马尔科夫转换模型例子
马尔可夫模型 举例

马尔可夫模型举例
马尔可夫模型是一种用来描述随机事件序列的数学模型。
其基本假设是当前状态只与前一状态有关,而与更早的状态无关。
这种模型在许多领域中都有应用,比如语言处理、金融、天气预测等。
以下是马尔可夫模型在天气预测中的一个例子:
假设有三种天气状态:晴天、多云和雨天。
每天的天气状态都只与前一天的状态有关,具体转移概率如下:
| 当前状态下一天状态 | 晴天 | 多云 | 雨天 |
| --------------------- | ---- | ---- | ---- |
| 晴天 | 0.8 | 0.1 | 0.1 |
| 多云 | 0.4 | 0.4 | 0.2 |
| 雨天 | 0.2 | 0.3 | 0.5 | 例如,如果今天是晴天,那么明天是多云的概率是0.1,雨天的概率是0.1,晴天的概率是0.8。
如果我们已知今天是晴天,那么未来几天的天气预测可以通过马尔可夫模型计算。
比如,如果我们想知道三天后的天气预测,我们可以使用矩阵乘法:
```
[0.8 0.1 0.1] [0.8 0.1 0.1] [0.8 0.1 0.1] [0.8 0.1 0.1]
[0.4 0.4 0.2] * [0.8 0.1 0.1] = [0.68 0.16 0.16]
[0.2 0.3 0.5] [0.8 0.1 0.1] [0.49 0.22 0.29]
```
结果表明,三天后的天气预测中,晴天的概率是0.49,多云的概率是0.22,雨天的概率是0.29。
通过马尔可夫模型,我们可以根据过去的天气状态,预测未来的天气情况。
这对于农业、旅游等领域都有重要的应用。
马尔可夫模型实例
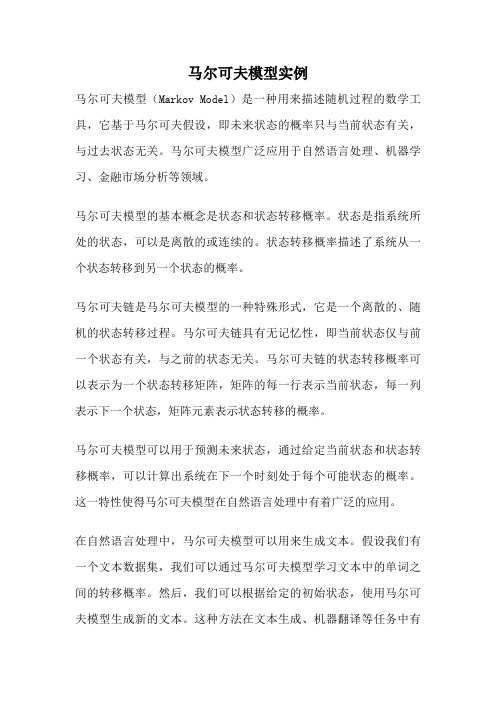
马尔可夫模型实例马尔可夫模型(Markov Model)是一种用来描述随机过程的数学工具,它基于马尔可夫假设,即未来状态的概率只与当前状态有关,与过去状态无关。
马尔可夫模型广泛应用于自然语言处理、机器学习、金融市场分析等领域。
马尔可夫模型的基本概念是状态和状态转移概率。
状态是指系统所处的状态,可以是离散的或连续的。
状态转移概率描述了系统从一个状态转移到另一个状态的概率。
马尔可夫链是马尔可夫模型的一种特殊形式,它是一个离散的、随机的状态转移过程。
马尔可夫链具有无记忆性,即当前状态仅与前一个状态有关,与之前的状态无关。
马尔可夫链的状态转移概率可以表示为一个状态转移矩阵,矩阵的每一行表示当前状态,每一列表示下一个状态,矩阵元素表示状态转移的概率。
马尔可夫模型可以用于预测未来状态,通过给定当前状态和状态转移概率,可以计算出系统在下一个时刻处于每个可能状态的概率。
这一特性使得马尔可夫模型在自然语言处理中有着广泛的应用。
在自然语言处理中,马尔可夫模型可以用来生成文本。
假设我们有一个文本数据集,我们可以通过马尔可夫模型学习文本中的单词之间的转移概率。
然后,我们可以根据给定的初始状态,使用马尔可夫模型生成新的文本。
这种方法在文本生成、机器翻译等任务中有着重要的应用。
马尔可夫模型还可以用于词性标注。
词性标注是指为文本中的每个词汇确定其词性。
通过马尔可夫模型,我们可以根据给定的句子和词性转移概率,计算出每个词汇的最可能词性。
这种方法在自然语言处理中的词性标注任务中被广泛使用。
除了自然语言处理,马尔可夫模型还在金融市场分析中有着重要的应用。
通过建立金融市场的马尔可夫模型,可以预测股票、外汇等金融产品的价格走势。
这种方法在金融领域的交易策略制定中起着重要的作用。
马尔可夫模型的应用还不局限于上述领域,还可以用于图像处理、音频处理等各种领域。
通过马尔可夫模型,我们可以对各种随机过程进行建模和预测,提高系统的性能和效率。
马尔可夫模型是一种描述随机过程的数学工具,它基于马尔可夫假设,可以用来预测未来状态。
马尔可夫预测模型案例

马尔可夫预测模型案例话说在一个充满活力的大学校园里,有个神奇的食堂。
这个食堂每天都供应好多不同的菜品,而我们就可以用马尔可夫预测模型来玩点有趣的分析。
咱先看看食堂有啥菜品呢,有红烧肉、炒青菜、番茄炒蛋、麻婆豆腐之类的。
我们假设这个学校的学生在选择菜品的时候是有一定“规律”的,这个规律就可以用马尔可夫模型来研究。
就拿小明同学来说吧。
我们发现啊,如果小明今天吃了红烧肉,那他明天选择炒青菜的概率是0.3,继续选择红烧肉的概率是0.2,选择番茄炒蛋的概率是0.4,选麻婆豆腐的概率是0.1。
这就像是一种菜品转换的魔法概率。
那我们怎么用这个模型预测呢?比如说,我们知道这个礼拜一小明吃了红烧肉。
那我们就可以根据这个初始状态(礼拜一吃红烧肉)和我们统计出来的那些菜品转换概率,来预测他礼拜二可能吃啥。
按照概率算的话,他有0.2的可能继续吃红烧肉,0.3的可能吃炒青菜,0.4的可能吃番茄炒蛋,0.1的可能吃麻婆豆腐。
要是我们想预测礼拜三他吃啥呢?那就更复杂一点啦。
我们得先算出礼拜二他各种选择的概率下,礼拜三的选择概率。
比如说,如果礼拜二他按照概率真的吃了炒青菜,那从炒青菜这个状态转换到其他菜品又有不同的概率,像从炒青菜再到红烧肉的概率可能是0.15,到番茄炒蛋可能是0.35,到麻婆豆腐可能是0.2,继续吃炒青菜是0.3。
我们就得把礼拜一吃红烧肉之后礼拜二所有可能的菜品选择,以及从这些选择再到礼拜三的菜品选择概率都考虑进去,最后算出礼拜三他吃每个菜品的综合概率。
再学校食堂的大厨想知道下周大概要准备多少份麻婆豆腐。
他就可以用这个马尔可夫预测模型,根据之前同学们的菜品选择习惯,来预测下一周有多少同学可能会选择麻婆豆腐,这样就可以提前准备合适的食材,避免浪费或者不够吃的情况啦。
