生存分析
生存状况的统计分析方法
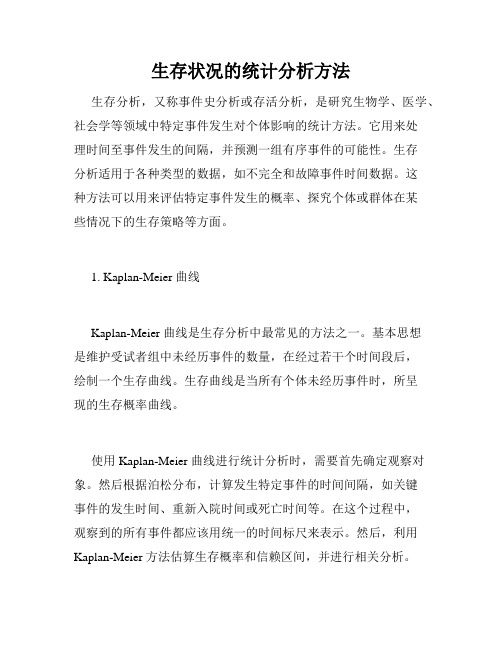
生存状况的统计分析方法生存分析,又称事件史分析或存活分析,是研究生物学、医学、社会学等领域中特定事件发生对个体影响的统计方法。
它用来处理时间至事件发生的间隔,并预测一组有序事件的可能性。
生存分析适用于各种类型的数据,如不完全和故障事件时间数据。
这种方法可以用来评估特定事件发生的概率、探究个体或群体在某些情况下的生存策略等方面。
1. Kaplan-Meier 曲线Kaplan-Meier 曲线是生存分析中最常见的方法之一。
基本思想是维护受试者组中未经历事件的数量,在经过若干个时间段后,绘制一个生存曲线。
生存曲线是当所有个体未经历事件时,所呈现的生存概率曲线。
使用 Kaplan-Meier 曲线进行统计分析时,需要首先确定观察对象。
然后根据泊松分布,计算发生特定事件的时间间隔,如关键事件的发生时间、重新入院时间或死亡时间等。
在这个过程中,观察到的所有事件都应该用统一的时间标尺来表示。
然后,利用Kaplan-Meier 方法估算生存概率和信赖区间,并进行相关分析。
2. Cox 比例风险模型Cox 比例风险模型是另一种常见的生存分析方法。
Cox 比例风险模型用于研究哪些因素与事件的发生有关,例如:在研究医疗发展的过程中,是否采用了更好的医疗技术、是否使用了更好的药物等。
比例风险集中于影响时间至事件对象出现的概率,模型的一般形式如下:$ Hazard = h(t) = h_0(t) * e^{X_ β} $其中,h(t) 是在时刻 t 处的危险率;h0(t) 是在时刻 t 处的基础危险率;X 代表解释变量向量。
(例如,发病风险、月经周期等)当 Cox 比例风险模型应用于生存数据时,观察对象通常是人群、社区、患者队列等等。
3. 计算生存指数计算生存指数是研究特定问题时应用的一种方法。
计算生存指数可以帮助你理解分析结果,并向其他人阐释研究发现。
生存指数用于表示某一集团受实验干扰的影响效应。
一般,生存指数是指在实验和对照组中,观察到的某个时间段内的患病率的比值。
临床研究中的生存分析与生命表计算
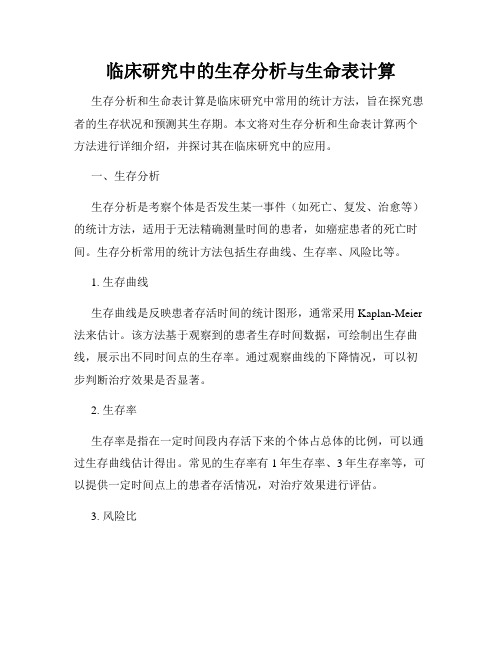
临床研究中的生存分析与生命表计算生存分析和生命表计算是临床研究中常用的统计方法,旨在探究患者的生存状况和预测其生存期。
本文将对生存分析和生命表计算两个方法进行详细介绍,并探讨其在临床研究中的应用。
