智能语音识别机器人——文献翻译
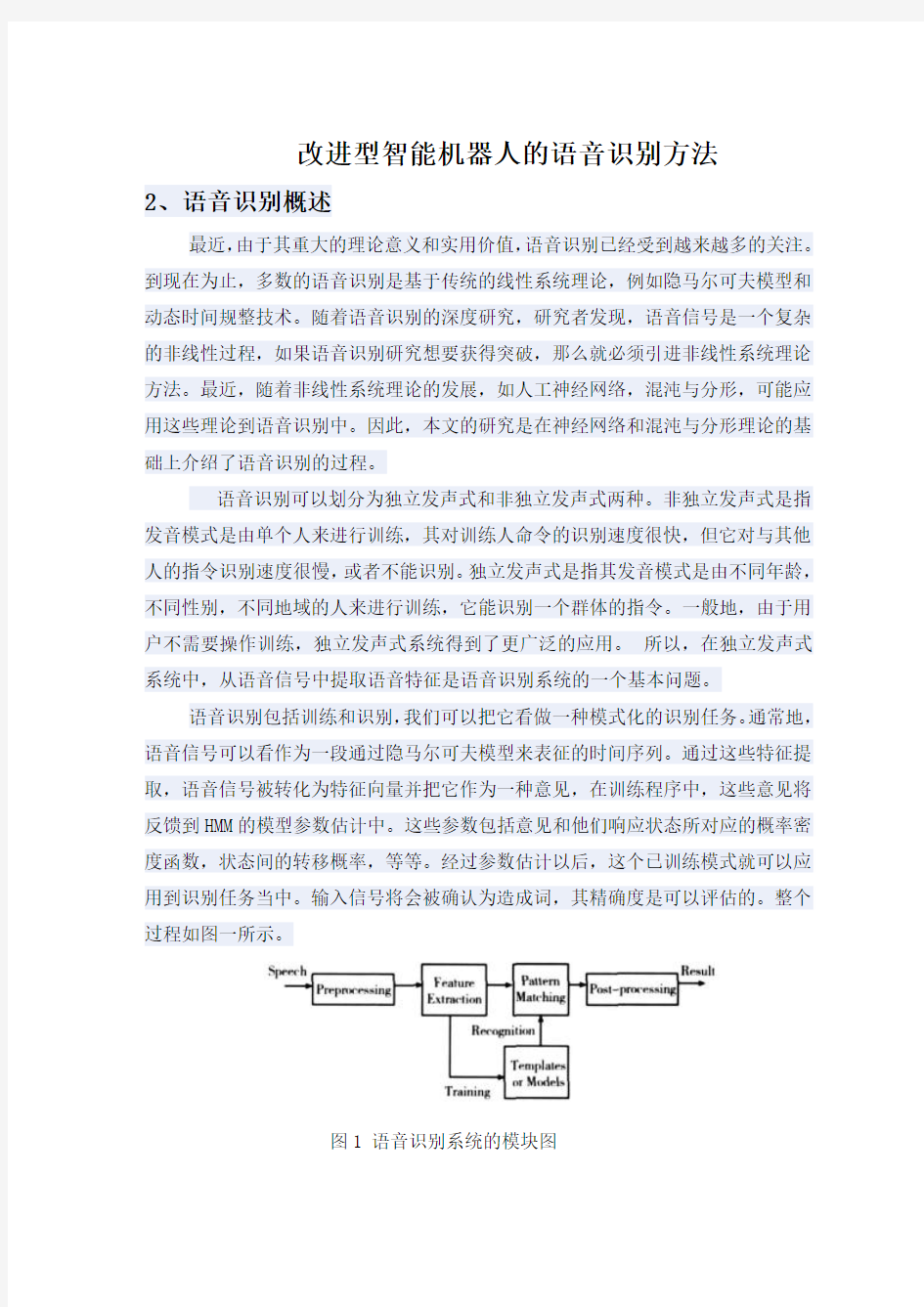

改进型智能机器人的语音识别方法
2、语音识别概述
最近,由于其重大的理论意义和实用价值,语音识别已经受到越来越多的关注。到现在为止,多数的语音识别是基于传统的线性系统理论,例如隐马尔可夫模型和动态时间规整技术。随着语音识别的深度研究,研究者发现,语音信号是一个复杂的非线性过程,如果语音识别研究想要获得突破,那么就必须引进非线性系统理论方法。最近,随着非线性系统理论的发展,如人工神经网络,混沌与分形,可能应用这些理论到语音识别中。因此,本文的研究是在神经网络和混沌与分形理论的基础上介绍了语音识别的过程。
语音识别可以划分为独立发声式和非独立发声式两种。非独立发声式是指发音模式是由单个人来进行训练,其对训练人命令的识别速度很快,但它对与其他人的指令识别速度很慢,或者不能识别。独立发声式是指其发音模式是由不同年龄,不同性别,不同地域的人来进行训练,它能识别一个群体的指令。一般地,由于用户不需要操作训练,独立发声式系统得到了更广泛的应用。所以,在独立发声式系统中,从语音信号中提取语音特征是语音识别系统的一个基本问题。
语音识别包括训练和识别,我们可以把它看做一种模式化的识别任务。通常地,语音信号可以看作为一段通过隐马尔可夫模型来表征的时间序列。通过这些特征提取,语音信号被转化为特征向量并把它作为一种意见,在训练程序中,这些意见将反馈到HMM的模型参数估计中。这些参数包括意见和他们响应状态所对应的概率密度函数,状态间的转移概率,等等。经过参数估计以后,这个已训练模式就可以应用到识别任务当中。输入信号将会被确认为造成词,其精确度是可以评估的。整个过程如图一所示。
图1 语音识别系统的模块图
3、理论与方法
从语音信号中进行独立扬声器的特征提取是语音识别系统中的一个基本问题。解决这个问题的最流行方法是应用线性预测倒谱系数和Mel频率倒谱系数。这两种方法都是基于一种假设的线形程序,该假设认为说话者所拥有的语音特性是由于声道共振造成的。这些信号特征构成了语音信号最基本的光谱结构。然而,在语音信号中,这些非线形信息不容易被当前的特征提取逻辑方法所提取,所以我们使用分型维数来测量非线形语音扰动。
本文利用传统的LPCC和非线性多尺度分形维数特征提取研究并实现语音识别系统。
3.1线性预测倒谱系数
线性预测系数是一个我们在做语音的线形预分析时得到的参数,它是关于毗邻语音样本间特征联系的参数。线形预分析正式基于以下几个概念建立起来的,即一个语音样本可以通过一些以前的样本的线形组合来快速地估计,根据真实语音样本在确切的分析框架(短时间内的)和预测样本之间的差别的最小平方原则,最后会确认出唯一的一组预测系数。
LPC可以用来估计语音信号的倒谱。在语音信号的短时倒谱分析中,这是一种特殊的处理方法。信道模型的系统函数可以通过如下的线形预分析来得到:
其中p代表线形预测命令,,(k=1,2,… …,p)代表预测参数,脉冲响应用
h(n)来表示,假设h(n)的倒谱是。那么(1)式可以扩展为(2)式:
将(1)带入(2),两边同时,(2)变成(3)。
就获得了方程(4):
那么可以通过来获得。
(5)中计算的倒谱系数叫做LPCC,n代表LPCC命令。
在我们采集LPCC参数以前,我们应该对语音信号进行预加重,帧处理,加工和终端窗口检测等,所以,中文命令字“前进”的端点检测如图2所示,接下来,断点检测后的中文命令字“前进”语音波形和LPCC的参数波形如图3所示。
图2 中文命令字“前进”的端点检测
图3 断点检测后的中文命令字“前进”语音波形和LPCC的参数波形
3.2 语音分形维数计算
分形维数是一个与分形的规模与数量相关的定值,也是对自我的结构相似性的测量。分形分维测量是[6-7]。从测量的角度来看,分形维数从整数扩展到了分数,打破了一般集拓扑学方面被整数分形维数的限制,分数大多是在欧几里得几何尺寸的延伸。
有许多关于分形维数的定义,例如相似维度,豪斯多夫维度,信息维度,相关维度,容积维度,计盒维度等等,其中,豪斯多夫维度是最古老同时也是最重要的,它的定义如【3】所示:
其中,表示需要多少个单位来覆盖子集F.
端点检测后,中文命令词“向前”的语音波形和分形维数波形如图4所示。
图4 端点检测后,中文命令词“向前”的语音波形和分形维数波形
3.3 改进的特征提取方法
考虑到LPCC语音信号和分形维数在表达上各自的优点,我们把它们二者混合到信号的特取中,即分形维数表表征语音时间波形图的自相似性,周期性,随机性,同时,LPCC特性在高语音质量和高识别速度上做得很好。
由于人工神经网络的非线性,自适应性,强大的自学能力这些明显的优点,它的优良分类和输入输出响应能力都使它非常适合解决语音识别问题。
由于人工神经网络的输入码的数量是固定的,因此,现在是进行正规化的特征参数输入到前神经网络[9],在我们的实验中,LPCC和每个样本的分形维数需要分别地通过时间规整化的网络,LPCC是一个4帧数据(LPCC1,LPCC2,LPCC3,LPCC4,每个参数都是14维的),分形维数被模范化为12维数据,(FD1,FD2,…FD12,每一个参数都是一维),以便于每个样本的特征向量有4*14+12*1=68-D维,该命令就是前56个维数是LPCC,剩下的12个维数是分形维数。因而,这样的一个特征向量可以表征语音信号的线形和非线性特征。
自动语音识别的结构和特征
自动语音识别是一项尖端技术,它允许一台计算机,甚至是一台手持掌上电脑(迈尔斯,2000)来识别那些需要朗读或者任何录音设备发音的词汇。自动语音识别技术的最终目的是让那些不论词汇量,背景噪音,说话者变音的人直白地说出的单词能够达到100%的准确率(CSLU,2002)。然而,大多数的自动语音识别工程师都承认这样一个现状,即对于一个大的语音词汇单位,当前的准确度水平仍然低于90%。举一个例子,Dragon's Naturally Speaking或者IBM公司,阐述了取决于口音,背景噪音,说话方式的基线识别的准确性仅仅为60%至80%(Ehsani & Knodt, 1998)。更多的能超越以上两个的昂贵的系统有Subarashii (Bernstein, et al., 1999), EduSpeak (Franco, etal., 2001), Phonepass (Hinks, 2001), ISLE Project (Menzel, et al., 2001) and RAD (CSLU, 2003)。语音识别的准确性将有望改善。
在自动语音识别产品中的几种语音识别方式中,隐马尔可夫模型(HMM)被认为是最主要的算法,并且被证明在处理大词汇语音时是最高效的(Ehsani & Knodt, 1998)。详细说明隐马尔可夫模型如何工作超出了本文的范围,但可以在任何关于语言处理的文章中找到。其中最好的是Jurafsky & Martin (2000) and Hosom, Cole, and Fanty (2003)。简而言之,隐马尔可夫模型计算输入接收信号和包含于一个拥有数以百计的本土音素录音的数据库的匹配可能性(Hinks, 2003, p. 5)。也就是说,一台基于隐马尔可夫模型的语音识别器可以计算输入一个发音的音素可以和一个基于概率论相应的模型达到的达到的接近度。高性能就意味着优良的发音,低性能就意味着劣质的发音(Larocca, et al., 1991)。
