Lecture2
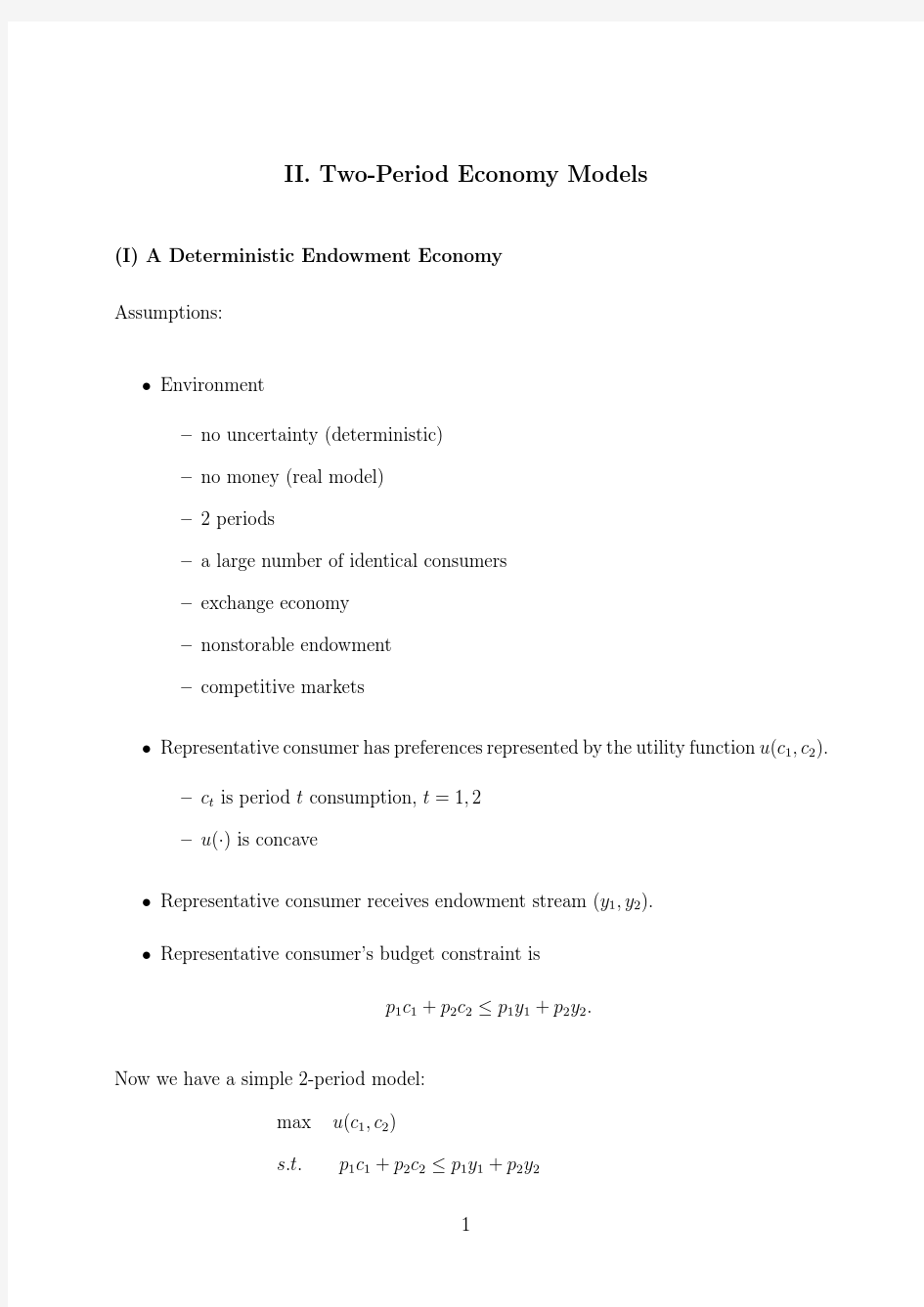
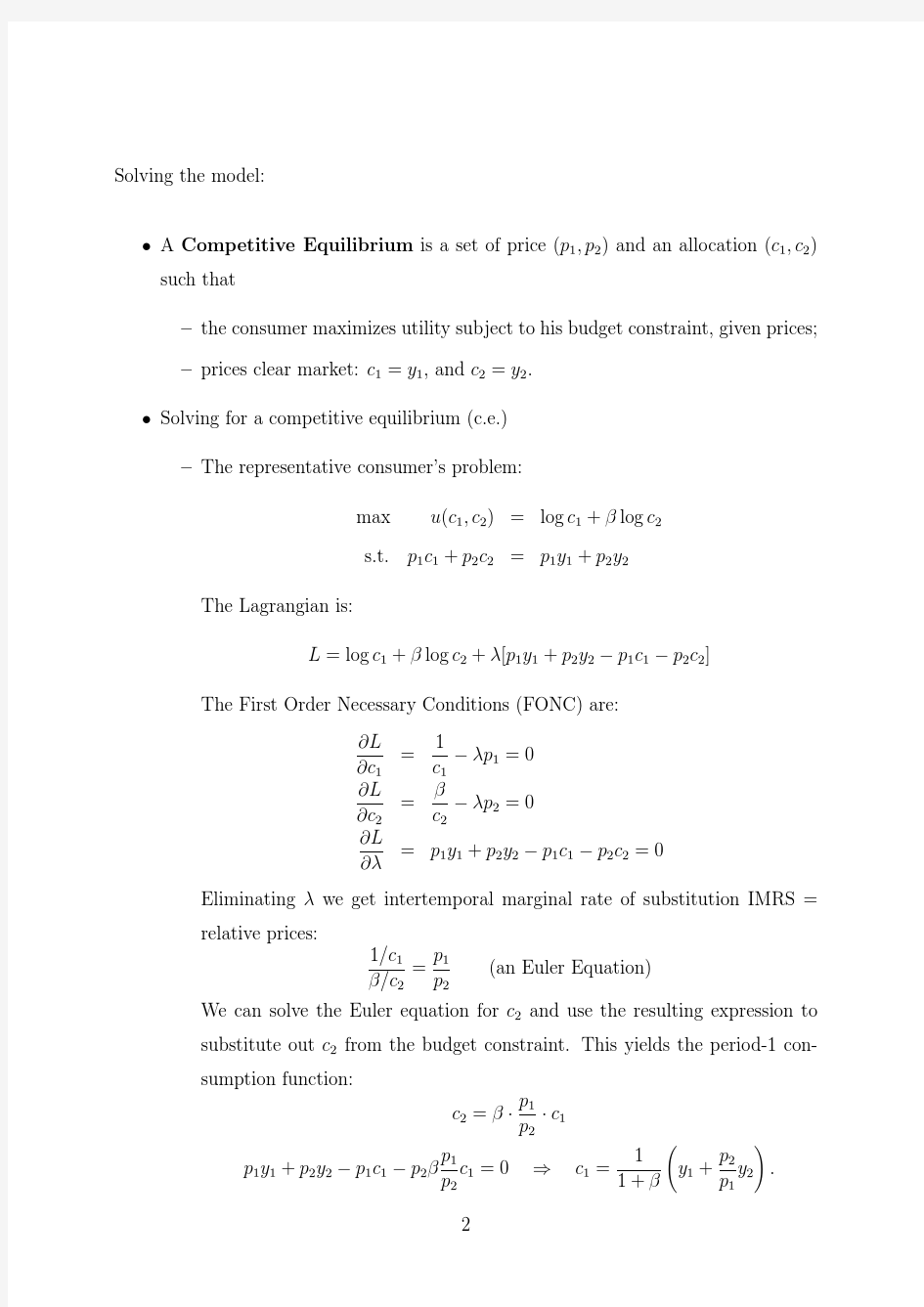
树形DP之个人整理总结
树形DP 二叉苹果树(ural 1108) 题目意思: 有一棵苹果树,苹果树的是一棵二叉树,共N个节点,树节点编号为1~N,编号为1的节点为树根,边可理解为树的分枝,每个分支都长着若干个苹果,现在要要求减去若干个分支,保留M个分支,要求这M个分支的苹果数量最多。 输入: N M 接下来的N-1行是树的边,和该边的苹果数N and M (1 ≤ M < N; 1 < N ≤ 100) 输出: 剩余苹果的最大数量。 input 5 2 1 3 1 1 4 10 2 3 20 3 5 20 output 21 算法: 删除了某个分支,那么这个分支下的子分支也同时删除。 保留M个分支,也就是删除N-M-1个分支。剩余的最多苹果数=总苹果数-剪掉的苹果数。注意本题给的边并没有按照树根--树叶的形式来给,也没有按照树的顺序给出边。本来想一个节点对应一个分支长着的苹果数量,cost[v]就表示v这个节点的苹果数,可以这样做,但是在输入的时候,不知道这个苹果数量是那个节点的,因为不知道哪个是哪个的子结点。所以用了无向图和苹果数加到边上去。 我的解法中:这题的树状DP的整体思想个pku3345是一样的。 有一些不一样的地方要注意一下: 本程序其实不仅仅针对二叉树,可以是任意的树,删除任意个分支都有算。 #include
int N,M; bool visit[MN]; struct NODE { int val; int cost; }; vector 基本句型的转换与扩大 (1)基本句型都是陈述句、肯定句和主动句,这些句型可以转换为疑问句、否定句和被动句。 the tourists have arrived. Have the tourists arrived? The tourists have not arrived. She gave him some money. Did she give him any money? She didn’t give him any money? He was given some money. Some money was given to him (2)句型扩大的语法手段之一是分句的并列。Eg: He was a handicapped child, but he persevered in his studies, and later he become a well-known scholar. He didn’t like us, and everyone knew it, but no one admitted it. (3)句型扩大的另一种语法手段是增加修饰成分和使用从属分句。也就是说利用从属分句来使思想表达复杂化。 The African people have long been our class friends in fighting against hegemonism霸权主义and power politics. The janitor staggered down the stairway, stunned by the bizarre noises and gasping for air. (4)增加的修饰成分还可以再被其他结构(如从属分句)所修饰。 The janitor staggered down the stairway, stunned by the bizarre noises he had just heard upstairs and gasping for air as he hesitantly held on to the banister栏杆.. 注意;有时,分句的并列和从属还可以同时并用。并列与从属两种手段同时并用的结果,往往会产生更加复杂的句子。Eg; When reports came into London Zoo that a wild puma had been spotted forty-five miles south of London, they were not taken seriously, but, as the evidence began to accumulate, experts from the Zoo felt obliged to investigate, for the descriptions given by people who claimed to have seen the puma were extraordinarily similar. 又例如: Furthermore , he is not only critical of the work of others, of his own, since he knows that man is the least reliable of scientific instruments 科学仪表and that a number of factors tend to disturb impartial 不偏不倚的,公正的and objective investigation. 