这个马尔可夫预测模型就像是一个菜品选择的小预言家,能根据之前的情况预测未来的可能性,是不是还挺有趣的呢?在一个奇妙的城市里,天气就像一个调皮的孩子,有时候晴空万里,有时候又阴云密布。
马尔科夫模型(转载)
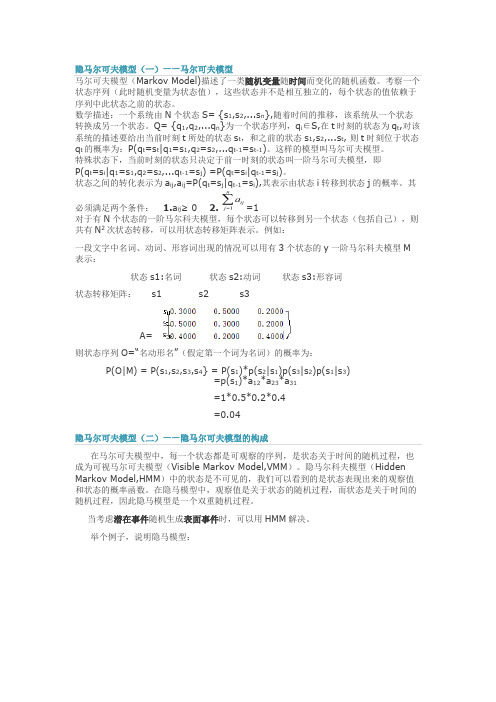
隐马尔可夫模型(一)——马尔可夫模型马尔可夫模型(Markov Model)描述了一类随机变量随时间而变化的随机函数。
考察一个状态序列(此时随机变量为状态值),这些状态并不是相互独立的,每个状态的值依赖于序列中此状态之前的状态。
数学描述:一个系统由N个状态S= {s1,s2,...s n},随着时间的推移,该系统从一个状态转换成另一个状态。
Q= {q1,q2,...q n}为一个状态序列,q i∈S,在t时刻的状态为q t,对该系统的描述要给出当前时刻t所处的状态s t,和之前的状态s1,s2,...s t, 则t时刻位于状态q t的概率为:P(q t=s t|q1=s1,q2=s2,...q t-1=s t-1)。
这样的模型叫马尔可夫模型。
特殊状态下,当前时刻的状态只决定于前一时刻的状态叫一阶马尔可夫模型,即P(q t=s i|q1=s1,q2=s2,...q t-1=s j) =P(q t=s i|q t-1=s j)。
状态之间的转化表示为a ij,a ij=P(q t=s j|q t-1=s i),其表示由状态i转移到状态j的概率。
其必须满足两个条件: 1.a ij≥ 0 2.=1对于有N个状态的一阶马尔科夫模型,每个状态可以转移到另一个状态(包括自己),则共有N2次状态转移,可以用状态转移矩阵表示。
例如:一段文字中名词、动词、形容词出现的情况可以用有3个状态的y一阶马尔科夫模型M 表示:状态s1:名词状态s2:动词状态s3:形容词状态转移矩阵: s1 s2 s3A=则状态序列O=“名动形名”(假定第一个词为名词)的概率为:P(O|M) = P(s1,s2,s3,s4} = P(s1)*p(s2|s1)p(s3|s2)p(s1|s3)=p(s1)*a12*a23*a31=1*0.5*0.2*0.4=0.04在马尔可夫模型中,每一个状态都是可观察的序列,是状态关于时间的随机过程,也成为可视马尔可夫模型(Visible Markov Model,VMM)。
马尔可夫区制转换向量自回归模型
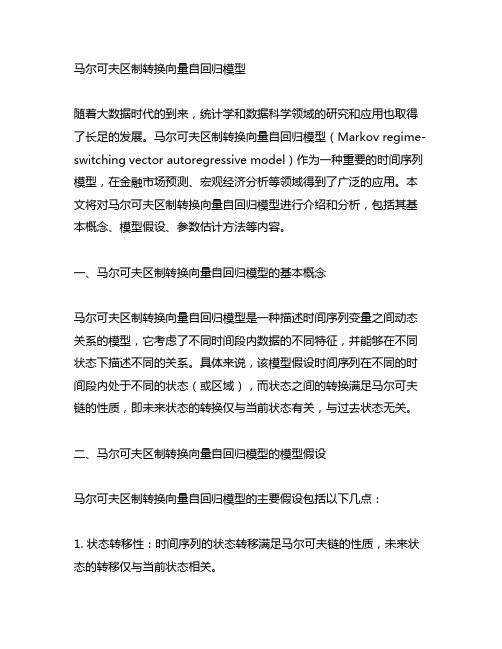
马尔可夫区制转换向量自回归模型随着大数据时代的到来,统计学和数据科学领域的研究和应用也取得了长足的发展。
马尔可夫区制转换向量自回归模型(Markov regime-switching vector autoregressive model)作为一种重要的时间序列模型,在金融市场预测、宏观经济分析等领域得到了广泛的应用。
本文将对马尔可夫区制转换向量自回归模型进行介绍和分析,包括其基本概念、模型假设、参数估计方法等内容。
一、马尔可夫区制转换向量自回归模型的基本概念马尔可夫区制转换向量自回归模型是一种描述时间序列变量之间动态关系的模型,它考虑了不同时间段内数据的不同特征,并能够在不同状态下描述不同的关系。
具体来说,该模型假设时间序列在不同的时间段内处于不同的状态(或区域),而状态之间的转换满足马尔可夫链的性质,即未来状态的转换仅与当前状态有关,与过去状态无关。
二、马尔可夫区制转换向量自回归模型的模型假设马尔可夫区制转换向量自回归模型的主要假设包括以下几点:1. 状态转移性:时间序列的状态转移满足马尔可夫链的性质,未来状态的转移仅与当前状态相关。
2. 向量自回归性:时间序列变量之间的关系可以用向量自回归模型描述,即当前时间点的向量可以由过去时间点的向量线性组合而成。
3. 区制转换性:时间序列的状态在不同时期具有不同的动态特征,模型需要考虑不同状态下的向量自回归关系。
以上假设为马尔可夫区制转换向量自回归模型的基本假设,这些假设使得模型能够较好地描述时间序列数据的动态演化。
三、马尔可夫区制转换向量自回归模型的参数估计方法马尔可夫区制转换向量自回归模型的参数估计是一个重要且复杂的问题,一般可以通过以下几种方法进行估计:1. 极大似然估计:假设时间序列的概率分布形式,通过最大化似然函数来得到模型参数的估计值。
这种方法需要对概率分布进行合理的假设,并且通常需要通过迭代算法来求解。
2. 贝叶斯方法:利用贝叶斯统计理论,结合先验分布和似然函数,通过马尔科夫链蒙特卡洛(MCMC)等方法得到模型参数的后验分布,进而得到参数的估计值。
第一节状态转移图第六章马尔可夫排队模型
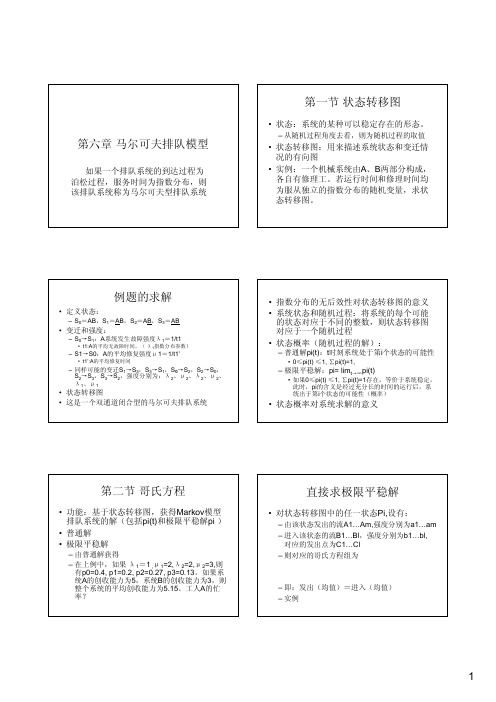
第六章马尔可夫排队模型如果一个排队系统的到达过程为泊松过程,服务时间为指数分布,则该排队系统称为马尔可夫型排队系统第一节状态转移图•状态:系统的某种可以稳定存在的形态。
–从随机过程角度去看,则为随机过程的取值•状态转移图:用来描述系统状态和变迁情况的有向图•实例:一个机械系统由A 、B 两部分构成,各自有修理工。
若运行时间和修理时间均为服从独立的指数分布的随机变量,求状态转移图。
例题的求解•定义状态:–S 0=AB ,S 1=AB ,S 2=AB ,S 3=AB•变迁和强度:–S 0→S 1:A 系统发生故障强度λ1=1/t1•t1:A 的平均无故障时间。