虽然语音识别已被普遍用于商业听写和获取特殊需要等目的,近年来,语言学习的市场占有率急剧增加(Aist, 1999; Eskenazi, 1999; Hinks, 2003)。早期的基于自动语音识别的软件程序采用基于模板的识别系统,其使用动态规划执行模式匹配或其他时间规范化技术(Dalby & Kewley-Port,1999). 这些程序包括Talk to Me (Auralog, 1995), the Tell Me More Series (Auralog, 2000), Triple-Play Plus (Mackey & Choi, 1998), New Dynamic English (DynEd, 1997), English Discoveries (Edusoft, 1998), and See it, Hear It, SAY IT! (CPI, 1997)。这些程序的大多数都不会提供任何反馈给超出简单说明的发音准确率,这个基于最接近模式匹配说明是由用户提出书面对话选择的。学习者不会被告之他们发音的准
确率。特别是内里,(2002年)评论例如Talk to Me和Tell Me More等作品中的波形图,因为他们期待浮华的买家,而不会提供有意义的反馈给用户。Talk to Me 2002年的版本已经包含了更多Hinks (2003)的特性,比如,信任对于学习者来说是非常有用的:
★一个视觉信号可以让学习者把他们的语调同模型扬声器发出的语调进行对比。★学习者发音的准确度通常以数字7来度量(越高越好)
★那些发音失真的词语会被识别出来并被明显地标注。
Improved speech recognition method
for intelligent robot
2、Overview of speech recognition
Speech recognition has received more and more attention recently due to the important theoretical meaning and practical value [5 ]. Up to now, most speech recognition is based on conventional linear system theory, such as Hidden Markov Model (HMM) and Dynamic Time Warping(DTW) . With the deep study of speech recognition, it is found that speech signal is a complex nonlinear process. If the study of speech recognition wants to break through, nonlinear
-system theory method must be introduced to it. Recently, with the developmentof nonlinea-system theories such as artificial neural networks(ANN) , chaos and fractal, it is possible to apply these theories to speech recognition. Therefore, the study of this paper is based on ANN and chaos and fractal theories are introduced to process speech recognition.
Speech recognition is divided into two ways that are speaker dependent and speaker independent. Speaker dependent refers to the pronunciation model trained by a single person, the identification rate of the training person?sorders is high, while others’orders is in low identification rate or can’t be recognized. Speaker independent refers to the pronunciation model
trained by persons of different age, sex and region, it can identify a group of persons’orders. Generally,speaker independent system ismorewidely used, since the user is not required to conduct the training. So extraction of speaker independent features from the speech signal is the fundamental problem of speaker recognition system.
Speech recognition can be viewed as a pattern recognition task, which includes training and recognition.Generally, speech signal can be viewed as a time sequence and characterized by the powerful hidden Markov model (HMM). Through the feature extraction, the speech signal is transferred into feature vectors and act asobservations. In the training procedure, these observationswill feed to estimate the model parameters of HMM. These parameters include probability density function for the observations and their corresponding states, transition probability between the states, etc. After the parameter estimation, the trained models can be used for recognition task. The input observations will be recognized as the resulted words and the accuracy can be evaluated. Thewhole process is illustrated in Fig. 1.
Fig. 1Block diagram of speech recognition system
3 Theory andmethod