主谓一致(一) “一致”指句子成分之间或词语之间在语法形式上的协调一致关系。主谓一致指主语和谓语动词之间在人称和“数”的方面的一致关系。这又叫做“主动一致”(subject-verb concord). 一、指导原则 主语和谓语动词一致的关系常为三种不同的原则所支配 1、语法一致主谓之间的一致关系主要表现在数的形式上,即用作主语的名词词组中心词 和谓语动词在单复数形式上的一致。 目录2-1随机过程:随机游动与鞅1 Lecture2 日期:2011-03-23作者:杨广宇 摘要 目录 1随机游动、例子2-2 2输光问题2-3 3首达时2-5 4反射原理、反正弦律及对偶2-9 5Wald等式2-15 6附注2-18 6.1鞅停止定理......................................2-18 6.2稳定律的一个例子..................................2-19 1随机游动、例子2-2 1随机游动、例子 随机游动是最简单的随机过程,它同Brown运动、马氏过程、鞅以及L′e vy过程都有紧密的联系,并且在金融、物理、化学和生物信息学中都有广泛和深入的应用。我们这里仅介绍随机游动的一些基本性质。 定义1设{ξn,n≥1}是独立同分布的随机变量序列,其分布函数为F。x∈Z,令 S0=x,S n=x+ n ∑ i=1 ξi,n≥1.(1) 称随机过程{S n,n≥0}为从x出发,具有步长分布F的随机游动。无说明情况下,总取x=0。 习题2随机游动{S n,n≥0}也可以表示为S0=0,S n=S n?1+ξn,n≥1。也即可将S n解释为粒子在时刻n的位置,每个时刻粒子以同样的分布随机的选择一个步长,并跳转到位置S n?1+ξn。试证明S2n?S n与S n相互独立并具有相同的分布。 例3(简单随机游动)若步长随机变量ξ1和?ξ1的分布相同,则说随机游动{S n}是对称随机游动。特别的,若P(ξ1=1)=P(ξ=?1)=1/2,称{S n}为Z上简单(对称)随机游动。若P(ξ1=1)=1?P(ξ=?1)=p=1/2,称{S n}为有偏紧邻随机游动。自然可以考虑Z d上的简单随机游动和有偏紧邻随机游动。 例4(Gauss随机游动)若步长随机变量ξ1取值空间为R,并且ξ1服从标准正态分布N(0,1),则说随机游动{S n}为Gauss随机游动。易知S n服从均值为0,方差为n的正态分布。 习题5由正态分布的可加性知:S n~N(0,n)。试证明n?1S n a.s.??→0。 例6(重尾随机游动)若步长随机变量ξ1是具有重尾分布1的随机变量,则说随机游动{S n}为重尾随机游动。譬如,令ξ1为具有密度函数 f(x)={ α 2 ·1|x|α+1,若|x|≥1; 0,其它. 1重尾随机变量:设X为非负随机变量,若任意的λ>0,EeλX=∞,则称X是重尾的,否则,若存在λ 0使得对任意的0<λ<λ0,EeλX<∞,称随机变量X是轻尾的。用分布来说,若任意的s≥0,lim t→∞[1?F(t+ s)]/[1?F(t)]=1,则说F为重尾分布。关于“重尾”有多种不同形式的定义,直观上,若密度函数依幂指衰减到0,则该分布为重尾分布;若密度函数依指数衰减0,则该分布为轻尾分布。常说的重尾分布一般为幂律分布。 英语主谓一致专项习题及答案解析及解析 一、主谓一致 1.How your winter holiday? —It great. But I tired now A.was, was, am B.is , was, was C.is, is , am D.is, is , was 【答案】A 【解析】 试题分析:句意:你寒假过得怎么样?--好极了。但是我现在很累。根据语境可知,第一个空用一般过去时态,主语是单数,谓语动词用was;其答语也是用一般过去时态,第二个空是一般现在时态,主语是I,谓语动词用am。根据题意,故选A。 考点:考查be动词的用法。 2.It is reported that the population of China smaller in the past few months. The aging(老龄化) is a big problem. More parents are encouraged to give birth to more babies. A.became B.will become C.have become D.has become 【答案】D 【解析】 【详解】 句意:据报道,中国人口在过去的几个月里已经变少了。老龄化是一个大问题。鼓励更多的父母生更多的孩子。A. became变成,一般过去时态;B. will become 将变成,一般将来时;C. have become已经变成,现在完成时;D. has become已经变成,现在完成时,第三人称单数。主语是the population,根据语境可知是现在完成时,其结构has done的形式。根据句意,故选D。 