughout this appendix,we use the t subscript to index observations and an n to denote the sample size. It is useful to write the multiple linear regression model with k parameters as follows:y t ϭ1ϩ2x t 2ϩ3x t 3ϩ… ϩk x tk ϩu t ,t ϭ 1,2,…,n ,(E.1)where y t is the dependent variable for observation t ,and x tj ,j ϭ 2,3,…,k ,are the inde-pendent variables. Notice how our labeling convention here differs from the text:we call the intercept 1and let 2,…,k denote the slope parameters. This relabeling is not important,but it simplifies the matrix approach to multiple regression.For each t ,define a 1 ϫk vector,x t ϭ(1,x t 2,…,x tk ),and let ϭ(1,2,…,k )Јbe the k ϫ1 vector of all parameters. Then,we can write (E.1) asy t ϭx t ϩu t ,t ϭ 1,2,…,n .(E.2)[Some authors prefer to define x t as a column vector,in which case,x t is replaced with x t Јin (E.2). Mathematically,it makes more sense to define it as a row vector.] We can write (E.2) in full matrix notation by appropriately defining data vectors and matrices. Let y denote the n ϫ1 vector of observations on y :the t th element of y is y t .Let X be the n ϫk vector of observations on the explanatory variables. In other words,the t th row of X consists of the vector x t . Equivalently,the (t ,j )th element of X is simply x tj :755A p p e n d i x EThe Linear Regression Model inMatrix Formn X ϫ k ϵϭ .Finally,let u be the n ϫ 1 vector of unobservable disturbances. Then,we can write (E.2)for all n observations in matrix notation :y ϭX ϩu .(E.3)Remember,because X is n ϫ k and is k ϫ 1,X is n ϫ 1.Estimation of proceeds by minimizing the sum of squared residuals,as in Section3.2. Define the sum of squared residuals function for any possible k ϫ 1 parameter vec-tor b asSSR(b ) ϵ͚nt ϭ1(y t Ϫx t b )2.The k ϫ 1 vector of ordinary least squares estimates,ˆϭ(ˆ1,ˆ2,…,ˆk ),minimizes SSR(b ) over all possible k ϫ 1 vectors b . This is a problem in multivariable calculus.For ˆto minimize the sum of squared residuals,it must solve the first order conditionѨSSR(ˆ)/Ѩb ϵ0.(E.4)Using the fact that the derivative of (y t Ϫx t b )2with respect to b is the 1ϫ k vector Ϫ2(y t Ϫx t b )x t ,(E.4) is equivalent to͚nt ϭ1xt Ј(y t Ϫx t ˆ) ϵ0.(E.5)(We have divided by Ϫ2 and taken the transpose.) We can write this first order condi-tion as͚nt ϭ1(y t Ϫˆ1Ϫˆ2x t 2Ϫ… Ϫˆk x tk ) ϭ0͚nt ϭ1x t 2(y t Ϫˆ1Ϫˆ2x t 2Ϫ… Ϫˆk x tk ) ϭ0...͚nt ϭ1x tk (y t Ϫˆ1Ϫˆ2x t 2Ϫ… Ϫˆk x tk ) ϭ0,which,apart from the different labeling convention,is identical to the first order condi-tions in equation (3.13). We want to write these in matrix form to make them more use-ful. Using the formula for partitioned multiplication in Appendix D,we see that (E.5)is equivalent to΅1x 12x 13...x 1k1x 22x 23...x 2k...1x n 2x n 3...x nk ΄΅x 1x 2...x n ΄Appendix E The Linear Regression Model in Matrix Form756Appendix E The Linear Regression Model in Matrix FormXЈ(yϪXˆ) ϭ0(E.6) or(XЈX)ˆϭXЈy.(E.7)It can be shown that (E.7) always has at least one solution. Multiple solutions do not help us,as we are looking for a unique set of OLS estimates given our data set. Assuming that the kϫ k symmetric matrix XЈX is nonsingular,we can premultiply both sides of (E.7) by (XЈX)Ϫ1to solve for the OLS estimator ˆ:ˆϭ(XЈX)Ϫ1XЈy.(E.8)This is the critical formula for matrix analysis of the multiple linear regression model. The assumption that XЈX is invertible is equivalent to the assumption that rank(X) ϭk, which means that the columns of X must be linearly independent. This is the matrix ver-sion of MLR.4 in Chapter 3.Before we continue,(E.8) warrants a word of warning. It is tempting to simplify the formula for ˆas follows:ˆϭ(XЈX)Ϫ1XЈyϭXϪ1(XЈ)Ϫ1XЈyϭXϪ1y.The flaw in this reasoning is that X is usually not a square matrix,and so it cannot be inverted. In other words,we cannot write (XЈX)Ϫ1ϭXϪ1(XЈ)Ϫ1unless nϭk,a case that virtually never arises in practice.The nϫ 1 vectors of OLS fitted values and residuals are given byyˆϭXˆ,uˆϭyϪyˆϭyϪXˆ.From (E.6) and the definition of uˆ,we can see that the first order condition for ˆis the same asXЈuˆϭ0.(E.9) Because the first column of X consists entirely of ones,(E.9) implies that the OLS residuals always sum to zero when an intercept is included in the equation and that the sample covariance between each independent variable and the OLS residuals is zero. (We discussed both of these properties in Chapter 3.)The sum of squared residuals can be written asSSR ϭ͚n tϭ1uˆt2ϭuˆЈuˆϭ(yϪXˆ)Ј(yϪXˆ).