一、生存分析生存分析是考察个体是否发生某一事件(如死亡、复发、治愈等)的统计方法,适用于无法精确测量时间的患者,如癌症患者的死亡时间。
生存分析常用的统计方法包括生存曲线、生存率、风险比等。
1. 生存曲线生存曲线是反映患者存活时间的统计图形,通常采用Kaplan-Meier 法来估计。
该方法基于观察到的患者生存时间数据,可绘制出生存曲线,展示出不同时间点的生存率。
通过观察曲线的下降情况,可以初步判断治疗效果是否显著。
2. 生存率生存率是指在一定时间段内存活下来的个体占总体的比例,可以通过生存曲线估计得出。
常见的生存率有1年生存率、3年生存率等,可以提供一定时间点上的患者存活情况,对治疗效果进行评估。
3. 风险比风险比是比较两组或多组患者生存时间的指标,用来评估不同治疗方法的效果。
通常采用Cox回归模型来计算,得出的风险比越大,说明在某一组患者中发生事件的风险越高,治疗效果越差。
二、生命表计算生命表计算是用来评估某一特定人群的生存概率和预测其实际寿命的方法。
生命表常用于人口学研究和流行病学研究中,可提供人群的整体生存情况和相应的死亡风险。
1. 准备数据生命表计算需要搜集大量的人口统计学数据,如人口年龄分布、死亡人数等。
根据这些数据,可以绘制出一个人口的年龄-死亡情况表。
2. 表格内容生命表中通常包含每个年龄组的人口数量、死亡数量、生存人数、死亡率、存活比率等。
通过统计和计算,可以得出各个年龄组的生存概率和死亡风险。
3. 应用和意义生命表计算可用于评估人口的整体生存情况和预测特定年龄组的死亡风险。
在临床研究中,生命表计算可以帮助医生预测患者的存活期,从而指导治疗方案的制定。
结语生存分析和生命表计算是临床研究中常用的统计方法,它们对于评估患者的生存情况和预测生存期具有重要意义。
生存分析与事件历史分析
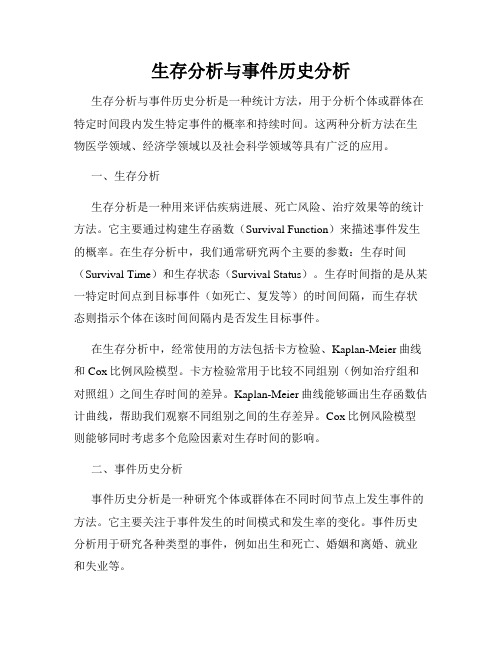
生存分析与事件历史分析生存分析与事件历史分析是一种统计方法,用于分析个体或群体在特定时间段内发生特定事件的概率和持续时间。
这两种分析方法在生物医学领域、经济学领域以及社会科学领域等具有广泛的应用。
一、生存分析生存分析是一种用来评估疾病进展、死亡风险、治疗效果等的统计方法。
它主要通过构建生存函数(Survival Function)来描述事件发生的概率。
在生存分析中,我们通常研究两个主要的参数:生存时间(Survival Time)和生存状态(Survival Status)。