3.In our class _____ of the students _____ girls. A.third fifths; is B.third fifth; are C.three fifth; is D.three fifths; are 【答案】D 【解析】 【详解】 句意:我们班五分之三的学生是女孩。 在英语中表示分数,分子要用基数词,分母用序数词,当分子大于一时,分母用复数形式。五分之三应该是three fifths;另外,分数+名词复数作主语的时候,后面的谓语动词应该用复数。所以选D。 4.(两者之中)任何一个 I don't agree with either of you on these issues. 在这些问题上,你们两个我都不同意。 连接词 conj. 动态规划专题(六):树型动态规划 (重庆巴蜀中学黄新军) 信息学竞赛中通常会出现这样的问题:给一棵树,要求以最少的代价(或取得最大收益)完成给定的操作。有很多问题都是在树和最优性的基础上进行了扩充和加强,从而变成了棘手的问题。这类问题通常规模较大,枚举算法的效率无法胜任,贪心算法不能得到最优解,因此要用动态规划解决。 和一般动态规划问题一样,这类问题的解决要考虑如下三步: 1、确立状态:几乎所以的问题都要保存以某结点为根的子树的情况,但是要根据具体问题考虑是否要加维,加几维,如何加维。 2、状态转移:状态转移的变化比较多,要根据具体问题具体分析,这也是本文例题分析的重点。 3、算法实现: 由于模型建立在树上,即为树型动态规划。 【例题1】二叉苹果树 【问题描述】 有一棵苹果树,如果树枝有分叉,一定是分2叉(就是说没有只有1个儿子的结点),这棵树共有N个结点(叶子点或者树枝分叉点),编号为1-N,树根编号一定是1。 我们用一根树枝两端连接的结点的编号来描述一根树枝的位置。下面是一颗有4个树枝的树: 现在这颗树枝条太多了,需要剪枝。但是一些树枝上长有苹果。给定需要保留的树枝数量,求出最多能留住多少苹果。 【文件输入】 第1行2个数,N和Q(1<=Q<=N,1 助动词的形式与作用 概述:英语常用的助动词(auxiliary verb)有shall,will,should,would,be,have,do等。助动词一般无词义,不能单独作谓语动词。助动词在句中的作用,在于帮助(谓语动词)构成各种时态、语态、语气、否定和疑问结构等。如: China has entered a great new era. 中国已进入了一个伟大的新时期。(帮助构成完成时态) Some boys are playing on the grass. 一些男孩正在草地上玩。 (帮助构成进行时态) Mother is written by Gorky. 《母亲》是高尔基写的。 (帮助构成被动语态) We Shall have the football match if it does not rain.如果不下雨,我们就赛足球。(帮助构成将来时态和否定结构) Do you see my point? 你明白我的意思吗?(帮助构成疑问结构) [注]在否定结构中,not须放在助动词后面。 看完这段之后,希望各位记住一个概念,助动词的作用是:帮助谓语动词实现各种功能,这是核心中核心。 为了便于理解与记忆,个人认为可以把助动词分成两类: 1、专职助动词: shall(should), will(would),can(could),may(might),must, ought to等;(因这些动词只能作助动词使用,没有其它用途。)看见这些动词之后,就可以直接期待其后会出现“谓语动词”了。这也可用于我们判断句子结构,简单不出错。 2、兼职助动词:be, have, do, dare, need等。(这些动词既可以用作助动词,也可用作谓语动词,助动词并不是他们唯一的作用。) 先讲第一类,专职助动词(情态动词)的用法。 情态动词的用法很多都是相同的,不必一项项学,我们先把这些相同的作用归纳到一起。 1、shall、will可用于表达一般将来时;这个就不多作说明了; 2、都可忽略主语的人称和数,不必采取相应的变化。 3、一般情况下,后面都需加动词原形。 (2、3)例:I/He/She/They can swim. 4、表示推测,表可能:may, can, must。 主谓一致练习题答案解析(30题) 1.Thereisalwaysapossibilitythattheinfectionwillreturn,butsofarthere__beennosignsoftrouble. A.has B.have C.are D.had 答案:B. 解析:在由notonly…butalso…,notjust…but…,or,either…or…,neither…nor…连接主语的句子中及在therebe句型中,谓语动词的单复数按就近原则处理,即按与谓语动词最靠近的那个主语来确定谓 语动词的单复数形式。 2.Thiskindofstories____instructivewhilestoriesofthatkind____harmfultochildren. A.is;seems B.are;seem C.is;seem D.are;seems 解析: 解析:“许解析:当 解析:每年”, 解析: 的, 故第一空格要填like。 