(E.10)All of the algebraic properties from Chapter 3 can be derived using matrix algebra. For example,we can show that the total sum of squares is equal to the explained sum of squares plus the sum of squared residuals [see (3.27)]. The use of matrices does not pro-vide a simpler proof than summation notation,so we do not provide another derivation.757The matrix approach to multiple regression can be used as the basis for a geometri-cal interpretation of regression. This involves mathematical concepts that are even more advanced than those we covered in Appendix D. [See Goldberger (1991) or Greene (1997).]E.2FINITE SAMPLE PROPERTIES OF OLSDeriving the expected value and variance of the OLS estimator ˆis facilitated by matrix algebra,but we must show some care in stating the assumptions.A S S U M P T I O N E.1(L I N E A R I N P A R A M E T E R S)The model can be written as in (E.3), where y is an observed nϫ 1 vector, X is an nϫ k observed matrix, and u is an nϫ 1 vector of unobserved errors or disturbances.A S S U M P T I O N E.2(Z E R O C O N D I T I O N A L M E A N)Conditional on the entire matrix X, each error ut has zero mean: E(ut͉X) ϭ0, tϭ1,2,…,n.In vector form,E(u͉X) ϭ0.(E.11) This assumption is implied by MLR.3 under the random sampling assumption,MLR.2.In time series applications,Assumption E.2 imposes strict exogeneity on the explana-tory variables,something discussed at length in Chapter 10. This rules out explanatory variables whose future values are correlated with ut; in particular,it eliminates laggeddependent variables. Under Assumption E.2,we can condition on the xtjwhen we com-pute the expected value of ˆ.A S S U M P T I O N E.3(N O P E R F E C T C O L L I N E A R I T Y) The matrix X has rank k.This is a careful statement of the assumption that rules out linear dependencies among the explanatory variables. Under Assumption E.3,XЈX is nonsingular,and so ˆis unique and can be written as in (E.8).T H E O R E M E.1(U N B I A S E D N E S S O F O L S)Under Assumptions E.1, E.2, and E.3, the OLS estimator ˆis unbiased for .P R O O F:Use Assumptions E.1 and E.3 and simple algebra to writeˆϭ(XЈX)Ϫ1XЈyϭ(XЈX)Ϫ1XЈ(Xϩu)ϭ(XЈX)Ϫ1(XЈX)ϩ(XЈX)Ϫ1XЈuϭϩ(XЈX)Ϫ1XЈu,(E.12)where we use the fact that (XЈX)Ϫ1(XЈX) ϭIk . Taking the expectation conditional on X givesAppendix E The Linear Regression Model in Matrix Form 758E(ˆ͉X)ϭϩ(XЈX)Ϫ1XЈE(u͉X)ϭϩ(XЈX)Ϫ1XЈ0ϭ,because E(u͉X) ϭ0under Assumption E.2. This argument clearly does not depend on the value of , so we have shown that ˆis unbiased.To obtain the simplest form of the variance-covariance matrix of ˆ,we impose the assumptions of homoskedasticity and no serial correlation.A S S U M P T I O N E.4(H O M O S K E D A S T I C I T Y A N DN O S E R I A L C O R R E L A T I O N)(i) Var(ut͉X) ϭ2, t ϭ 1,2,…,n. (ii) Cov(u t,u s͉X) ϭ0, for all t s. In matrix form, we canwrite these two assumptions asVar(u͉X) ϭ2I n,(E.13)where Inis the nϫ n identity matrix.Part (i) of Assumption E.4 is the homoskedasticity assumption:the variance of utcan-not depend on any element of X,and the variance must be constant across observations, t. Part (ii) is the no serial correlation assumption:the errors cannot be correlated across observations. Under random sampling,and in any other cross-sectional sampling schemes with independent observations,part (ii) of Assumption E.4 automatically holds. For time series applications,part (ii) rules out correlation in the errors over time (both conditional on X and unconditionally).Because of (E.13),we often say that u has scalar variance-covariance matrix when Assumption E.4 holds. We can now derive the variance-covariance matrix of the OLS estimator.T H E O R E M E.2(V A R I A N C E-C O V A R I A N C EM A T R I X O F T H E O L S E S T I M A T O R)Under Assumptions E.1 through E.4,Var(ˆ͉X) ϭ2(XЈX)Ϫ1.(E.14)P R O O F:From the last formula in equation (E.12), we haveVar(ˆ͉X) ϭVar[(XЈX)Ϫ1XЈu͉X] ϭ(XЈX)Ϫ1XЈ[Var(u͉X)]X(XЈX)Ϫ1.Now, we use Assumption E.4 to getVar(ˆ͉X)ϭ(XЈX)Ϫ1XЈ(2I n)X(XЈX)Ϫ1ϭ2(XЈX)Ϫ1XЈX(XЈX)Ϫ1ϭ2(XЈX)Ϫ1.Appendix E The Linear Regression Model in Matrix Form759Formula (E.14) means that the variance of ˆj (conditional on X ) is obtained by multi-plying 2by the j th diagonal element of (X ЈX )Ϫ1. For the slope coefficients,we gave an interpretable formula in equation (3.51). Equation (E.14) also tells us how to obtain the covariance between any two OLS estimates:multiply 2by the appropriate off diago-nal element of (X ЈX )Ϫ1. In Chapter 4,we showed how to avoid explicitly finding covariances for obtaining confidence intervals and hypotheses tests by appropriately rewriting the model.The Gauss-Markov Theorem,in its full generality,can be proven.T H E O R E M E .3 (G A U S S -M A R K O V T H E O R E M )Under Assumptions E.1 through E.4, ˆis the best linear unbiased estimator.P R O O F :Any other linear estimator of can be written as˜ ϭA Јy ,(E.15)where A is an n ϫ k matrix. In order for ˜to be unbiased conditional on X , A can consist of nonrandom numbers and functions of X . (For example, A cannot be a function of y .) To see what further restrictions on A are needed, write˜ϭA Ј(X ϩu ) ϭ(A ЈX )ϩA Јu .(E.16)Then,E(˜͉X )ϭA ЈX ϩE(A Јu ͉X )ϭA ЈX ϩA ЈE(u ͉X ) since A is a function of XϭA ЈX since E(u ͉X ) ϭ0.For ˜to be an unbiased estimator of , it must be true that E(˜͉X ) ϭfor all k ϫ 1 vec-tors , that is,A ЈX ϭfor all k ϫ 1 vectors .