Extraction of speaker independent features from the speech signal is the fundamental problem of speaker recognition system. The standard methodology for solving this problem uses Linear Predictive Cepstral Coefficients (LPCC) and Mel-Frequency Cepstral Co-efficient (MFCC). Both these methods are linear procedures based on the assumption that speaker features have properties caused by the vocal tract resonances. These features form the basic spectral structure of the speech signal. However, the non-linear information in speech signals is not easily extracted by the present feature extraction methodologies. So we use fractal dimension to measure non2linear speech turbulence.
This paper investigates and implements speaker identification system using both traditional LPCC and non-linear multiscaled fractal dimension feature extraction.
3. 1L inear Predictive Cepstral Coefficients
Linear prediction coefficient (LPC) is a parameter setwhich is obtained when we do linear prediction analysis of speech. It is about some correlation characteristics between adjacent speech samples. Linear prediction analysis is based on the following basic concepts. That is, a speech sample can be estimated approximately by the linear combination of some past speech samples. According to the minimal square sum principle of difference between real speech sample in certain analysis frame
short-time and predictive sample, the only group ofprediction coefficients can be determined.
LPC coefficient can be used to estimate speech signal cepstrum. This is a special processing method in analysis of speech signal short-time cepstrum. System function of channelmodel is obtained by linear prediction analysis as follow.
Where p represents linear prediction order, ak,(k=1,2,…,p) represent sprediction coefficient, Impulse response is represented by h(n). Suppose
cepstrum of h(n) is represented by ,then (1) can be expanded as (2).
The cepstrum coefficient calculated in the way of (5) is called LPCC, n represents LPCC order.
When we extract LPCC parameter before, we should carry on speech signal pre-emphasis, framing processing, windowingprocessing and endpoints detection etc. , so the endpoint detection of Chinese command word“Forward”is shown in Fig.2, next, the speech waveform ofChinese command word“Forward”and LPCC parameter waveform after Endpoint detection is shown in Fig. 3.
3. 2 Speech Fractal Dimension Computation
Fractal dimension is a quantitative value from the scale relation on the meaning of fractal, and also a measuring on self-similarity of its structure. The fractal measuring is fractal dimension[6-7]. From the viewpoint of measuring, fractal dimension is extended from integer to fraction, breaking the limitof the general to pology set dimension being integer Fractal dimension,fraction mostly, is dimension extension in Euclidean geometry.
There are many definitions on fractal dimension, eg.,similar dimension, Hausdoff dimension, inforation dimension, correlation dimension, capability imension, box-counting dimension etc. , where,Hausdoff dimension is oldest and also most important, for any sets, it is defined as[3].
Where, M£(F) denotes how many unit £needed to cover subset F.
In thispaper, the Box-Counting dimension (DB) of ,F, is obtained by partitioning the plane with squares grids of side £, and the numberof squares that intersect the plane (N(£)) and is defined as[8].