生存时间指的是从某一特定时间点到目标事件(如死亡、复发等)的时间间隔,而生存状态则指示个体在该时间间隔内是否发生目标事件。
在生存分析中,经常使用的方法包括卡方检验、Kaplan-Meier曲线和Cox比例风险模型。
卡方检验常用于比较不同组别(例如治疗组和对照组)之间生存时间的差异。
Kaplan-Meier曲线能够画出生存函数估计曲线,帮助我们观察不同组别之间的生存差异。
Cox比例风险模型则能够同时考虑多个危险因素对生存时间的影响。
二、事件历史分析事件历史分析是一种研究个体或群体在不同时间节点上发生事件的方法。
它主要关注于事件发生的时间模式和发生率的变化。
事件历史分析用于研究各种类型的事件,例如出生和死亡、婚姻和离婚、就业和失业等。
事件历史分析通常使用的方法包括卡方检验、Kaplan-Meier曲线和Cox比例风险模型。
卡方检验用于比较不同群体(例如男性和女性)之间事件发生率的差异。
Kaplan-Meier曲线能够显示事件发生率随时间的变化趋势。
Cox比例风险模型则用于估计多个危险因素对事件发生率的影响。
三、生存分析与事件历史分析的应用生存分析和事件历史分析在不同领域有着广泛的应用。
在医学领域,生存分析可用于评估药物疗效、预测患者生存时间,并帮助医生制定个体化的治疗方案。
在经济学领域,生存分析可用于研究企业的存续时间、分析经济周期,并对市场趋势进行预测。
生存分析知识总结
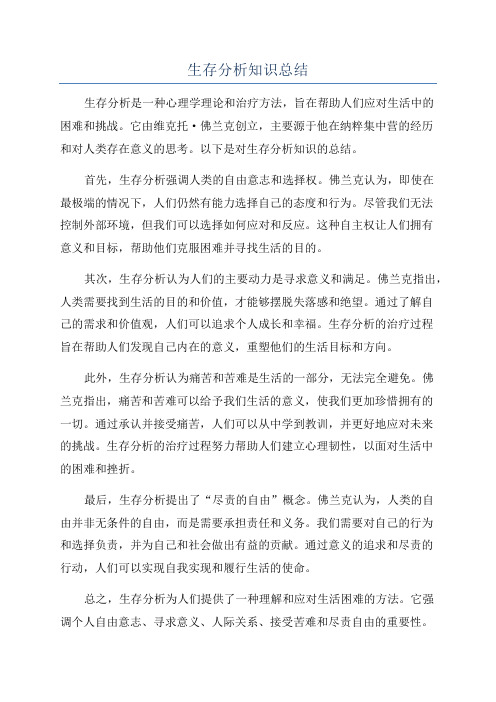
生存分析知识总结生存分析是一种心理学理论和治疗方法,旨在帮助人们应对生活中的困难和挑战。
它由维克托·佛兰克创立,主要源于他在纳粹集中营的经历和对人类存在意义的思考。
以下是对生存分析知识的总结。
首先,生存分析强调人类的自由意志和选择权。
佛兰克认为,即使在最极端的情况下,人们仍然有能力选择自己的态度和行为。
尽管我们无法控制外部环境,但我们可以选择如何应对和反应。
这种自主权让人们拥有意义和目标,帮助他们克服困难并寻找生活的目的。
其次,生存分析认为人们的主要动力是寻求意义和满足。
佛兰克指出,人类需要找到生活的目的和价值,才能够摆脱失落感和绝望。
通过了解自己的需求和价值观,人们可以追求个人成长和幸福。
生存分析的治疗过程旨在帮助人们发现自己内在的意义,重塑他们的生活目标和方向。
此外,生存分析认为痛苦和苦难是生活的一部分,无法完全避免。