(E.17)Because A ЈX is a k ϫ k matrix, (E.17) holds if and only if A ЈX ϭI k . Equations (E.15) and (E.17) characterize the class of linear, unbiased estimators for .Next, from (E.16), we haveVar(˜͉X ) ϭA Ј[Var(u ͉X )]A ϭ2A ЈA ,by Assumption E.4. Therefore,Var(˜͉X ) ϪVar(ˆ͉X )ϭ2[A ЈA Ϫ(X ЈX )Ϫ1]ϭ2[A ЈA ϪA ЈX (X ЈX )Ϫ1X ЈA ] because A ЈX ϭI kϭ2A Ј[I n ϪX (X ЈX )Ϫ1X Ј]Aϵ2A ЈMA ,where M ϵI n ϪX (X ЈX )Ϫ1X Ј. Because M is symmetric and idempotent, A ЈMA is positive semi-definite for any n ϫ k matrix A . This establishes that the OLS estimator ˆis BLUE. How Appendix E The Linear Regression Model in Matrix Form 760Appendix E The Linear Regression Model in Matrix Formis this significant? Let c be any kϫ 1 vector and consider the linear combination cЈϭc11ϩc22ϩ… ϩc kk, which is a scalar. The unbiased estimators of cЈare cЈˆand cЈ˜. ButVar(c˜͉X) ϪVar(cЈˆ͉X) ϭcЈ[Var(˜͉X) ϪVar(ˆ͉X)]cՆ0,because [Var(˜͉X) ϪVar(ˆ͉X)] is p.s.d. Therefore, when it is used for estimating any linear combination of , OLS yields the smallest variance. In particular, Var(ˆj͉X) ՅVar(˜j͉X) for any other linear, unbiased estimator of j.The unbiased estimator of the error variance 2can be written asˆ2ϭuˆЈuˆ/(n Ϫk),where we have labeled the explanatory variables so that there are k total parameters, including the intercept.T H E O R E M E.4(U N B I A S E D N E S S O Fˆ2)Under Assumptions E.1 through E.4, ˆ2is unbiased for 2: E(ˆ2͉X) ϭ2for all 2Ͼ0. P R O O F:Write uˆϭyϪXˆϭyϪX(XЈX)Ϫ1XЈyϭM yϭM u, where MϭI nϪX(XЈX)Ϫ1XЈ,and the last equality follows because MXϭ0. Because M is symmetric and idempotent,uˆЈuˆϭuЈMЈM uϭuЈM u.Because uЈM u is a scalar, it equals its trace. Therefore,ϭE(uЈM u͉X)ϭE[tr(uЈM u)͉X] ϭE[tr(M uuЈ)͉X]ϭtr[E(M uuЈ|X)] ϭtr[M E(uuЈ|X)]ϭtr(M2I n) ϭ2tr(M) ϭ2(nϪ k).The last equality follows from tr(M) ϭtr(I) Ϫtr[X(XЈX)Ϫ1XЈ] ϭnϪtr[(XЈX)Ϫ1XЈX] ϭnϪn) ϭnϪk. Therefore,tr(IkE(ˆ2͉X) ϭE(uЈM u͉X)/(nϪ k) ϭ2.E.3STATISTICAL INFERENCEWhen we add the final classical linear model assumption,ˆhas a multivariate normal distribution,which leads to the t and F distributions for the standard test statistics cov-ered in Chapter 4.A S S U M P T I O N E.5(N O R M A L I T Y O F E R R O R S)are independent and identically distributed as Normal(0,2). Conditional on X, the utEquivalently, u given X is distributed as multivariate normal with mean zero and variance-covariance matrix 2I n: u~ Normal(0,2I n).761Appendix E The Linear Regression Model in Matrix Form Under Assumption E.5,each uis independent of the explanatory variables for all t. Inta time series setting,this is essentially the strict exogeneity assumption.T H E O R E M E.5(N O R M A L I T Y O Fˆ)Under the classical linear model Assumptions E.1 through E.5, ˆconditional on X is dis-tributed as multivariate normal with mean and variance-covariance matrix 2(XЈX)Ϫ1.Theorem E.5 is the basis for statistical inference involving . In fact,along with the properties of the chi-square,t,and F distributions that we summarized in Appendix D, we can use Theorem E.5 to establish that t statistics have a t distribution under Assumptions E.1 through E.5 (under the null hypothesis) and likewise for F statistics. We illustrate with a proof for the t statistics.T H E O R E M E.6Under Assumptions E.1 through E.5,(ˆjϪj)/se(ˆj) ~ t nϪk,j ϭ 1,2,…,k.P R O O F:The proof requires several steps; the following statements are initially conditional on X. First, by Theorem E.5, (ˆjϪj)/sd(ˆ) ~ Normal(0,1), where sd(ˆj) ϭ͙ෆc jj, and c jj is the j th diagonal element of (XЈX)Ϫ1. Next, under Assumptions E.1 through E.5, conditional on X,(n Ϫ k)ˆ2/2~ 2nϪk.(E.18)This follows because (nϪk)ˆ2/2ϭ(u/)ЈM(u/), where M is the nϫn symmetric, idem-potent matrix defined in Theorem E.4. But u/~ Normal(0,I n) by Assumption E.5. It follows from Property 1 for the chi-square distribution in Appendix D that (u/)ЈM(u/) ~ 2nϪk (because M has rank nϪk).We also need to show that ˆand ˆ2are independent. But ˆϭϩ(XЈX)Ϫ1XЈu, and ˆ2ϭuЈM u/(nϪk). Now, [(XЈX)Ϫ1XЈ]Mϭ0because XЈMϭ0. It follows, from Property 5 of the multivariate normal distribution in Appendix D, that ˆand M u are independent. Since ˆ2is a function of M u, ˆand ˆ2are also independent.Finally, we can write(ˆjϪj)/se(ˆj) ϭ[(ˆjϪj)/sd(ˆj)]/(ˆ2/2)1/2,which is the ratio of a standard normal random variable and the square root of a 2nϪk/(nϪk) random variable. We just showed that these are independent, and so, by def-inition of a t random variable, (ˆjϪj)/se(ˆj) has the t nϪk distribution. Because this distri-bution does not depend on X, it is the unconditional distribution of (ˆjϪj)/se(ˆj) as well.From this theorem,we can plug in any hypothesized value for j and use the t statistic for testing hypotheses,as usual.Under Assumptions E.1 through E.5,we can compute what is known as the Cramer-Rao lower bound for the variance-covariance matrix of unbiased estimators of (again762conditional on X ) [see Greene (1997,Chapter 4)]. This can be shown to be 2(X ЈX )Ϫ1,which is exactly the variance-covariance matrix of the OLS estimator. This