The speech waveform of Chinese command word“Forward”and fractal dimension waveform after Endpoint detection is shown in Fig. 4. 3. 3Improved feature extractions method
Considering the respective advantages on expressing speech signal of LPCC and fractal dimension,we mix both to be the feature signal, that is, fractal dimension denotes the self2similarity, periodicity and randomness of speech time wave shape, meanwhile LPCC feature is good for speech quality and high on identification rate.
Due to ANN′s nonlinearity, self-adaptability, robust and self-learning such obvious advantages, its good classification and input2output reflection ability are suitable to resolve speech recognition problem.
Due to the number of ANN input nodes being fixed, therefore time regularization is carried out to the feature parameter before inputted to the neural network[9]. In our experiments, LPCC and fractal dimension of each
sample are need to get through the network of time regularization separately, LPCC is 4-frame data(LPCC1,LPCC2,LPCC3,LPCC4, each frame parameter is 14-D), fractal dimension is regularized to be12-frame data(FD1,FD2,…,FD12, each frame parameter is 1-D), so that the feature vector of each sample has 4*14+1*12=68-D, the order is, the first 56 dimensions are LPCC, the rest 12 dimensions are fractal dimensions. Thus, such mixed feature parameter can show speech linear and nonlinear characteristics as well.
Architectures and Features of ASR ASR is a cutting edge technology that allows a computer or even a hand-held PDA (Myers, 2000) to identify words that are read aloud or spoken into any sound-recording device. The ultimate purpose of ASR technology is to allow 100% accuracy with all words that are intelligibly spoken by any person regardless of vocabulary size, background noise, or speaker variables (CSLU, 2002). However, most ASR engineers admit that the current accuracy level for a large vocabulary unit of speech (e.g., the sentence) remains less than 90%. Dragon's Naturally Speaking or IBM's ViaV oice, for example, show a baseline recognition accuracy of only 60% to 80%, depending upon accent, background noise, type of utterance, etc. (Ehsani & Knodt, 1998). More expensive systems that are reported to outperform these two are Subarashii (Bernstein, et al., 1999), EduSpeak (Franco, et al., 2001), Phonepass (Hinks, 2001), ISLE Project (Menzel, et al., 2001) and RAD (CSLU, 2003). ASR accuracy is expected to improve. Among several types of speech recognizers used in ASR products, both implemented and proposed, the Hidden Markov Model (HMM) is one of the most dominant algorithms and has proven to be an effective method of dealing with large units of speech (Ehsani & Knodt, 1998). Detailed descriptions of how the HHM model works go beyond the scope of this paper and can be found in any text concerned with language processing; among the best are Jurafsky & Martin (2000) and Hosom, Cole, and Fanty
(2003). Put simply, HMM computes the probable match between the input it receives and phonemes contained in a database of hundreds of native speaker recordings (Hinks, 2003, p. 5). That is, a speech recognizer based on HMM computes how close the phonemes of a spoken input are to a corresponding model, based on probability theory. High likelihood represents good pronunciation; low likelihood represents poor pronunciation (Larocca, et al., 1991).
While ASR has been commonly used for such purposes as business dictation and special needs accessibility, its market presence for language learning has increased dramatically in recent years (Aist, 1999; Eskenazi, 1999; Hinks, 2003). Early ASR-based software programs adopted template-based recognition systems which perform pattern matching using dynamic programming or other time normalization techniques (Dalby & Kewley-Port, 1999). These programs include Talk to Me (Auralog, 1995), the Tell Me More Series (Auralog, 2000), Triple-Play Plus (Mackey & Choi, 1998), New Dynamic English (DynEd, 1997), English Discoveries (Edusoft, 1998), and See it, Hear It, SAY IT! (CPI, 1997). Most of these programs do not provide any feedback on pronunciation accuracy beyond simply indicating which written dialogue choice the user has made, based on the closest pattern match. Learners are not told the accuracy of their pronunciation. In particular, Neri, et al. (2002) criticizes the graphical wave forms presented in products such as Talk to Me and Tell Me More because
they look flashy to buyers, but do not give meaningful feedback to users. The 2000 version of Talk to Me has incorporated more of the features that Hinks (2003), for example, believes are useful to learners:
★A visual signal allows learners to compare their intonation to that of the model speaker.
★The learners' pronunciation accuracy is scored on a scale of seven (the higher the better).