(λ1指数分布参数)–S1→S0:A 的平均修复强度μ1=1/t1’•t1’:A 的平均修复时间–同样可能的变迁S 1→S 3,S 3→S 1,S 0→S 2,S 2→S 0,S 2→S 3,S 3→S 2,强度分别为:λ2、μ2、λ2、μ2、λ1、μ1•状态转移图•这是一个双通道闭合型的马尔可夫排队系统•指数分布的无后效性对状态转移图的意义•系统状态和随机过程:将系统的每个可能的状态对应于不同的整数,则状态转移图对应于一个随机过程•状态概率(随机过程的解):–普通解pi(t):t 时刻系统处于第i 个状态的可能性•0≤pi(t) ≤1, ∑pi(t)=1,–极限平稳解:pi= lim t →∞pi(t)•如果0≤pi(t) ≤1, ∑pi(t)=1存在,等价于系统稳定,此时,pi 的含义是经过充分长的时间的运行后,系统出于第i 个状态的可能性(概率)•状态概率对系统求解的意义第二节哥氏方程•功能:基于状态转移图,获得Markov 模型排队系统的解(包括pi(t)和极限平稳解pi )•普通解•极限平稳解–由普通解获得–在上例中,如果λ1=1,μ1=2,λ2=2,μ2=3,则有p0=0.4, p1=0.2, p2=0.27, p3=0.13。
如果系统A 的创收能力为5,系统B 的创收能力为3,则整个系统的平均创收能力为5.15。
隐马尔可夫模型(有例子-具体易懂)课件
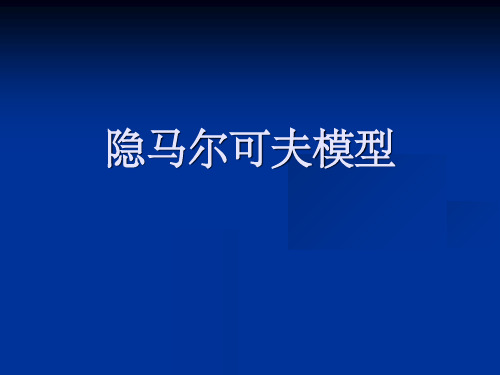
定义前向变量为:
“在时间步t, 得到t之前的所有明符号序列, 且时间 步t的状态是Si”这一事件的概率, 记为 (t, i) = P(o1,…,ot, qt = Si|λ)
则
算法过程
HMM的网格结构
前向算法过程演示
t=1
t=2
t=3
t=4
t=5
t=T
t=6
t=7
问题 1 – 评估问题
给定
一个骰子掷出的点数记录
124552646214614613613666166466163661636616361651561511514612356234
问题
会出现这个点数记录的概率有多大? 求P(O|λ)
问题 2 – 解码问题
给定
一个骰子掷出的点数记录
124552646214614613613666166466163661636616361651561511514612356234
HMM的三个基本问题
令 λ = {π,A,B} 为给定HMM的参数, 令 O = O1,...,OT 为观察值序列,则有关于 隐马尔可夫模型(HMM)的三个基本问题: 1.评估问题: 对于给定模型,求某个观察值序列的概率P(O|λ) ; 2.解码问题: 对于给定模型和观察值序列,求可能性最大的状态序列maxQ{P(Q|O,λ)}; 3.学习问题: 对于给定的一个观察值序列O,调整参数λ,使得观察值出现的概率P(O|λ)最大。
5点
1/6
3/16
6点
1/6
3/8
公平骰子A与灌铅骰子B的区别:
时间
1
2
3
4
5
6
7
骰子
A
A
中天会计事务所马尔可夫模型例题(最完整的例题分析)

中天会计事务所马尔可夫模型例题一、问题分析中天会计事务所由于公司业务日益繁忙,常造成公司事务工作应接不暇,解决该公司出现的这种问题的有效办法是要实施人力资源的供给预测技术。
根据对该公司材料的深入分析,可采用马尔可夫模型这一供给预测方法对该事务所的人力资源状况进行预测。
马尔可夫分析法是一种统计方法,其方法的基本思想是:找出过去人力资源变动的规律,用以来推测未来人力变动的趋势。
马尔可夫分析法适用于外在环境变化不大的情况下,如果外在环境变化较大的时候这种方法则难以用过去的经验情况预测未来。
马尔可夫分析法的分析过程通常是分几个时期来收集数据,然后在得出平均值,利用这些数据代表每一种职位的人员变动频率,就可以推测出人员的变动情况。
二、项目策划(一)第一步是编制人员变动概率矩阵表。
根据公司提供的内部资料:公司的各职位人员如下表1所示。
期到另一个时期(如从某一年到下一年)在两个工作之间调动的雇员数量的历年平均百分比(以小数表示)。
(注:一般以3—5年为周期来估计年平均百分比。
周期越长,根据过去人员变动所推测的未来人员变动就越准确。
)(二)第二步是编制人员变动矩阵表。
将上面的表2做成一个人员变动矩阵表,其具体过程是将中天会计事务所各个阶层员工流动的概率与各职位人数分别相乘即可预测出下一期人员可能调动的情况如下表3所示。
内,而有20%离职。
在任何一年里,平均65%的会计员留在原岗位工作,15%提升为高级会计师,20%离职。
这些历史数据代表了每一种工作中人员变动的概率。
(三)第三步是预测未来的人员变动(供给量)情况。
将计划初期每一种工作的人员数量与每一种工作的人员变动概率相乘,然后纵向相加,即得出表4的组织内部未来劳动力的净供给量。
(四)第四步是该会计事务所的某一期预测如上表4所示。
1、如上表4所示,会计员离职人数最多,离职率也最高,这说明这一职位在将来会出现短缺的现象,据此公司可采取以下具体的对策:①查明公司会计员离职率高的原因,采取必然的措施尽快地降低离职率②加大对公司会计员的培训力度,使他们尽快地晋升为会计师③采取多种方式,广开人员补充的渠道,吸引更多的专业人才补充岗位空缺。
- 1、下载文档前请自行甄别文档内容的完整性,平台不提供额外的编辑、内容补充、找答案等附加服务。
- 2、"仅部分预览"的文档,不可在线预览部分如存在完整性等问题,可反馈申请退款(可完整预览的文档不适用该条件!)。
- 3、如文档侵犯您的权益,请联系客服反馈,我们会尽快为您处理(人工客服工作时间:9:00-18:30)。