implies that ˆis the minimum variance unbiased estimator of (conditional on X ):Var(˜͉X ) ϪVar(ˆ͉X ) is positive semi-definite for any other unbiased estimator ˜; we no longer have to restrict our attention to estimators linear in y .It is easy to show that the OLS estimator is in fact the maximum likelihood estima-tor of under Assumption E.5. For each t ,the distribution of y t given X is Normal(x t ,2). Because the y t are independent conditional on X ,the likelihood func-tion for the sample is obtained from the product of the densities:͟nt ϭ1(22)Ϫ1/2exp[Ϫ(y t Ϫx t )2/(22)].Maximizing this function with respect to and 2is the same as maximizing its nat-ural logarithm:͚nt ϭ1[Ϫ(1/2)log(22) Ϫ(yt Ϫx t )2/(22)].For obtaining ˆ,this is the same as minimizing͚nt ϭ1(y t Ϫx t )2—the division by 22does not affect the optimization—which is just the problem that OLS solves. The esti-mator of 2that we have used,SSR/(n Ϫk ),turns out not to be the MLE of 2; the MLE is SSR/n ,which is a biased estimator. Because the unbiased estimator of 2results in t and F statistics with exact t and F distributions under the null,it is always used instead of the MLE.SUMMARYThis appendix has provided a brief discussion of the linear regression model using matrix notation. This material is included for more advanced classes that use matrix algebra,but it is not needed to read the text. In effect,this appendix proves some of the results that we either stated without proof,proved only in special cases,or proved through a more cumbersome method of proof. Other topics—such as asymptotic prop-erties,instrumental variables estimation,and panel data models—can be given concise treatments using matrices. Advanced texts in econometrics,including Davidson and MacKinnon (1993),Greene (1997),and Wooldridge (1999),can be consulted for details.KEY TERMSAppendix E The Linear Regression Model in Matrix Form 763First Order Condition Matrix Notation Minimum Variance Unbiased Scalar Variance-Covariance MatrixVariance-Covariance Matrix of the OLS EstimatorPROBLEMSE.1Let x t be the 1ϫ k vector of explanatory variables for observation t . Show that the OLS estimator ˆcan be written asˆϭΘ͚n tϭ1xt Јx t ΙϪ1Θ͚nt ϭ1xt Јy t Ι.Dividing each summation by n shows that ˆis a function of sample averages.E.2Let ˆbe the k ϫ 1 vector of OLS estimates.(i)Show that for any k ϫ 1 vector b ,we can write the sum of squaredresiduals asSSR(b ) ϭu ˆЈu ˆϩ(ˆϪb )ЈX ЈX (ˆϪb ).[Hint :Write (y Ϫ X b )Ј(y ϪX b ) ϭ[u ˆϩX (ˆϪb )]Ј[u ˆϩX (ˆϪb )]and use the fact that X Јu ˆϭ0.](ii)Explain how the expression for SSR(b ) in part (i) proves that ˆuniquely minimizes SSR(b ) over all possible values of b ,assuming Xhas rank k .E.3Let ˆbe the OLS estimate from the regression of y on X . Let A be a k ϫ k non-singular matrix and define z t ϵx t A ,t ϭ 1,…,n . Therefore,z t is 1ϫ k and is a non-singular linear combination of x t . Let Z be the n ϫ k matrix with rows z t . Let ˜denote the OLS estimate from a regression ofy on Z .(i)Show that ˜ϭA Ϫ1ˆ.(ii)Let y ˆt be the fitted values from the original regression and let y ˜t be thefitted values from regressing y on Z . Show that y ˜t ϭy ˆt ,for all t ϭ1,2,…,n . How do the residuals from the two regressions compare?(iii)Show that the estimated variance matrix for ˜is ˆ2A Ϫ1(X ЈX )Ϫ1A Ϫ1,where ˆ2is the usual variance estimate from regressing y on X .(iv)Let the ˆj be the OLS estimates from regressing y t on 1,x t 2,…,x tk ,andlet the ˜j be the OLS estimates from the regression of yt on 1,a 2x t 2,…,a k x tk ,where a j 0,j ϭ 2,…,k . Use the results from part (i)to find the relationship between the ˜j and the ˆj .(v)Assuming the setup of part (iv),use part (iii) to show that se(˜j ) ϭse(ˆj )/͉a j ͉.(vi)Assuming the setup of part (iv),show that the absolute values of the tstatistics for ˜j and ˆj are identical.Appendix E The Linear Regression Model in Matrix Form 764。
伍德里奇《计量经济学导论--现代观点》1
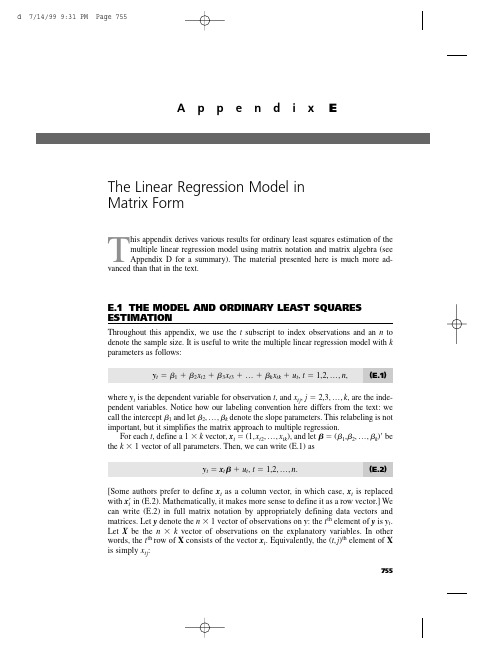
T his appendix derives various results for ordinary least squares estimation of themultiple linear regression model using matrix notation and matrix algebra (see Appendix D for a summary). The material presented here is much more ad-vanced than that in the text.E.1THE MODEL AND ORDINARY LEAST SQUARES ESTIMATIONThroughout this appendix,we use the t subscript to index observations and an n to denote the sample size. It is useful to write the multiple linear regression model with k parameters as follows:y t ϭ1ϩ2x t 2ϩ3x t 3ϩ… ϩk x tk ϩu t ,t ϭ 1,2,…,n ,(E.1)where y t is the dependent variable for observation t ,and x tj ,j ϭ 2,3,…,k ,are the inde-pendent variables. Notice how our labeling convention here differs from the text:we call the intercept 1and let 2,…,k denote the slope parameters. This relabeling is not important,but it simplifies the matrix approach to multiple regression.For each t ,define a 1 ϫk vector,x t ϭ(1,x t 2,…,x tk ),and let ϭ(1,2,…,k )Јbe the k ϫ1 vector of all parameters. Then,we can write (E.1) asy t ϭx t ϩu t ,t ϭ 1,2,…,n .