Words whose pronunciation fails to be recognized are highlighted
智能机器人的语音识别
智能机器人的语音识别 语音识别概述 最近,由于其重大的理论意义和实用价值,语音识别已经受到越来越多的关注。到现在为止,多数的语音识别是基于传统的线性系统理论,例如隐马尔可夫模型和动态时间规整技术。随着语音识别的深度研究,研究者发现,语音信号是一个复杂的非线性过程,如果语音识别研究想要获得突破,那么就必须引进非线性系统理论方法。最近,随着非线性系统理论的发展,如人工神经网络,混沌与分形,可能应用这些理论到语音识别中。因此,本文的研究是在神经网络和混沌与分形理论的基础上介绍了语音识别的过程。 语音识别可以划分为独立发声式和非独立发声式两种。非独立发声式是指发音模式是由单个人来进行训练,其对训练人命令的识别速度很快,但它对与其他人的指令识别速度很慢,或者不能识别。独立发声式是指其发音模式是由不同年龄,不同性别,不同地域的人来进行训练,它能识别一个群体的指令。一般地,由于用户不需要操作训练,独立发声式系统得到了更广泛的应用。所以,在独立发声式系统中,从语音信号中提取语音特征是语音识别系统的一个基本问题。 语音识别包括训练和识别,我们可以把它看做一种模式化的识别任务。通常地,语音信号可以看作为一段通过隐马尔可夫模型来表征的时间序列。通过这些特征提取,语音信号被转化为特征向量并把它作为一种意见,在训练程序中,这些意见将反馈到HMM的模型参数估计中。这些参数包括意见和他们响应状态所对应的概率密度函数,状态间的转移概率,等等。经过参数估计以后,这个已训练模式就可以应用到识别任务当中。输入信号将会被确认为造成词,其精确度是可以评估的。整个过程如图一所示。 图1 语音识别系统的模块图
3、理论与方法 从语音信号中进行独立扬声器的特征提取是语音识别系统中的一个基本问题。解决这个问题的最流行方法是应用线性预测倒谱系数和Mel频率倒谱系数。这两种方法都是基于一种假设的线形程序,该假设认为说话者所拥有的语音特性是由于声道共振造成的。这些信号特征构成了语音信号最基本的光谱结构。然而,在语音信号中,这些非线形信息不容易被当前的特征提取逻辑方法所提取,所以我们使用分型维数来测量非线形语音扰动。 本文利用传统的LPCC和非线性多尺度分形维数特征提取研究并实现语音识别系统。 3.1线性预测倒谱系数 线性预测系数是一个我们在做语音的线形预分析时得到的参数,它是关于毗邻语音样本间特征联系的参数。线形预分析正式基于以下几个概念建立起来的,即一个语音样本可以通过一些以前的样本的线形组合来快速地估计,根据真实语音样本在确切的分析框架(短时间内的)和预测样本之间的差别的最小平方原则,最后会确认出唯一的一组预测系数。 LPC可以用来估计语音信号的倒谱。在语音信号的短时倒谱分析中,这是一种特殊的处理方法。信道模型的系统函数可以通过如下的线形预分析来得到: 其中p代表线形预测命令,,(k=1,2,… …,p)代表预测参数,脉冲响应用 h(n)来表示,假设h(n)的倒谱是。那么(1)式可以扩展为(2)式: 将(1)带入(2),两边同时,(2)变成(3)。 就获得了方程(4):
人形机器人论文中英文资料对照外文翻译
中英文资料对照外文翻译 最小化传感级别不确定性联合策略的机械手控制 摘要:人形机器人的应用应该要求机器人的行为和举止表现得象人。下面的决定和控制自己在很大程度上的不确定性并存在于获取信息感觉器官的非结构化动态环境中的软件计算方法人一样能想得到。在机器人领域,关键问题之一是在感官数据中提取有用的知识,然后对信息以及感觉的不确定性划分为各个层次。本文提出了一种基于广义融合杂交分类(人工神经网络的力量,论坛渔业局)已制定和申请验证的生成合成数据观测模型,以及从实际硬件机器人。选择这个融合,主要的目标是根据内部(联合传感器)和外部( Vision 摄像头)感觉信息最大限度地减少不确定性机器人操纵的任务。目前已被广泛有效的一种方法论就是研究专门配置5个自由度的实验室机器人和模型模拟视觉控制的机械手。在最近调查的主要不确定性的处理方法包括加权参数选择(几何融合),并指出经过训练在标准操纵机器人控制器的设计的神经网络是无法使用的。这些方法在混合配置,大大减少了更快和更精确不同级别的机械手控制的不确定性,这中方法已经通过了严格的模拟仿真和试验。 关键词:传感器融合,频分双工,游离脂肪酸,人工神经网络,软计算,机械手,可重复性,准确性,协方差矩阵,不确定性,不确定性椭球。 1 引言 各种各样的机器人的应用(工业,军事,科学,医药,社会福利,家庭和娱乐)已涌现了越来越多产品,它们操作范围大并呢那个在非结构化环境中运行 [ 3,12,15]。在大多数情况下,如何认识环境正在发生变化且每个瞬间最优控制机器人的动作是至关重要的。移动机器人也基本上都有定位和操作非常大的非结构化的动态环境和处理重大的不确定性的能力[ 1,9,19 ]。每当机器人操作在随意性自然环境时,在给定的工作将做完的条件下总是存在着某种程
机器人外文翻译
英文原文出自《Advanced Technology Libraries》2008年第5期 Robot Robot is a type of mechantronics equipment which synthesizes the last research achievement of engine and precision engine, micro-electronics and computer, automation control and drive, sensor and message dispose and artificial intelligence and so on. With the development of economic and the demand for automation control, robot technology is developed quickly and all types of the robots products are come into being. The practicality use of robot products not only solves the problems which are difficult to operate for human being, but also advances the industrial automation program. At present, the research and development of robot involves several kinds of technology and the robot system configuration is so complex that the cost at large is high which to a certain extent limit the robot abroad use. To development economic practicality and high reliability robot system will be value to robot social application and economy development. With the rapid progress with the control economy and expanding of the modern cities, the let of sewage is increasing quickly: With the development of modern technology and the enhancement of consciousness about environment reserve, more and more people realized the importance and urgent of sewage disposal. Active bacteria method is an effective technique for sewage disposal,The lacunaris plastic is an effective basement for active bacteria adhesion for sewage disposal. The abundance requirement for lacunaris plastic makes it is a consequent for the plastic producing with automation and high productivity. Therefore, it is very necessary to design a manipulator that can automatically fulfill the plastic holding. With the analysis of the problems in the design of the plastic holding manipulator and synthesizing the robot research and development condition in recent years, a economic scheme is concluded on the basis of the analysis of mechanical configuration, transform system, drive device and control system and guided by the idea of the characteristic and complex of mechanical configuration,
机械设计设计外文文献翻译、中英文翻译、外文翻译
机械设计 摘要:机器是由机械装置和其它组件组成的。它是一种用来转换或传递能量的装置,例如:发动机、涡轮机、车辆、起重机、印刷机、洗衣机、照相机和摄影机等。许多原则和设计方法不但适用于机器的设计,也适用于非机器的设计。术语中的“机械装置设计”的含义要比“机械设计”的含义更为广泛一些,机械装置设计包括机械设计。在分析运动及设计结构时,要把产品外型以及以后的保养也要考虑在机械设计中。在机械工程领域中,以及其它工程领域中,所有这些都需要机械设备,比如:开关、凸轮、阀门、船舶以及搅拌机等。 关键词:设计流程设计规则机械设计 设计流程 设计开始之前就要想到机器的实际性,现存的机器需要在耐用性、效率、重量、速度,或者成本上得到改善。新的机器必需具有以前机器所能执行的功能。 在设计的初始阶段,应该允许设计人员充分发挥创造性,不要受到任何约束。即使产生了许多不切实际的想法,也会在设计的早期,即在绘制图纸之前被改正掉。只有这样,才不致于阻断创新的思路。通常,还要提出几套设计方案,然后加以比较。很有可能在这个计划最后决定中,使用了某些不在计划之内的一些设想。 一般的当外型特点和组件部分的尺寸特点分析得透彻时,就可以全面的设计和分析。接着还要客观的分析机器性能的优越性,以及它的安全、重量、耐用性,并且竞争力的成本也要考虑在分析结果之内。每一个至关重要的部分要优化它的比例和尺寸,同时也要保持与其它组成部分相协调。 也要选择原材料和处理原材料的方法。通过力学原理来分析和实现这些重要的特性,如那些静态反应的能量和摩擦力的最佳利用,像动力惯性、加速动力和能量;包括弹性材料的强度、应力和刚度等材料的物理特性,以及流体润滑和驱动器的流体力学。设计的过程是重复和合作的过程,无论是正式或非正式的进行,对设计者来说每个阶段都很重要。 最后,以图样为设计的标准,并建立将来的模型。如果它的测试是符合事先要
机器人结构论文中英文对照资料外文翻译文献