7.NotonlyIbutalsoJaneandMary____tiredofhavingoneexaminationafteranother. A.is B.are C.am D.be 答案:B. 解析:notonly…butalso…连接两个并列主语时,句子的谓语根据就近的原则处理。 8.One-thirdofthearea____coveredwithgreentrees.Aboutseventypercentofthetrees____beenplanted. A.are;have B.is;has C.is;have D.are;has 答案:C. 解析:“分数或百分数+ofthe+名词或代词”作主语时,谓语动词的单、复数形式取决于名词或代词 的单、复数形式。 9.Twohundredandfiftypounds____toounreasonableapriceforasecond-handcar. A.is B.are C.were D.be 树形图详细讲解 1. Indicate the category of each word in the following sentences. a) The old lady suddenly left. Det A N Qual V b) The car stopped at the end of the road. Det N V P Det N P Det N c) The snow might have blocked the road. Det N Aux Aux V Det N d) He never appears quite mature. N Qual V Deg A 2. The following phrases include a head, a complement, and a specifier. Draw the appropriate tree structure for each. a) full of people AP A P N full of people b) a story about a sentimental girl NP NP PP Det N P NP Det A N a story about a sentimental girl c) often read detective stories VP Qual V NP A N often read detective stories d) the argument against the proposals NP NP PP Det N P NP Det N the argument against the proposals e) move towards the window VP V PP P Det N move towards the window 3. Draw phrase structure trees for each of the following sentences. a) The jet landed. InflP(=S) NP Infl VP Det N Pst V The jet landed b) Mary became very ill. InflP(=S) NP Infl VP N Pst V AP Deg A Mary became very ill 英语主谓一致专题训练答案及解析 一、主谓一致 1.—It’s a pity that my teachers parents allow me to swim alone. —After all you are too young, safety first. A.either...or B.neither… nor C.both...and D.not only...but also 【答案】B 【解析】 【详解】 句意:——很遗憾,我的老师和父母都不允许我独自游泳。——毕竟你还太小,安全第一。 考查不定代词辨析。A. 要么……要么,表示二者择其一;B. 既不……也不,表示两者都不; C. …和…(两者)都; D. 不仅……而且。根据回答After all you are too young, safety first.可知老师和父母都不允许我独自游泳,故选B。 2.There is only one position. The boss has to choose Jack Tom to be a manager. A.both; and B.neither; nor C.either; or D.not only; but also 【答案】C 【解析】 句意:只有一个位置,老板必须选择或者杰克或者汤姆作为经理。根据句意因为只有一个位置,结合选项,推测意思是老板不得不选择杰克或汤姆当经理,即固定短语either…or…,或者…或者,故答案选C。 点睛:A. both; and两者都…,连接两个不同的词语作主语时,谓语动词用复数;B. neither; nor既不…也不…;C. either; or或者…或者…;D. not only; but also不仅…而且…;B、C、D三个选项在连接两个不同的词语作主语时,其谓语动词应与最近的一个主语在人称和数上保持一致,就是我们所说的“就近原则”。