(E.2)[Some authors prefer to define x t as a column vector,in which case,x t is replaced with x t Јin (E.2). Mathematically,it makes more sense to define it as a row vector.] We can write (E.2) in full matrix notation by appropriately defining data vectors and matrices. Let y denote the n ϫ1 vector of observations on y :the t th element of y is y t .Let X be the n ϫk vector of observations on the explanatory variables. In other words,the t th row of X consists of the vector x t . Equivalently,the (t ,j )th element of X is simply x tj :755A p p e n d i x EThe Linear Regression Model inMatrix Formn X ϫ k ϵϭ .Finally,let u be the n ϫ 1 vector of unobservable disturbances. Then,we can write (E.2)for all n observations in matrix notation :y ϭX ϩu .(E.3)Remember,because X is n ϫ k and is k ϫ 1,X is n ϫ 1.Estimation of proceeds by minimizing the sum of squared residuals,as in Section3.2. Define the sum of squared residuals function for any possible k ϫ 1 parameter vec-tor b asSSR(b ) ϵ͚nt ϭ1(y t Ϫx t b )2.The k ϫ 1 vector of ordinary least squares estimates,ˆϭ(ˆ1,ˆ2,…,ˆk ),minimizes SSR(b ) over all possible k ϫ 1 vectors b . This is a problem in multivariable calculus.For ˆto minimize the sum of squared residuals,it must solve the first order conditionѨSSR(ˆ)/Ѩb ϵ0.(E.4)Using the fact that the derivative of (y t Ϫx t b )2with respect to b is the 1ϫ k vector Ϫ2(y t Ϫx t b )x t ,(E.4) is equivalent to͚nt ϭ1xt Ј(y t Ϫx t ˆ) ϵ0.(E.5)(We have divided by Ϫ2 and taken the transpose.) We can write this first order condi-tion as͚nt ϭ1(y t Ϫˆ1Ϫˆ2x t 2Ϫ… Ϫˆk x tk ) ϭ0͚nt ϭ1x t 2(y t Ϫˆ1Ϫˆ2x t 2Ϫ… Ϫˆk x tk ) ϭ0...͚nt ϭ1x tk (y t Ϫˆ1Ϫˆ2x t 2Ϫ… Ϫˆk x tk ) ϭ0,which,apart from the different labeling convention,is identical to the first order condi-tions in equation (3.13). We want to write these in matrix form to make them more use-ful. Using the formula for partitioned multiplication in Appendix D,we see that (E.5)is equivalent to΅1x 12x 13...x 1k1x 22x 23...x 2k...1x n 2x n 3...x nk ΄΅x 1x 2...x n ΄Appendix E The Linear Regression Model in Matrix Form756Appendix E The Linear Regression Model in Matrix FormXЈ(yϪXˆ) ϭ0(E.6) or(XЈX)ˆϭXЈy.(E.7)It can be shown that (E.7) always has at least one solution. Multiple solutions do not help us,as we are looking for a unique set of OLS estimates given our data set. Assuming that the kϫ k symmetric matrix XЈX is nonsingular,we can premultiply both sides of (E.7) by (XЈX)Ϫ1to solve for the OLS estimator ˆ:ˆϭ(XЈX)Ϫ1XЈy.(E.8)This is the critical formula for matrix analysis of the multiple linear regression model. The assumption that XЈX is invertible is equivalent to the assumption that rank(X) ϭk, which means that the columns of X must be linearly independent. This is the matrix ver-sion of MLR.4 in Chapter 3.Before we continue,(E.8) warrants a word of warning. It is tempting to simplify the formula for ˆas follows:ˆϭ(XЈX)Ϫ1XЈyϭXϪ1(XЈ)Ϫ1XЈyϭXϪ1y.The flaw in this reasoning is that X is usually not a square matrix,and so it cannot be inverted. In other words,we cannot write (XЈX)Ϫ1ϭXϪ1(XЈ)Ϫ1unless nϭk,a case that virtually never arises in practice.The nϫ 1 vectors of OLS fitted values and residuals are given byyˆϭXˆ,uˆϭyϪyˆϭyϪXˆ.From (E.6) and the definition of uˆ,we can see that the first order condition for ˆis the same asXЈuˆϭ0.(E.9) Because the first column of X consists entirely of ones,(E.9) implies that the OLS residuals always sum to zero when an intercept is included in the equation and that the sample covariance between each independent variable and the OLS residuals is zero. (We discussed both of these properties in Chapter 3.)The sum of squared residuals can be written asSSR ϭ͚n tϭ1uˆt2ϭuˆЈuˆϭ(yϪXˆ)Ј(yϪXˆ).(E.10)All of the algebraic properties from Chapter 3 can be derived using matrix algebra. For example,we can show that the total sum of squares is equal to the explained sum of squares plus the sum of squared residuals [see (3.27)]. The use of matrices does not pro-vide a simpler proof than summation notation,so we do not provide another derivation.757The matrix approach to multiple regression can be used as the basis for a geometri-cal interpretation of regression. This involves mathematical concepts that are even more advanced than those we covered in Appendix D. [See Goldberger (1991) or Greene (1997).]E.2FINITE SAMPLE PROPERTIES OF OLSDeriving the expected value and variance of the OLS estimator ˆis facilitated by matrix algebra,but we must show some care in stating the assumptions.A S S U M P T I O N E.1(L I N E A R I N P A R A M E T E R S)The model can be written as in (E.3), where y is an observed nϫ 1 vector, X is an nϫ k observed matrix, and u is an nϫ 1 vector of unobserved errors or disturbances.A S S U M P T I O N E.2(Z E R O C O N D I T I O N A L M E A N)Conditional on the entire matrix X, each error ut has zero mean: E(ut͉X) ϭ0, tϭ1,2,…,n.In vector form,E(u͉X) ϭ0.