The R User Conference 2009 July 8-10, Agrocampus-Ouest, Rennes, FranceEstimating Markovian Switching Regression Models in An application to model energy price in SpainS. Fontdecaba, M. P. Muñoz , J. A. Sànchez*Department of Statistics and Operations Research Universitat Politècnica de Catalunya - UPC* josep.a.sanchez@Markovian Switching Models. An application to model energy price in Spain1 Introduction & Objectives 2 Methodology 3 Data 4 Results 5 ConclusionsOutline1. Introduction & Objectives 2. Methodology 3. Application to energy price 4. Results 5. Conclusions2Markovian Switching Models. An application to model energy price in Spain1 Introduction & Objectives 2 Methodology 3 Data 4 Results 5 Conclusions1. IntroductionThe model we consider is of the MARKOVIAN SWITCHING (MS) type, originally defined by Hamilton (1989).•MSVAR library - Krolszing (1998) (not available free acces: OX) •MSVARlib - Bellone (2005) (Less user friendly) •MSRegression - Perlin (2007) (Libraries in Matlab)3Markovian Switching Models. An application to model energy price in Spain1 Introduction & Objectives 2 Methodology 3 Data 4 Results 5 Conclusions1. Objectives1. Built a set of functions to explain time series according to a Markovian Switching Regression model. 2. Resolution of the problems during the estimation of the Markovian Switching models. 3. Application of Markovian Switching models in energy price in Spain according to the demand, raw material prices and financial indicators.4Markovian Switching Models. An application to model energy price in Spain1 Introduction & Objectives 2 Methodology 3 Data 4 Results 5 Conclusions2. Markovian SwitchingS1 S2 (…) SK(…)tS1 S2SK(…)MARKOVIANP(St=i | St-1=j, St-2=k,…,S1=k)= P(St=i | St-1=j)SWITCHINGt=1 t=2 t=4 t=6 t=5t=3Markovian Switching Models. An application to model energy price in Spain1 Introduction & Objectives 2 Methodology 3 Data 4 Results 5 Conclusions2. Markovian Switchingp12 p11 p22S1Price=f1(X1, X2, X3, X4,…, Xk)p21S2Price=f2(X1, X2, X3, X4,…, Xk)S1 S26tMarkovian Switching Models. An application to model energy price in Spain1 Introduction & Objectives 2 Methodology 3 Data 4 Results 5 Conclusions2. Markovian SwitchingS : Number of statesVARIABLES WITH SWITCHING EFFECTVARIABLES WITHOUT SWITCHING EFFECTParameters of the model to estimate:Deviations of the states: Coefficients of the regression with Switching effect : Coefficients of the regression without Switching effect : Transition probabilities:7Markovian Switching Models. An application to model energy price in Spain1 Introduction & Objectives 2 Methodology 3 Data 4 Results 5 ConclusionsINPUT (user) Dependent variable (Pt) Independent variables (X’s) Number of states (S) Switching Indicator OUTPUT - Evolution of State assignation with probabilities Parameters Estimation (θ) NumericFor each time instant… Perlin (2005)MODEL FITTINGLMGraphic-State Assignation Probability assignment in each state8Markovian Switching Models. An application to model energy price in Spain1 Introduction & Objectives 2 Methodology 3 Data 4 Results 5 ConclusionsModel parameters:Θ = (β ,σ , Π)Model Likelihood:L(Θ; y1:T , X 1:T ) = f ( y1:T | X 1:T , β , σ ) =T∑∑t =1Sf ( yt | S t , X t , β , σ ) P ( S t | Π )The state S is a non-observable latent variable Likelihood = marginal of the conjoint density for y and S9Markovian Switching Models. An application to model energy price in Spain1 Introduction & Objectives 2 Methodology 3 Data 4 Results 5 ConclusionsIn this case, the functional dependence between y and X corresponds to a linear model (OLS)y | X , β , σ ~ N ( Xβ , σ I )2Conditioning on the state S means a different set of parameters for each state.y | S , X , β , σ ~ N ( Xβ(S ),σ(S )2I)Other set-up can be considered: - Extending predictors Autoregressive models - Modifying response distribution Generalized LM - More complex functional dependence Non-linear models10Markovian Switching Models. An application to model energy price in Spain1 Introduction & Objectives 2 Methodology 3 Data 4 Results 5 Conclusions3. Application – Energy priceElectricity markets are characterized by: inelasticity of the demand impossibility of storage Seasonality character: fluctuations of demand due to weather conditions and human habits In the last decade, the issue of modeling and forecasting prices had been the key question to: determine the causes of price behavior Macroeconomic significance of the prices of raw materials. Spain is an importer country11Markovian Switching Models. An application to model energy price in Spain1 Introduction & Objectives 2 Methodology 3 Data 4 Results 5 Conclusions3. Application – Energy priceThe objective of the application is to identify the influence on the energy price of: the demand the price of the raw materials financial information of the markets during different states of its evolution.Development of an R Code to estimate MSM12Markovian Switching Models. An application to model energy price in Spain1 