本题根据句意可知C选项符合题意,故答案选C。 3.There a basketball game between Class 8 and Class 12 tomorrow afternoon. A.is B.has C.will be D.will have 【答案】C 【解析】 【详解】 句意:明天下午在8班和12班之间将有一场篮球比赛。A. is是,单数;B. has有,第三人称单数;C. will be将是;D. will have将有。这里是there be句型,不能出现have/has,排除BD;根据时间状语tomorrow afternoon.可知用一般将来时,其结构是there will be+主语+介词短语。根据题意,故选C。 4.Smog and haze is a kind of air pollution. It _______ people _____ about their health. 最新主谓一致考点解析(Word版附答案) 一、主谓一致 1.______________ something wrong with my bike. Can I use yours? A.It is B.It was C.There is D.There was 【答案】C 【解析】 【详解】 句意:我的自行车出毛病了。我可以用你的吗? 考查there be句型的用法。句型There is something wrong with sb/sth.意思是某人或某物有问题。根据下文“Can I use yours?”一般现在时,可知此句使用一般现在时,故排除D(一般过去时),用there is(一般现在时)。选C。 2.Fifty percent of my monthly pocket money _________ spent on entertainment. A.am B.is C.are D.be 【答案】B 【解析】 【详解】 句意:我每个月零花钱中的50%花费在了娱乐上。am用于主语为第一人称I的时候;is用于主语是单数时;are用于主语是复数时;be是am, is和are的原形。该句的主语为Fifty percent of my monthly pocket money,分数或者百分数+of+名词作主语时,谓语动词的单复数形式应与名词形式一致,这里money是不可数名词,谓语动词应用单数,故应选B。 3.My sister with my parents _______dumplings when I got home yesterday. A.are making B.is making C.was making D.were making 【答案】C 【解析】 试题分析:句意:当我昨天下午到家的时候,我的姐姐和我的父母正在包饺子。with连接句子的主语时,应该与with前的主语一致,谓语动词应该用单数形式,根据时间状语可知,这里应该用过去进行时,故选C。 考点:考查动词的时态。 4.Peter with his classmates ____ for the bus when the earthquake happened. A.is waiting B.was waiting C.are waiting D.were waiting 【答案】B 【解析】 【详解】 句意:地震发生时,彼得和同学正在等公共汽车。选项A/C是现在进行时;选项B/D是过去进行时。根据when the earthquake happened. 地震发生时。彼得和同学正在等公共汽 Lecture2Diction Part1Varieties of English 1.1Variation in English 1.2Style&Register(P14) Formal spoken Address at Gettysburg,1863 Fourscore and seven years ago our fathers brought forth on this continent a new nation,conceived in liberty and dedicated to the proposition that all men are created equal.Now we are engaged in a great civil war,testing whether that nation,or any nation so conceived and so dedicated,can long endure…. ...It is rather for us to be here dedicated to the great task remaining before us—that from these honored dead we take increased devotion to that cause for which they gave the last full measure of devotion;that we here highly resolve that these dead shall not have died in vain;that this nation,under God,shall have a new birth of freedom;and that government of the people,by the people,for the people,shall not perish from the earth. 