(E.11) This assumption is implied by MLR.3 under the random sampling assumption,MLR.2.In time series applications,Assumption E.2 imposes strict exogeneity on the explana-tory variables,something discussed at length in Chapter 10. This rules out explanatory variables whose future values are correlated with ut; in particular,it eliminates laggeddependent variables. Under Assumption E.2,we can condition on the xtjwhen we com-pute the expected value of ˆ.A S S U M P T I O N E.3(N O P E R F E C T C O L L I N E A R I T Y) The matrix X has rank k.This is a careful statement of the assumption that rules out linear dependencies among the explanatory variables. Under Assumption E.3,XЈX is nonsingular,and so ˆis unique and can be written as in (E.8).T H E O R E M E.1(U N B I A S E D N E S S O F O L S)Under Assumptions E.1, E.2, and E.3, the OLS estimator ˆis unbiased for .P R O O F:Use Assumptions E.1 and E.3 and simple algebra to writeˆϭ(XЈX)Ϫ1XЈyϭ(XЈX)Ϫ1XЈ(Xϩu)ϭ(XЈX)Ϫ1(XЈX)ϩ(XЈX)Ϫ1XЈuϭϩ(XЈX)Ϫ1XЈu,(E.12)where we use the fact that (XЈX)Ϫ1(XЈX) ϭIk . Taking the expectation conditional on X givesAppendix E The Linear Regression Model in Matrix Form 758E(ˆ͉X)ϭϩ(XЈX)Ϫ1XЈE(u͉X)ϭϩ(XЈX)Ϫ1XЈ0ϭ,because E(u͉X) ϭ0under Assumption E.2. This argument clearly does not depend on the value of , so we have shown that ˆis unbiased.To obtain the simplest form of the variance-covariance matrix of ˆ,we impose the assumptions of homoskedasticity and no serial correlation.A S S U M P T I O N E.4(H O M O S K E D A S T I C I T Y A N DN O S E R I A L C O R R E L A T I O N)(i) Var(ut͉X) ϭ2, t ϭ 1,2,…,n. (ii) Cov(u t,u s͉X) ϭ0, for all t s. In matrix form, we canwrite these two assumptions asVar(u͉X) ϭ2I n,(E.13)where Inis the nϫ n identity matrix.Part (i) of Assumption E.4 is the homoskedasticity assumption:the variance of utcan-not depend on any element of X,and the variance must be constant across observations, t. Part (ii) is the no serial correlation assumption:the errors cannot be correlated across observations. Under random sampling,and in any other cross-sectional sampling schemes with independent observations,part (ii) of Assumption E.4 automatically holds. For time series applications,part (ii) rules out correlation in the errors over time (both conditional on X and unconditionally).Because of (E.13),we often say that u has scalar variance-covariance matrix when Assumption E.4 holds. We can now derive the variance-covariance matrix of the OLS estimator.T H E O R E M E.2(V A R I A N C E-C O V A R I A N C EM A T R I X O F T H E O L S E S T I M A T O R)Under Assumptions E.1 through E.4,Var(ˆ͉X) ϭ2(XЈX)Ϫ1.(E.14)P R O O F:From the last formula in equation (E.12), we haveVar(ˆ͉X) ϭVar[(XЈX)Ϫ1XЈu͉X] ϭ(XЈX)Ϫ1XЈ[Var(u͉X)]X(XЈX)Ϫ1.Now, we use Assumption E.4 to getVar(ˆ͉X)ϭ(XЈX)Ϫ1XЈ(2I n)X(XЈX)Ϫ1ϭ2(XЈX)Ϫ1XЈX(XЈX)Ϫ1ϭ2(XЈX)Ϫ1.Appendix E The Linear Regression Model in Matrix Form759Formula (E.14) means that the variance of ˆj (conditional on X ) is obtained by multi-plying 2by the j th diagonal element of (X ЈX )Ϫ1. For the slope coefficients,we gave an interpretable formula in equation (3.51). Equation (E.14) also tells us how to obtain the covariance between any two OLS estimates:multiply 2by the appropriate off diago-nal element of (X ЈX )Ϫ1. In Chapter 4,we showed how to avoid explicitly finding covariances for obtaining confidence intervals and hypotheses tests by appropriately rewriting the model.The Gauss-Markov Theorem,in its full generality,can be proven.T H E O R E M E .3 (G A U S S -M A R K O V T H E O R E M )Under Assumptions E.1 through E.4, ˆis the best linear unbiased estimator.P R O O F :Any other linear estimator of can be written as˜ ϭA Јy ,(E.15)where A is an n ϫ k matrix. In order for ˜to be unbiased conditional on X , A can consist of nonrandom numbers and functions of X . (For example, A cannot be a function of y .) To see what further restrictions on A are needed, write˜ϭA Ј(X ϩu ) ϭ(A ЈX )ϩA Јu .(E.16)Then,E(˜͉X )ϭA ЈX ϩE(A Јu ͉X )ϭA ЈX ϩA ЈE(u ͉X ) since A is a function of XϭA ЈX since E(u ͉X ) ϭ0.For ˜to be an unbiased estimator of , it must be true that E(˜͉X ) ϭfor all k ϫ 1 vec-tors , that is,A ЈX ϭfor all k ϫ 1 vectors .(E.17)Because A ЈX is a k ϫ k matrix, (E.17) holds if and only if A ЈX ϭI k . Equations (E.15) and (E.17) characterize the class of linear, unbiased estimators for .Next, from (E.16), we haveVar(˜͉X ) ϭA Ј[Var(u ͉X )]A ϭ2A ЈA ,by Assumption E.4. Therefore,Var(˜͉X ) ϪVar(ˆ͉X )ϭ2[A ЈA Ϫ(X ЈX )Ϫ1]ϭ2[A ЈA ϪA ЈX (X ЈX )Ϫ1X ЈA ] because A ЈX ϭI kϭ2A Ј[I n ϪX (X ЈX )Ϫ1X Ј]Aϵ2A ЈMA ,where M ϵI n ϪX (X ЈX )Ϫ1X Ј. Because M is symmetric and idempotent, A ЈMA is positive semi-definite for any n ϫ k matrix A . This establishes that the OLS estimator ˆis BLUE. How Appendix E The Linear Regression Model in Matrix Form 760Appendix E The Linear Regression Model in Matrix Formis this significant? Let c be any kϫ 1 vector and consider the linear combination