Introduction & Objectives 2 Methodology 3 Data 4 Results 5 Conclusions3. DataENERGYData from January 1, 2002 to October 31, 2008 (daily data– working days: Monday to Friday)RAW MATERIALS • Oil Price(€/barril)FINANCIAL • Exchange Rate between Dolar Euro(USD-Euro)• Average price of energy(Cent/Kw.h)• Gas Price(€/MW.h)• Daily demand of energy(GWh)• Coal Price(€/T)• Ibex 35 Index• Price of CO2 Allowances (€/T)Bierbrauer, Truck and Weron (2006) Amano and Norden (1998); Zachmann (2007) 13Markovian Switching Models. An application to model energy price in Spain1 Introduction & Objectives 2 Methodology903 DataOIL4 Results5 ConclusionsUSD-€1.13. DataPRICE10 8 Cent/kw.h 6 4€/Barril506070804020302002200320042005 Anys2006200720082009GAS800.7 20020.80.91.0200320042005 Anys2006200720082009-0.7360IBEX16000Anys€/MWh2402002200320042005 Anys20062007200820090900120COAL8001006000 20028000DEMAND140¡¡ NO!!1000020022003200420052006200720082009120001400020200320042005 Anys2006200720082009€/T800.78GWh700600.792002 2003 2004 2005 Anys 2006 2007 2008 20096005002002200320042005 Anys200620072008200930402025CO2>ts.plot(data)2002 2003 2004 2005 Anys 2006 2007 2008 2009€/T051015Markovian Switching Models. An application to model energy price in Spain1 Introduction & Objectives 2 Methodology 3 Data 4 Results 5 Conclusions4. Estimation ProcedureMAXIMUM LIKELIHOOD ESTIMATION OF THE PARAMETERS Number of parameters: 18 1. Convergence not assured!!How to determine starting values: Considering “No switching” • Same model under both regimes • Probability of change equal to 0.5 Estimate linear model (OLS) with all the observations15Markovian Switching Models. An application to model energy price in Spain1 Introduction & Objectives 2 Methodology 3 Data 4 Results 5 Conclusions4. Resultsβ βConst. βDemand βOil βGas βCoal βUSD/E βIbex35 Estim. -9.046 -0.0090 0.0832 0.0420 -0.00819 6.059 -0.0001 Std.Error 0.54006*** 0.0004*** 0.0037*** 0.0039*** 0.00201 0.3646*** 0.00001***R2=0.57σ=1.06Markovian Switching Models. An application to model energy price in Spain1 Introduction & Objectives 2 Methodology 3 Data 4 Results 5 Conclusions4. Estimation ProcedureMAXIMUM LIKELIHOOD ESTIMATION OF THE PARAMETERS 2. Non-linear optimization (Newton-Raphson) Evaluation of the likelihood of the model Function optim to find MLE 3. Expectation step for St Calculate the Expectation of St under the current estimates of the parameters