1.3Spoken&written(1a—6b,P14) Spoken:More informal,More personal,Less concise,Less organized Active voice,Simple connectors,wordy Written:More formal,More impersonal,More precise,Economical in the use of words,Passive voice,Complex connectors,concise More example: (a)Cleared,the site would be very valuable. (b)If it were cleared,the site would be very valuable.(written) 1.4Formal&Informal Word choice (a)The concert concluded with a performance of Beethoven’s5th symphony.(formal) (b)They ended the concert with Beethoven’s5th symphony. Grammar use (a)Bill speaks French more fluently than I.(formal) (b)Bill speaks French more fluently than me. 1.5General English A middle course between formal and informal levels Usually the best choice for college or business writing Formal general informal Automobile car wheels Visage face mug Odious offensive gross Attire clothes threads Spectacles glasses specs In your dictionary,you may find special usage labels for words or particular definitions of words that differ from the general English vocabulary. 主谓一致练习题答案解析(30题) 1. There is always a possibility that the infection will return, but so far there __ been no signs of trouble. A. has B. have C. are D. had 答案:B. 解析:在由not only…but also…, not just…but…, or, either…or…, neither…nor…连接主语的句子中及在there be句型中,谓语动词的单复数按就近原则处理,即按与谓语动词最靠近的那个主语来确定谓语动词的单复数形式。 2. This kind of stories ____ instructive while stories of that kind ____ harmful to children. A. is; seems B. are; seem C. is; seem D. are; seems 答案:C. 解析:this kind of stories的中心词为kind,是单数;stories of that kind的中心词为stories,是复数,故选C。 3. The number of people invited ____ fifty, but a number of them ____ absent for different reasons. A. were; was B. was; was C. was; were D. were; were 答案:C. 解析:“the number of+复数名词”意思是“……的数量”,谓语用单数;“a number of+复数名词”意思是“许多……”,谓语用复数。 4. A poet and artist ____ coming to speak to us about Chinese literature and painting tomorrow afternoon. A. is B. are C. was D. were 答案:A. 解析:当and连结的两个名词是指同一个人或同一件事,and后的名词前没有冠词,谓语动词应该用单数形式;在and后面的名词前有冠词,谓语就用复数形式。 