cЈϭc11ϩc22ϩ… ϩc kk, which is a scalar. The unbiased estimators of cЈare cЈˆand cЈ˜. ButVar(c˜͉X) ϪVar(cЈˆ͉X) ϭcЈ[Var(˜͉X) ϪVar(ˆ͉X)]cՆ0,because [Var(˜͉X) ϪVar(ˆ͉X)] is p.s.d. Therefore, when it is used for estimating any linear combination of , OLS yields the smallest variance. In particular, Var(ˆj͉X) ՅVar(˜j͉X) for any other linear, unbiased estimator of j.The unbiased estimator of the error variance 2can be written asˆ2ϭuˆЈuˆ/(n Ϫk),where we have labeled the explanatory variables so that there are k total parameters, including the intercept.T H E O R E M E.4(U N B I A S E D N E S S O Fˆ2)Under Assumptions E.1 through E.4, ˆ2is unbiased for 2: E(ˆ2͉X) ϭ2for all 2Ͼ0. P R O O F:Write uˆϭyϪXˆϭyϪX(XЈX)Ϫ1XЈyϭM yϭM u, where MϭI nϪX(XЈX)Ϫ1XЈ,and the last equality follows because MXϭ0. Because M is symmetric and idempotent,uˆЈuˆϭuЈMЈM uϭuЈM u.Because uЈM u is a scalar, it equals its trace. Therefore,ϭE(uЈM u͉X)ϭE[tr(uЈM u)͉X] ϭE[tr(M uuЈ)͉X]ϭtr[E(M uuЈ|X)] ϭtr[M E(uuЈ|X)]ϭtr(M2I n) ϭ2tr(M) ϭ2(nϪ k).The last equality follows from tr(M) ϭtr(I) Ϫtr[X(XЈX)Ϫ1XЈ] ϭnϪtr[(XЈX)Ϫ1XЈX] ϭnϪn) ϭnϪk. Therefore,tr(IkE(ˆ2͉X) ϭE(uЈM u͉X)/(nϪ k) ϭ2.E.3STATISTICAL INFERENCEWhen we add the final classical linear model assumption,ˆhas a multivariate normal distribution,which leads to the t and F distributions for the standard test statistics cov-ered in Chapter 4.A S S U M P T I O N E.5(N O R M A L I T Y O F E R R O R S)are independent and identically distributed as Normal(0,2). Conditional on X, the utEquivalently, u given X is distributed as multivariate normal with mean zero and variance-covariance matrix 2I n: u~ Normal(0,2I n).761Appendix E The Linear Regression Model in Matrix Form Under Assumption E.5,each uis independent of the explanatory variables for all t. Inta time series setting,this is essentially the strict exogeneity assumption.T H E O R E M E.5(N O R M A L I T Y O Fˆ)Under the classical linear model Assumptions E.1 through E.5, ˆconditional on X is dis-tributed as multivariate normal with mean and variance-covariance matrix 2(XЈX)Ϫ1.Theorem E.5 is the basis for statistical inference involving . In fact,along with the properties of the chi-square,t,and F distributions that we summarized in Appendix D, we can use Theorem E.5 to establish that t statistics have a t distribution under Assumptions E.1 through E.5 (under the null hypothesis) and likewise for F statistics. We illustrate with a proof for the t statistics.T H E O R E M E.6Under Assumptions E.1 through E.5,(ˆjϪj)/se(ˆj) ~ t nϪk,j ϭ 1,2,…,k.P R O O F:The proof requires several steps; the following statements are initially conditional on X. First, by Theorem E.5, (ˆjϪj)/sd(ˆ) ~ Normal(0,1), where sd(ˆj) ϭ͙ෆc jj, and c jj is the j th diagonal element of (XЈX)Ϫ1. Next, under Assumptions E.1 through E.5, conditional on X,(n Ϫ k)ˆ2/2~ 2nϪk.(E.18)This follows because (nϪk)ˆ2/2ϭ(u/)ЈM(u/), where M is the nϫn symmetric, idem-potent matrix defined in Theorem E.4. But u/~ Normal(0,I n) by Assumption E.5. It follows from Property 1 for the chi-square distribution in Appendix D that (u/)ЈM(u/) ~ 2nϪk (because M has rank nϪk).We also need to show that ˆand ˆ2are independent. But ˆϭϩ(XЈX)Ϫ1XЈu, and ˆ2ϭuЈM u/(nϪk). Now, [(XЈX)Ϫ1XЈ]Mϭ0because XЈMϭ0. It follows, from Property 5 of the multivariate normal distribution in Appendix D, that ˆand M u are independent. Since ˆ2is a function of M u, ˆand ˆ2are also independent.Finally, we can write(ˆjϪj)/se(ˆj) ϭ[(ˆjϪj)/sd(ˆj)]/(ˆ2/2)1/2,which is the ratio of a standard normal random variable and the square root of a 2nϪk/(nϪk) random variable. We just showed that these are independent, and so, by def-inition of a t random variable, (ˆjϪj)/se(ˆj) has the t nϪk distribution. Because this distri-bution does not depend on X, it is the unconditional distribution of (ˆjϪj)/se(ˆj) as well.From this theorem,we can plug in any hypothesized value for j and use the t statistic for testing hypotheses,as usual.Under Assumptions E.1 through E.5,we can compute what is known as the Cramer-Rao lower bound for the variance-covariance matrix of unbiased estimators of (again762conditional on X ) [see Greene (1997,Chapter 4)]. This can be shown to be 2(X ЈX )Ϫ1,which is exactly the variance-covariance matrix of the OLS estimator. This implies that ˆis the minimum variance unbiased estimator of (conditional on X ):Var(˜͉X ) ϪVar(ˆ͉X ) is positive semi-definite for any other unbiased estimator ˜; we no longer have to restrict our attention to estimators linear in y .It is easy to show that the OLS estimator is in fact the maximum likelihood estima-tor of under Assumption E.5. For each t ,the distribution of y t given X is Normal(x t ,2). Because the y t are independent conditional on X ,the likelihood func-tion for the sample is obtained from the product of the densities:͟nt ϭ1(22)Ϫ1/2exp[Ϫ(y t Ϫx t )2/(22)].Maximizing this function with respect to and 2is the same as maximizing its nat-ural logarithm:͚nt