EM Alg. Assign each observation to one of the states 4. Maximization step for parameters Conditioning on the values for St, obtain new estimates Estimate linear model (OLS) for each state go to step 2 until convergence17Markovian Switching Models. An application to model energy price in Spain1 Introduction & Objectives 2 Methodology 3 Data 4 Results 5 Conclusions4. Numerical ResultsState=1 ------Coefficients: Estimate Std. Error t value Pr(>|t|) (Intercept) -9.575e+00 6.425e-01 -14.901 < 2e-16 *** Demanda 1.115e-02 5.601e-04 19.898 < 2e-16 *** Petroli 8.161e-02 4.924e-03 16.575 < 2e-16 *** Gas 2.192e-02 4.212e-03 5.204 2.44e-07 *** Carbo 1.406e-03 2.097e-03 0.670 0.503 EurDol 6.219e+00 4.189e-01 14.844 < 2e-16 *** Ibex35 -1.850e-04 2.337e-05 -7.918 7.30e-15 *** --Signif. codes: 0 ‘***’ 0.001 ‘**’ 0.01 ‘*’ 0.05 ‘.’ 0.1 ‘ ’ 1 Residual standard error: 0.8421 on 873 degrees of freedom Multiple R-squared: 0.6648, Adjusted R-squared: 0.6625 Residuals: Min 1Q Median -1.90943 -0.35887 -0.01232 State=2 ------Coefficients: Estimate Std. Error t value Pr(>|t|) (Intercept) -7.361e+00 3.751e-01 -19.623 < 2e-16 *** Demanda 5.301e-03 3.155e-04 16.799 < 2e-16 *** Petroli 5.371e-02 2.539e-03 21.151 < 2e-16 *** Gas 2.338e-02 3.148e-03 7.428 2.57e-13 *** Carbo 1.350e-02 1.613e-03 8.371 < 2e-16 *** EurDol 5.106e+00 2.566e-01 19.902 < 2e-16 *** Ibex35 -2.486e-05 9.812e-06 -2.534 0.0115 * --Signif. codes: 0 ‘***’ 0.001 ‘**’ 0.01 ‘*’ 0.05 ‘.’ 0.1 ‘ ’ 1 Residual standard error: 0.5229 on 897 degrees of freedom Multiple R-squared: 0.8105, Adjusted R-squared: 0.8093Model under State 1 Model under State 2 Transition Matrix3Q 0.37846Max 1.53960Transition Matrix ----------------1 2 1 0.9715 0.0487 2 0.0284 0.9512 Likelihood: 1875.747Pooled Residuals: Residual standard error: 0.69659 on 1770 degrees of freedom Multiple R-squared: 0.816691Markovian Switching Models. An application to model energy price in Spain1 Introduction & Objectives 2 Methodology 3 Data 4 Results 5 Conclusions4. Numerical Resultsβ βConst. βDemand βOil βGas βCoal βUSD/E βIbex35 Estim. -9.575 0.0115 0.08161 0.02192 0.00014 6.219 -0.0008 Std.Error 0.6421*** 0.0005*** 0.0049*** 0.0049*** 0.0021 0.418*** 0.00001*** β βConst. βDemand βOil βGas βCoal βUSD/E βIbex35 Estim. -7.3610 0.005301 0.05371 0.0238 -0.01352 5.1062 -0.00002 Std.Error 0.37515*** 0.0003*** 0.00253*** 0.0031*** 0.00162*** 0.256*** 0.00009***σ=0.842R2=0.66σ=0.52R2=0.81Markovian