佛兰克指出,痛苦和苦难可以给予我们生活的意义,使我们更加珍惜拥有的一切。
通过承认并接受痛苦,人们可以从中学到教训,并更好地应对未来的挑战。
生存分析的治疗过程努力帮助人们建立心理韧性,以面对生活中的困难和挫折。
最后,生存分析提出了“尽责的自由”概念。
佛兰克认为,人类的自由并非无条件的自由,而是需要承担责任和义务。
我们需要对自己的行为和选择负责,并为自己和社会做出有益的贡献。
通过意义的追求和尽责的行动,人们可以实现自我实现和履行生活的使命。
总之,生存分析为人们提供了一种理解和应对生活困难的方法。
它强调个人自由意志、寻求意义、人际关系、接受苦难和尽责自由的重要性。
通过生存分析,人们可以找到内在的目的和满足,拥有有意义和充实的生活。
生存分析方法在医学研究中的应用
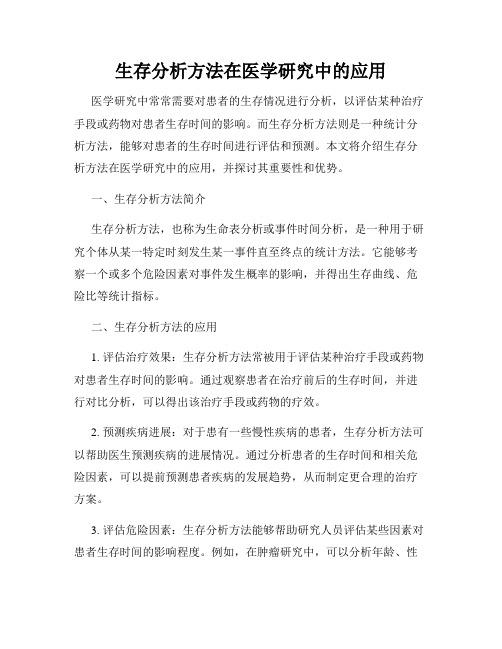
生存分析方法在医学研究中的应用医学研究中常常需要对患者的生存情况进行分析,以评估某种治疗手段或药物对患者生存时间的影响。
而生存分析方法则是一种统计分析方法,能够对患者的生存时间进行评估和预测。
本文将介绍生存分析方法在医学研究中的应用,并探讨其重要性和优势。
一、生存分析方法简介生存分析方法,也称为生命表分析或事件时间分析,是一种用于研究个体从某一特定时刻发生某一事件直至终点的统计方法。
它能够考察一个或多个危险因素对事件发生概率的影响,并得出生存曲线、危险比等统计指标。
二、生存分析方法的应用1. 评估治疗效果:生存分析方法常被用于评估某种治疗手段或药物对患者生存时间的影响。
通过观察患者在治疗前后的生存时间,并进行对比分析,可以得出该治疗手段或药物的疗效。
2. 预测疾病进展:对于患有一些慢性疾病的患者,生存分析方法可以帮助医生预测疾病的进展情况。
通过分析患者的生存时间和相关危险因素,可以提前预测患者疾病的发展趋势,从而制定更合理的治疗方案。
3. 评估危险因素:生存分析方法能够帮助研究人员评估某些因素对患者生存时间的影响程度。
例如,在肿瘤研究中,可以分析年龄、性别、肿瘤分期等因素对患者生存时间的影响,以确定哪些因素是导致患者生存状况变化的主要原因。
4. 比较生存曲线:生存分析方法可以帮助研究人员比较不同组别患者的生存情况。
通过构建生存曲线,并使用适当的统计方法对曲线进行比较,可以得出不同组别患者之间的生存差异,从而寻找可能的影响因素。
三、生存分析方法的优势1. 可以利用完整的观测数据:生存分析方法能够充分利用患者的完整观测数据,即使部分患者在研究期间内没有发生事件,也能够进行分析。