句意:一位诗人兼艺术家明天下午要来给我们作关于中国文学和绘画方面的报告。 5. The average of students of the school going to university each year ____ over 600. A. are B. has been C. have been D. is 答案:D. 解析:“the average of+复数名词”作主语与单数动词连用,排除A、C。根据时间状语each year“每年”,句子应用一般现在时,选D。 6. Jack, as well as his friends who ____ football games, ____ travelled with the team. A. likes; has B. like; have C. like; has D. likes; have 答案:C. 解析:as well as连接的名词作主语所强调的是它前面的名词,故谓语动词要和该名词保持一致。本题的主语是Jack,故谓语动词要用has travelled;who之后从句的实际主语是先行词his friends的,故第一空格要填like。 7. Not only I but also Jane and Mary ____ tired of having one examination after another. A. is B. are C. am D. be 答案:B. 解析:not only…but also…连接两个并列主语时,句子的谓语根据就近的原则处理。 8. One-third of the area ____ covered with green trees. About seventy percent of the trees ____ been planted. A. are; have B. is; has C. is; have D. are; has 1/62 建工学院土木系Dept. of Civil Engineering Autumn 2008 Solution to Lecture 2 Dr. Shao Weiyun Dept. of Civil Engineering Email:shaowy@https://www.360docs.net/doc/229276568.html, 2/62 建工学院土木系Dept. of Civil Engineering Autumn 2008 2.10 The basic elements of a hydraulic press are shown in Fig. P2.10.The plunger has an area of 1 in.2, and a force, F 1, can be applied to the plunger through a lever mechanism having a mechanical advantage of 8 to 1. If the large piston has an area of 150 in.2, what load, F 2, can be raised by a force of 30 lb applied to the lever? Neglect the hydrostatic pressure variation. 3/62 建工学院土木系 Dept. of Civil Engineering Autumn 2008 2.16A U-tube manometer is connected to a closed tank as shown in Fig. P2.16. The air pressure in the tank is 0.50 psi and the liquid in the tank is oil (γ= 54.0 lb/ft3). The pressure at point A is 2.00 psi. Determine: (a) the depth of oil, z , and (b) the differential reading, h , on the manometer. 4/62 建工学院土木系 Dept. of Civil Engineering Autumn 2008 2.21?The inverted U-tube manometer of Fig. P2.21 contains oil (SG = 0.9) and water as shown. The pressure differential between pipes A and B , pA ?pB , is ?5 kPa. Determine the differential reading, h . .主谓一致 主谓一致是指一个句子的主语和谓语动词保持数的一致关系。英语主谓一致一般要遵循三条原则,即:语法一致原则;意义一致原则和就近原则。 1.语法一致原则 语法一致原则是指谓语动词和它的主语在语法形式上必须取得一致,即主语是单数形式,谓语动词用单数形式,主语为复数形式,谓语动词也用复数形式,如: Tom doesn’t like swimming. 