中英文对照资料外文翻译文献 FEM Optimization for Robot Structure Abstract In optimal design for robot structures, design models need to he modified and computed repeatedly. Because modifying usually can not automatically be run, it consumes a lot of time. This paper gives a method that uses APDL language of ANSYS 5.5 software to generate an optimal control program, which mike optimal procedure run automatically and optimal efficiency be improved. 1)Introduction Industrial robot is a kind of machine, which is controlled by computers. Because efficiency and maneuverability are higher than traditional machines, industrial robot is used extensively in industry. For the sake of efficiency and maneuverability, reducing mass and increasing stiffness is more important than traditional machines, in structure design of industrial robot. A lot of methods are used in optimization design of structure. Finite element method is a much effective method. In general, modeling and modifying are manual, which is feasible when model is simple. When model is complicated, optimization time is longer. In the longer optimization time, calculation time is usually very little, a majority of time is used for modeling and modifying. It is key of improving efficiency of structure optimization how to reduce modeling and modifying time. APDL language is an interactive development tool, which is based on ANSYS and is offered to program users. APDL language has typical function of some large computer languages. For example, parameter definition similar to constant and variable definition, branch and loop control, and macro call similar to function and subroutine call, etc. Besides these, it possesses powerful capability of mathematical calculation. The capability of mathematical calculation includes arithmetic calculation, comparison, rounding, and trigonometric function, exponential function and hyperbola function of standard FORTRAN language, etc. By means of APDL language, the data can be read and then calculated, which is in database of ANSYS program, and running process of ANSYS program can be controlled.
机械专业外文翻译(中英文翻译)
外文翻译 英文原文 Belt Conveying Systems Development of driving system Among the methods of material conveying employed,belt conveyors play a very important part in the reliable carrying of material over long distances at competitive cost.Conveyor systems have become larger and more complex and drive systems have also been going through a process of evolution and will continue to do so.Nowadays,bigger belts require more power and have brought the need for larger individual drives as well as multiple drives such as 3 drives of 750 kW for one belt(this is the case for the conveyor drives in Chengzhuang Mine).The ability to control drive acceleration torque is critical to belt conveyors’performance.An efficient drive system should be able to provide smooth,soft starts while maintaining belt tensions within the specified safe limits.For load sharing on multiple drives.torque and speed control are also important considerations in the drive system’s design. Due to the advances in conveyor drive control technology,at present many more reliable.Cost-effective and performance-driven conveyor drive systems covering a wide range of power are available for customers’ choices[1]. 1 Analysis on conveyor drive technologies 1.1 Direct drives Full-voltage starters.With a full-voltage starter design,the conveyor head shaft is direct-coupled to the motor through the gear drive.Direct full-voltage starters are adequate for relatively low-power, simple-profile conveyors.With direct fu11-voltage starters.no control is provided for various conveyor loads and.depending on the ratio between fu11-and no-1oad power requirements,empty starting times can be three or four times faster than full load.The maintenance-free starting system is simple,low-cost and very reliable.However, they cannot control starting torque and maximum stall torque;therefore.they are
人工智能论文 语音识别
信息学院 《人工智能及其应用》课程论文题目:基于神经网络的语音信号识别 作者黄超班级自动08-1BF班 系别信息学院专业自动化 完成时间 2011.6.12
基于神经网络的语音信号识别 摘要 语言是人类之间交流信息的主要手段之一,自电脑发明以来,人们就一直致力于使电 脑能够理解自然语言。语音识别技术是集声学、语音学、语言学、计算机、信息处理和人工 智能等诸领域的一项综合技术,应用需求十分广阔,长期以来一直是人们研究的热点。 神经网络是在现代科学研究成果的基础上提出来的模拟人脑结构机制的一门新兴科 学,它模拟了人类神经元活动的原理,具有自学习、联想、对比、推理和概括能力,为很好 地解决语音识别这样一个复杂的模式分类问题提供了新的途径。 本文针时语音识别的特点.对BP神经网络在语音识别技术中的应用进行了探索性研究, 进而结合人工智能领域较为有效的方法——遗传(GA)算法。针对传统BP算法识别准确率高 但训练速度慢的缺点,对BP网络进行改进,构建了一种基于遗传神经网络的语音识别算法(GABP),并建立相应的语音识别系统。仿真实验表明,该算法有效地缩短了识别时问,提 高了网络训练速度和语音的识别率。 关键词:语音识别,神经网络,遗传算法,遗传神经网络,BP网络 THE RSREARCH OF SPEECH RECOGNITION BASED ON THE NEURAL NETWORK ABSTRACT Language is one of the most important means of exchanging information among the mankind.Since the computer was invented,many scientists have been devoted to enabling the computer to understand the natural language.Speech recognition is a comprehensive technology of such areas as acoustics,phonetics,linguistics,computer science,information processing and artificial intelligence,which can be used widely.The research of speech recognition technology has been focused by the world for a long time.The neural network is a new developing science,which simulates the mechanism of human brain and was putted forward by the developing of modern science.It is not the overall description of human brain,but the abstract,simulation and simplifying of the physical neural networks of human beings. The purpose of the research in this area is exploring the human brain mechanisms in information processing,storing and searching.If people can understand these mechanisms,a new way for the research of artificial intelligence,information processing and etc.