2. 考虑了时间因素:与传统的二分类方法相比,生存分析方法能够更准确地反映个体从发生事件到终点的时间。
因此,可以更好地评估危险因素对生存时间的影响。
3. 考虑了患者失访情况:在医学研究中,患者可能因为多种原因失访或丧失随访资料。
生存分析的基本方法
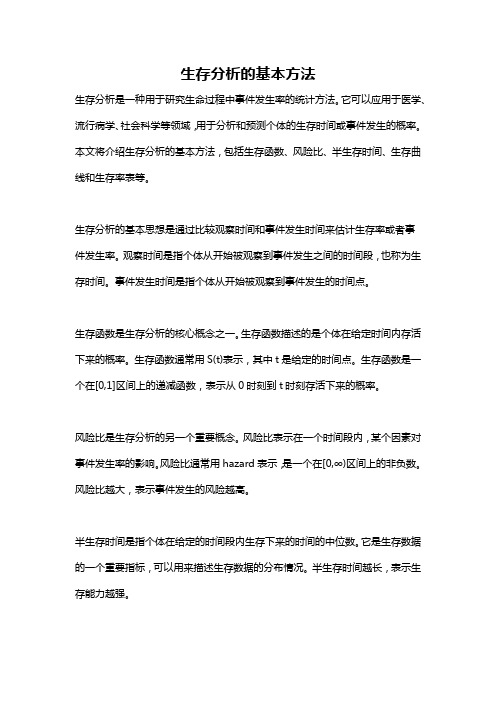
生存分析的基本方法生存分析是一种用于研究生命过程中事件发生率的统计方法。
它可以应用于医学、流行病学、社会科学等领域,用于分析和预测个体的生存时间或事件发生的概率。
本文将介绍生存分析的基本方法,包括生存函数、风险比、半生存时间、生存曲线和生存率表等。
生存分析的基本思想是通过比较观察时间和事件发生时间来估计生存率或者事件发生率。
观察时间是指个体从开始被观察到事件发生之间的时间段,也称为生存时间。
事件发生时间是指个体从开始被观察到事件发生的时间点。
生存函数是生存分析的核心概念之一。
生存函数描述的是个体在给定时间内存活下来的概率。
生存函数通常用S(t)表示,其中t是给定的时间点。
生存函数是一个在[0,1]区间上的递减函数,表示从0时刻到t时刻存活下来的概率。
风险比是生存分析的另一个重要概念。
风险比表示在一个时间段内,某个因素对事件发生率的影响。
风险比通常用hazard表示,是一个在[0,∞)区间上的非负数。
风险比越大,表示事件发生的风险越高。
半生存时间是指个体在给定的时间段内生存下来的时间的中位数。
它是生存数据的一个重要指标,可以用来描述生存数据的分布情况。
半生存时间越长,表示生存能力越强。
生存曲线是用来描述不同时间段个体存活下来的比例。
生存曲线通常是一个递减的曲线,随着时间的推移,曲线的斜率越来越陡峭,表示个体存活的概率逐渐减小。
生存率表是一种用表格形式表示的生存数据汇总。
生存率表通常包括时间段、观察个体数、事件发生个体数、累积观察个体数、累积事件发生个体数和生存函数等内容。
生存率表可以帮助研究人员更直观地了解生存数据的分布情况。
生存分析的方法还包括生存回归分析、生存树分析、生存指标筛选等。
生存回归分析是一种用于分析多个因素对生存数据的影响的方法,可以用来确定生存数据中重要的预测因素。
生存树分析是一种用于构建生存数据分类模型的方法,可以用于预测个体的存活概率。
生存指标筛选是一种用于选择生存数据中重要的预测指标的方法,可以帮助研究人员更准确地预测个体的生存时间。