汤姆不喜欢游泳。 Jane and Mary look alike. 简和玛丽看起来很像。 2.意义一致原则 意义一致原则是指谓语动词和主语的一致,同由主语所表达的单数概念来决定,而不是根据主语的实际语法形式。 有时主语的语法形式是单数,但所表达的概念是复数意义,这时动词应采用复数形式,如: The football team are having breakfast now. 足球队员们在吃早饭。 The news was exciting. 这条消息令人振奋。 3.就近原则 就近原则指谓语动词的人称和数要在形式上与最靠近它的那个名词或代词取得一致。当一个句子有两个主语而它们又是either...or…, neither…nor…, not only…but also…, or 等连接时,谓语动词与靠近它的主语保持一致,如: Either your students or Mr Wang knows this. 你的学生们或王老师知道这件事。 Neither Jim nor his friends were interested in this film. 汤姆和他的朋友们都对这部电影不感兴趣。 主谓一致这三个原则中有很多需要注意的问题, 1.主语为集体名词 (1)某些集体名词既可表示单数也可表示复数。如果集体名词作为一个整体看待,谓语动词用单数形式,如果就其中一 个成员而言,谓语动词用复数形式,如: Our group were discussing heatedly on the subject. 我们小组成员正对这个问题进行热烈的讨论。(group 指小组成员)Our group is made up of four girls and five boys. 我们小组有4个女孩和5个男孩组成。(group 指小组整体) The population in China is very large, and one third of the population are farmers. 中国的人口很多,其中三分之一是农民。(前一个population 指整体,后一个population 指成员) (2)某些集体名词如people, police, cattle, youth 等常作复数看待,谓语动词与复数形式的谓语搭配,如: The police are searching for him. 警察正在搜寻他。 The cattle were driven away from the hill. 那些牲畜从山上被赶走了。 3.某些名词如equipment(设备),furniture(家具),jewelry(珠宝),clothing(衣服),machinery(机械),表示单数 概念,谓语动词要相应地采用单数形式,如: A lot of equipment has been replaced by the new equipment. 许多旧设备已经被新设备代替了。 All the machinery in the factory was controlled by robots. 这个厂所有的机械都由机器人来控制的。 2.主语为代词 (1).有些代词尽管意义上是多数,但谓语动词要用单数形式,这类代词有:either, neither, each, another, somebody, someone, something, anything, anyone, everyone, everything, everybody, no one, nothing, nobody 等,如: Neither likes the friends of the other. 两人都不喜欢对方的朋友。 Everything around us is matter. 我们周围所有的东西都是物质。 注意: ①在neither of 与either of 的结构中,谓语动词可用单数形式也可用复数形式,如: Neither of them was / were in good health, but both worked hard. 他们俩身体都不好,但工作却都很努力。 ②each 用于单数名词前,谓语动词用单数形式, each 用于复数主语后,谓语动词用复数形式。each of, each one of 后 接复数名词或代词,但谓语动词常用单数形式。 Each (one) of the houses was different. 每座房子都不同。 Each student has been given their own e-mail address. 每个学生都得到了一个自己的电子邮件地址。第二讲 基本句型的转换与扩大 主谓一致(一)
SP(Lecture2)
英语主谓一致专项习题及答案解析及解析
动态规划专题(六):树型动态规划
张琮逻辑英语第二讲 助动词的形式与作用
主谓一致 参考答案 解析
最新英语语言学树型图详细讲解
英语主谓一致专题训练答案及解析
最新 主谓一致考点解析(Word版附答案)
Lecture2 Diction
主谓一致 答案 解析 (2)
Lecture2_Solution
主谓一致用法详解及练习题 附答案