工业机器人外文翻译
附录外文文献 原文 Industrial Robots Definition “A robot is a reprogrammable,multifunctional machine designed to manipulate materials,parts,tools,or specialized devices,through variable programmed motions for the performance of a variety of tasks.” --Robotics Industries Association “A robot is an automatic device that performs functions normally ascribrd to humans or a machine in orm of a human.” --Websters Dictionary The industrial robot is used in the manufacturing environment to increase productivity . It can be used to do routine and tedious assembly line jobs , or it can perform jobs that might be hazardous to do routine and tedious assembly line jobs , or it can perform jobs that might be hazardous to the human worker . For example , one of the first industrial robots was used to replace the nuclear fuel rods in nuclear power plants . A human doing this job might be exposed to harmful amounts of radiation . The industrial robot can also operate on the assembly line , putting together small components , such as placing electronic components on a printed circuit board . Thus , the human worker can be relieved of the routine operation of this tedious task . Robots can also be programmed to defuse bombs , to serve the handicapped , and to perform functions in numerous applications in our society . The robot can be thought of as a machine that will move an end-of-arm tool , sensor , and gripper to a preprogrammed location . When the robot arrives at this location , it will perform some sort of task . This task could be welding , sealing , machine loading , machine unloading , or a host of assembly jobs . Generally , this work can be accomplished without the involvement of a human being , except for programming and for turning the system on and off . The basic terminology of robotic systems is introduced in the following :
智能避障机器人设计外文翻译
INTELLIGENT VEHICLE Our society is awash in “machine intelligence” of various kinds.Over the last century, we have witnessed more and more of the “drudgery” of daily living being replaced by devices such as washing machines. One remaining area of both drudgery and danger, however, is the daily act ofdriving automobiles 1.2 million people were killed in traffic crashes in 2002, which was 2.1% of all globaldeaths and the 11th ranked cause of death . If this trend continues, an estimated 8.5 million people will be dying every year in road crashes by 2020. In fact, the U.S. Department of Transportation has estimated the overall societal cost of road crashes annually in the United States at greater than $230 billion. When hundreds or thousands of vehicles are sharing the same roads at the same time, leading to the all too familiar experience of congested traffic. Traffic congestion undermines our quality of life in the same way air pollution undermines public health.Around 1990, road transportation professionals began to apply them to traffic and road management. Thus was born the intelligent transportation system(ITS). Starting in the late 1990s, ITS systems were developed and deployed. In developed countries, travelers today have access to signifi-cant amounts of information about travel conditions, whether they are driving their own vehicle or riding on public transit systems. As the world energy crisis, and the war and the energy
发动机类外文文献翻译(中文)
. 轻型发动机设计方案 摘要: 在过去的一些年里,新一代汽车发动机燃油效率并不是像我们预料中的那样会有所降低。其原因:汽车重量的增加。通过对汽车发动机整车重量以及部分重量的分析知:曲轴箱作为一个单一部件具有潜在的可减少重量的部件,这篇论文讲述的是通过利用轻型材料和现代的设计手段减少发动机重量的方法。 将轻型材料应用于曲轴箱设计构思中包含着广泛的设计理念,这种设计理念就是尽最大可能利用被选材料所具有的可能性去减少汽车重量,以下我将详细的谈论关于直列式和V-型发动机特殊方法的构思,发动机重量减轻也可以利用中小型发动机来代替又大又重的发动机,现代技术以被应用于现存的发动机设计构思中从而增加发动机功率重量比,使发动机性能得到提高因此它的市场价值也得到提高。 新型轻型发动机设计方案中有一个重要方面就是与传统发动机设计理念相比要尽量减小发动机零部件数量,因为这样对于减少整车重量有着非常重要作用。 介绍: 汽车在生态方面和将来继续充当普通交通工具的要求已经显著提高尤其在美国和欧洲。通过合法的要求使那些有压力的顾客在这方面得到缓解。必需考虑到这样的事实,对于燃油的消耗,排放,回收在利用这些中心问题要有一个回应。 在过去的一些年里,汽车发动机的发展取的了进步,使发动机功率得到了显著的提高同时在降低发动机燃油消耗和排放方面已经付出巨大的努力。通过应用直喷,废气涡轮增压和多气门技术于柴油发动机中,使发动机的性能得到显著提高。 新车取代与在它之前所有具有相类似功能车时,其新车发动机工作效率的提高并不是通过对原有车的有效改进。整个交通工具工作效率停滞不前甚至降低的原因是在过去的十五年里增加了15%~20%的车辆(图1)。尽管轻型材料的使用不断增长以及设计者有意识的向轻型结构方面设计但是重量减轻却被其他方面所弥补。读者可以通过以下方面得知:多余的汽车外形 安全方面的改进