生存分析(survivalanalysis)
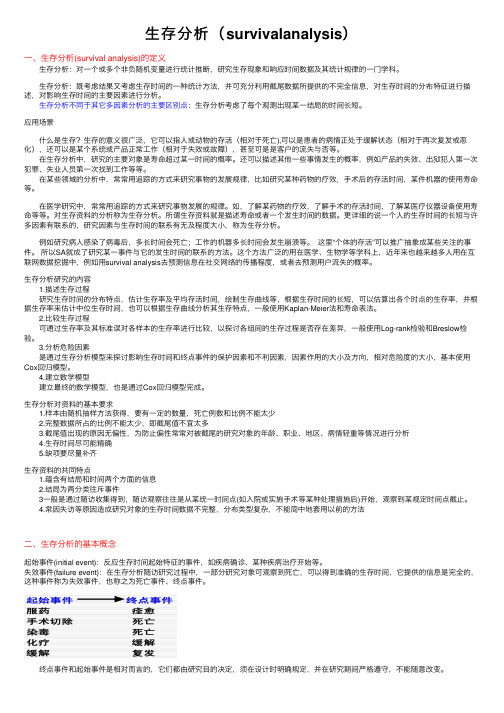
⽣存分析(survivalanalysis)⼀、⽣存分析(survival analysis)的定义 ⽣存分析:对⼀个或多个⾮负随机变量进⾏统计推断,研究⽣存现象和响应时间数据及其统计规律的⼀门学科。
⽣存分析:既考虑结果⼜考虑⽣存时间的⼀种统计⽅法,并可充分利⽤截尾数据所提供的不完全信息,对⽣存时间的分布特征进⾏描述,对影响⽣存时间的主要因素进⾏分析。
⽣存分析不同于其它多因素分析的主要区别点:⽣存分析考虑了每个观测出现某⼀结局的时间长短。
应⽤场景 什么是⽣存?⽣存的意义很⼴泛,它可以指⼈或动物的存活(相对于死亡),可以是患者的病情正处于缓解状态(相对于再次复发或恶化),还可以是某个系统或产品正常⼯作(相对于失效或故障),甚⾄可是是客户的流失与否等。
在⽣存分析中,研究的主要对象是寿命超过某⼀时间的概率。
还可以描述其他⼀些事情发⽣的概率,例如产品的失效、出狱犯⼈第⼀次犯罪、失业⼈员第⼀次找到⼯作等等。
在某些领域的分析中,常常⽤追踪的⽅式来研究事物的发展规律,⽐如研究某种药物的疗效,⼿术后的存活时间,某件机器的使⽤寿命等。
在医学研究中,常常⽤追踪的⽅式来研究事物发展的规律。
如,了解某药物的疗效,了解⼿术的存活时间,了解某医疗仪器设备使⽤寿命等等。
对⽣存资料的分析称为⽣存分析。
所谓⽣存资料就是描述寿命或者⼀个发⽣时间的数据。
更详细的说⼀个⼈的⽣存时间的长短与许多因素有联系的,研究因素与⽣存时间的联系有⽆及程度⼤⼩,称为⽣存分析。
例如研究病⼈感染了病毒后,多长时间会死亡;⼯作的机器多长时间会发⽣崩溃等。
这⾥“个体的存活”可以推⼴抽象成某些关注的事件。
所以SA就成了研究某⼀事件与它的发⽣时间的联系的⽅法。
这个⽅法⼴泛的⽤在医学、⽣物学等学科上,近年来也越来越多⼈⽤在互联⽹数据挖掘中,例如⽤survival analysis去预测信息在社交⽹络的传播程度,或者去预测⽤户流失的概率。
⽣存分析研究的内容 1.描述⽣存过程 研究⽣存时间的分布特点,估计⽣存率及平均存活时间,绘制⽣存曲线等,根据⽣存时间的长短,可以估算出各个时点的⽣存率,并根据⽣存率来估计中位⽣存时间,也可以根据⽣存曲线分析其⽣存特点,⼀般使⽤Kaplan-Meier法和寿命表法。
生存分析公式生存函数风险比生存曲线