ϭ1[Ϫ(1/2)log(22) Ϫ(yt Ϫx t )2/(22)].For obtaining ˆ,this is the same as minimizing͚nt ϭ1(y t Ϫx t )2—the division by 22does not affect the optimization—which is just the problem that OLS solves. The esti-mator of 2that we have used,SSR/(n Ϫk ),turns out not to be the MLE of 2; the MLE is SSR/n ,which is a biased estimator. Because the unbiased estimator of 2results in t and F statistics with exact t and F distributions under the null,it is always used instead of the MLE.SUMMARYThis appendix has provided a brief discussion of the linear regression model using matrix notation. This material is included for more advanced classes that use matrix algebra,but it is not needed to read the text. In effect,this appendix proves some of the results that we either stated without proof,proved only in special cases,or proved through a more cumbersome method of proof. Other topics—such as asymptotic prop-erties,instrumental variables estimation,and panel data models—can be given concise treatments using matrices. Advanced texts in econometrics,including Davidson and MacKinnon (1993),Greene (1997),and Wooldridge (1999),can be consulted for details.KEY TERMSAppendix E The Linear Regression Model in Matrix Form 763First Order Condition Matrix Notation Minimum Variance Unbiased Scalar Variance-Covariance MatrixVariance-Covariance Matrix of the OLS EstimatorPROBLEMSE.1Let x t be the 1ϫ k vector of explanatory variables for observation t . Show that the OLS estimator ˆcan be written asˆϭΘ͚n tϭ1xt Јx t ΙϪ1Θ͚nt ϭ1xt Јy t Ι.Dividing each summation by n shows that ˆis a function of sample averages.E.2Let ˆbe the k ϫ 1 vector of OLS estimates.(i)Show that for any k ϫ 1 vector b ,we can write the sum of squaredresiduals asSSR(b ) ϭu ˆЈu ˆϩ(ˆϪb )ЈX ЈX (ˆϪb ).[Hint :Write (y Ϫ X b )Ј(y ϪX b ) ϭ[u ˆϩX (ˆϪb )]Ј[u ˆϩX (ˆϪb )]and use the fact that X Јu ˆϭ0.](ii)Explain how the expression for SSR(b ) in part (i) proves that ˆuniquely minimizes SSR(b ) over all possible values of b ,assuming Xhas rank k .E.3Let ˆbe the OLS estimate from the regression of y on X . Let A be a k ϫ k non-singular matrix and define z t ϵx t A ,t ϭ 1,…,n . Therefore,z t is 1ϫ k and is a non-singular linear combination of x t . Let Z be the n ϫ k matrix with rows z t . Let ˜denote the OLS estimate from a regression ofy on Z .(i)Show that ˜ϭA Ϫ1ˆ.(ii)Let y ˆt be the fitted values from the original regression and let y ˜t be thefitted values from regressing y on Z . Show that y ˜t ϭy ˆt ,for all t ϭ1,2,…,n . How do the residuals from the two regressions compare?(iii)Show that the estimated variance matrix for ˜is ˆ2A Ϫ1(X ЈX )Ϫ1A Ϫ1,where ˆ2is the usual variance estimate from regressing y on X .(iv)Let the ˆj be the OLS estimates from regressing y t on 1,x t 2,…,x tk ,andlet the ˜j be the OLS estimates from the regression of yt on 1,a 2x t 2,…,a k x tk ,where a j 0,j ϭ 2,…,k . Use the results from part (i)to find the relationship between the ˜j and the ˆj .(v)Assuming the setup of part (iv),use part (iii) to show that se(˜j ) ϭse(ˆj )/͉a j ͉.(vi)Assuming the setup of part (iv),show that the absolute values of the tstatistics for ˜j and ˆj are identical.Appendix E The Linear Regression Model in Matrix Form 764。
伍德里奇计量经济学导论第四版
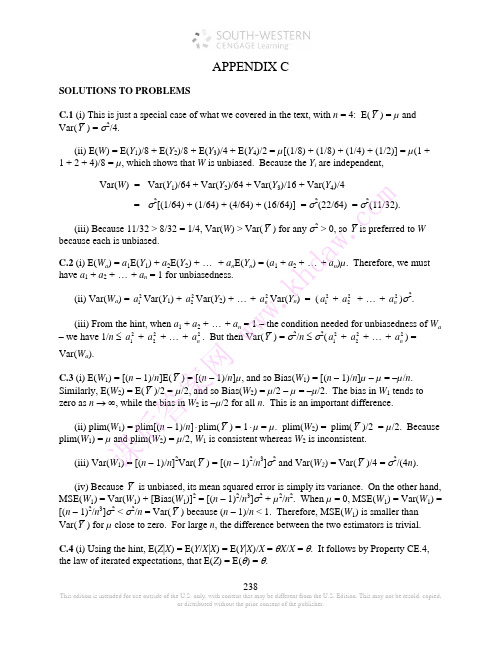
课
后
(ii) plim(W1) = plim[(n – 1)/n] ⋅ plim( Y ) = 1 ⋅ µ = µ. plim(W2) = plim( Y )/2 = µ/2. Because plim(W1) = µ and plim(W2) = µ/2, W1 is consistent whereas W2 is inconsistent.
m
(ii) This follows from part (i) and the fact that the sample average is unbiased for the population average: write
W1 = n −1 ∑ (Yi / X i ) = n −1 ∑ Z i ,
i =1 i =1
n
n
where Zi = Yi/Xi. From part (i), E(Zi) = θ for all i. (iii) In general, the average of the ratios, Yi/Xi, is not the ratio of averages, W2 = Y / X . (This non-equivalence is discussed a bit on page 676.) Nevertheless, W2 is also unbiased, as a simple application of the law of iterated expectations shows. First, E(Yi|X1,…,Xn) = E(Yi|Xi) under random sampling because the observations are independent. Therefore, E(Yi|X1,…,Xn) = θ X i and so
计量经济学总结:计量各小章伍德里奇
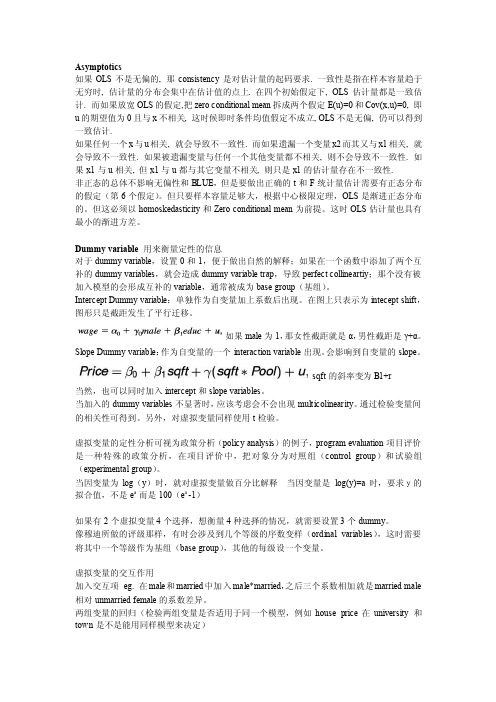
Asymptotics如果OLS不是无偏的, 那consistency是对估计量的起码要求. 一致性是指在样本容量趋于无穷时, 估计量的分布会集中在估计值的点上. 在四个初始假定下, OLS估计量都是一致估计. 而如果放宽OLS的假定,把zero conditional mean拆成两个假定E(u)=0和Cov(x,u)=0, 即u的期望值为0且与x不相关, 这时候即时条件均值假定不成立, OLS不是无偏, 仍可以得到一致估计.如果任何一个x与u相关, 就会导致不一致性. 而如果遗漏一个变量x2而其又与x1相关, 就会导致不一致性. 如果被遗漏变量与任何一个其他变量都不相关, 则不会导致不一致性. 如果x1与u相关, 但x1与u都与其它变量不相关, 则只是x1的估计量存在不一致性.非正态的总体不影响无偏性和BLUE,但是要做出正确的t和F统计量估计需要有正态分布的假定(第6个假定)。
但只要样本容量足够大,根据中心极限定理,OLS是渐进正态分布的。
但这必须以homoskedasticity和Zero conditional mean为前提。
这时OLS估计量也具有最小的渐进方差。
Dummy variable用来衡量定性的信息对于dummy variable,设置0和1,便于做出自然的解释;如果在一个函数中添加了两个互补的dummy variables,就会造成dummy variable trap,导致perfect collineartiy;那个没有被加入模型的会形成互补的variable,通常被成为base group(基组)。
Intercept Dummy variable:单独作为自变量加上系数后出现。
在图上只表示为intecept shift,图形只是截距发生了平行迁移。
如果male为1,那女性截距就是α,男性截距是γ+α。
Slope Dummy variable:作为自变量的一个interaction variable出现。