Switching Models. An application to model energy price in Spain1 Introduction & Objectives 2 Methodology 3 Data 4 Results 5 Conclusions4. Graphical Results10 2002 2003 2004 2005 2006 2007 2008 dep 4 2 6 801.0 2002 20035002004 20051000200615002007 20082 - Estat0.00.20.40.60.8050010001500201 Introduction & Objectives 3 Data2 Methodology 4 Results 5 Conclusions214. Graphical Resultsm o d $e [, 1]050010001500-4-202461 Introduction & Objectives 3 Data2 Methodology 5 Conclusions224. Results2 - E s t a t 0500100015000.00.20.40.60.81.02002200320042005200620072008EconomicRecession Rising Oil PricesTsunami Atypical Situation.Weather Cause Gulf War. Iraq War.“The Corralito”Yukos4 Results1 Introduction & Objectives 3 Data2 Methodology 4 Results 235. Conclusions5 Conclusions & FR1.Detection of a model that is not constant over the time2.Implementation of a estimation methodology forMarkovian Switching models (2 states) using3.Relationship between states changes of price andUSD-Euro and Ibex354.State changes in energy price caused by differentmovements in raw materials price (oil and gas ).1 Introduction & Objectives 3 Data2 Methodology 4 Results 5 Conclusions 245. Future Lines1.Reaction time on the market:Introduce some lagsinformation of explanatory variables.2.Autoregressive terms:Introduce previous prices asexplanatory variables.3.Orthogonal Model:Consider the components of PCAand FA as explanatory variables.4.Flexibility of the model:Check the need of switchingeffect. Increase the number of states.5 Conclusions & FRWork on the routines to be a unique function that allows to the user:1 Introduction & Objectives 3 Data2 Methodology 4 Results 5 Conclusions 25ReferencesBierbrauer, M., Trück, S., Weron, R., 2003. “Modeling Electricity Prices with Regime Switching Models ”, Phisica A 336, 39-48.Hamilton, J., 2005. “Regime Swtiching Models ”, La Jolla CA 92093-0508.Goldfeld, S., Quantd, R., 2005. “A Markov model for switching Regression ”,Journal of Econometrics 135, 349-376.Perlin, M., 2007. “Estimation, Simulation and Forecasting of a Markov Switching Regression ”, (General case in Matlab).Zachmann, G., 2006. “A Markov Switching model of the merit order to compare British and German Price formation ”, Discussion paper. German Institute for Economics Research5 Conclusions & FR2 Methodology 4 Results 5 Conclusions 1 Introduction& Objectives3 DataThank youuse ’s!。