人工智能专业外文翻译-机器人
译文资料: 机器人 首先我介绍一下机器人产生的背景,机器人技术的发展,它应该说是一个科学技术发展共同的一个综合性的结果,同时,为社会经济发展产生了一个重大影响的一门科学技术,它的发展归功于在第二次世界大战中各国加强了经济的投入,就加强了本国的经济的发展。另一方面它也是生产力发展的需求的必然结果,也是人类自身发展的必然结果,那么随着人类的发展,人们在不断探讨自然过程中,在认识和改造自然过程中,需要能够解放人的一种奴隶。那么这种奴隶就是代替人们去能够从事复杂和繁重的体力劳动,实现人们对不可达世界的认识和改造,这也是人们在科技发展过程中的一个客观需要。 机器人有三个发展阶段,那么也就是说,我们习惯于把机器人分成三类,一种是第一代机器人,那么也叫示教再现型机器人,它是通过一个计算机,来控制一个多自由度的一个机械,通过示教存储程序和信息,工作时把信息读取出来,然后发出指令,这样的话机器人可以重复的根据人当时示教的结果,再现出这种动作,比方说汽车的点焊机器人,它只要把这个点焊的过程示教完以后,它总是重复这样一种工作,它对于外界的环境没有感知,这个力操作力的大小,这个工件存在不存在,焊的好与坏,它并不知道,那么实际上这种从第一代机器人,也就存在它这种缺陷,因此,在20世纪70年代后期,人们开始研究第二代机器人,叫带感觉的机器人,这种带感觉的机器人是类似人在某种功能的感觉,比如说力觉、触觉、滑觉、视觉、听觉和人进行相类比,有了各种各样的感觉,比方说在机器人抓一个物体的时候,它实际上力的大小能感觉出来,它能够通过视觉,能够去感受和识别它的形状、大小、颜色。抓一个鸡蛋,它能通过一个触觉,知道它的力的大小和滑动的情况。第三代机器人,也是我们机器人学中一个理想的所追求的最高级的阶段,叫智能机器人,那么只要告诉它做什么,不用告诉它怎么去做,它就能完成运动,感知思维和人机通讯的这种功能和机能,那么这个目前的发展还是相对的只是在局部有这种智能的概念和含义,但真正完整意义的这种智能机器人实际上并没有存在,而只是随着我们不断的科学技术的发展,智能的概念越来越丰富,它内涵越来越宽。 下面我简单介绍一下我国机器人发展的基本概况。由于我们国家存在很多其
Manufacturing Engineering and Technology(机械类英文文献+翻译)
Manufacturing Engineering and Technology—Machining Serope kalpakjian;Steven R.Schmid 机械工业出版社2004年3月第1版 20.9 MACHINABILITY The machinability of a material usually defined in terms of four factors: 1、Surface finish and integrity of the machined part; 2、Tool life obtained; 3、Force and power requirements; 4、Chip control. Thus, good machinability good surface finish and integrity, long tool life, and low force And power requirements. As for chip control, long and thin (stringy) cured chips, if not broken up, can severely interfere with the cutting operation by becoming entangled in the cutting zone. Because of the complex nature of cutting operations, it is difficult to establish relationships that quantitatively define the machinability of a material. In manufacturing plants, tool life and surface roughness are generally considered to be the most important factors in machinability. Although not used much any more, approximate machinability ratings are available in the example below. 20.9.1 Machinability Of Steels Because steels are among the most important engineering materials (as noted in Chapter 5), their machinability has been studied extensively. The machinability of steels has been mainly improved by adding lead and sulfur to obtain so-called free-machining steels. Resulfurized and Rephosphorized steels. Sulfur in steels forms manganese sulfide inclusions (second-phase particles), which act as stress raisers in the primary shear zone. As a result, the chips produced break up easily and are small; this improves machinability. The size, shape, distribution, and concentration of these inclusions significantly influence machinability. Elements such as tellurium and selenium, which are both chemically similar to sulfur, act as inclusion modifiers in
搬运机器人外文翻译
外文翻译 专业机械电子工程 学生姓名张华 班级 B机电092 学号 05 指导教师袁健
外文资料名称:Research,design and experiment of end effector for wafer transfer robot 外文资料出处:Industrail Robot:An International Journal 附件: 1.外文资料翻译译文 2.外文原文
晶片传送机器人末端效应器研究、设计和实验 刘延杰、徐梦、曹玉梅 张华译 摘要:目的——晶片传送机器人扮演一个重要角色IC制造行业并且末端执行器是一个重要的组成部分的机器人。本文的目的是使晶片传送机器人通过研究其末端执行器提高传输效率,同时减少晶片变形。 设计/方法/方法——有限元方法分析了晶片变形。对于在真空晶片传送机器人工作,首先,作者运用来自壁虎的超细纤维阵列的设计灵感研究机器人的末端执行器,和现在之间方程机器人的交通加速度和参数的超细纤维数组。基于这些研究,一种微阵列凹凸设计和应用到一个结构优化的末端执行器。对于晶片传送机器人工作在大气环境中,作者分析了不同因素的影响晶片变形。在吸收面积的压力分布的计算公式,提出了最大传输加速度。最后, 根据这些研究得到了一个新的种末端执行器设计大气机器人。 结果——实验结果表明, 通过本文研究应用晶片传送机器人的转换效率已经得到显着提高。并且晶片变形吸收力得到控制。 实际意义——通过实验可以看出,通过本文的研究,可以用来提高机器人传输能力, 在生产环境中减少晶片变形。还为进一步改进和研究末端执行器打下坚实的基础,。 创意/价值——这是第一次应用研究由壁虎启发了的超细纤维阵列真空晶片传送机器人。本文还通过有限元方法仔细分析不同因素在晶片变形的影响。关键词:晶片传送机器人末端执行器、超细纤维数组、晶片 1.介绍