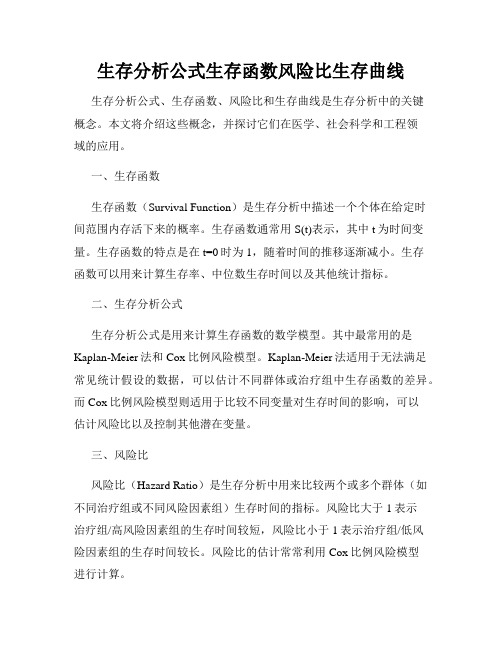
生存分析公式生存函数风险比生存曲线生存分析公式、生存函数、风险比和生存曲线是生存分析中的关键概念。
本文将介绍这些概念,并探讨它们在医学、社会科学和工程领域的应用。
一、生存函数生存函数(Survival Function)是生存分析中描述一个个体在给定时间范围内存活下来的概率。
生存函数通常用S(t)表示,其中t为时间变量。
生存函数的特点是在t=0时为1,随着时间的推移逐渐减小。
生存函数可以用来计算生存率、中位数生存时间以及其他统计指标。
二、生存分析公式生存分析公式是用来计算生存函数的数学模型。
其中最常用的是Kaplan-Meier法和Cox比例风险模型。
Kaplan-Meier法适用于无法满足常见统计假设的数据,可以估计不同群体或治疗组中生存函数的差异。
而Cox比例风险模型则适用于比较不同变量对生存时间的影响,可以估计风险比以及控制其他潜在变量。
三、风险比风险比(Hazard Ratio)是生存分析中用来比较两个或多个群体(如不同治疗组或不同风险因素组)生存时间的指标。
风险比大于1表示治疗组/高风险因素组的生存时间较短,风险比小于1表示治疗组/低风险因素组的生存时间较长。
风险比的估计常常利用Cox比例风险模型进行计算。
四、生存曲线生存曲线(Survival Curve)是反映个体生存概率随时间变化的图形。
生存曲线通常以时间为横轴,以生存函数为纵轴,表达从给定时间开始,个体在不同时间点存活下来的概率。
生存曲线可以用于比较不同群体或治疗组之间的生存差异,并可通过Kaplan-Meier法绘制。
在医学领域,生存分析广泛应用于肿瘤学、流行病学和临床研究中,用于评估治疗效果、预测生存时间以及分析相关风险因素。
例如,在肿瘤学中,生存曲线可以帮助医生评估肿瘤患者的存活率,并制定更合适的治疗方案。
在社会科学领域,生存分析可以用于研究人口学和行为科学中的各种事件,如婚姻研究、失业研究和犯罪研究。
通过生存分析,研究者可以分析个体在给定事件(如离婚、失业或犯罪)发生之前的生存时间及相关风险因素,为决策制定提供参考。
- 1、下载文档前请自行甄别文档内容的完整性,平台不提供额外的编辑、内容补充、找答案等附加服务。
- 2、"仅部分预览"的文档,不可在线预览部分如存在完整性等问题,可反馈申请退款(可完整预览的文档不适用该条件!)。
- 3、如文档侵犯您的权益,请联系客服反馈,我们会尽快为您处理(人工客服工作时间:9:00-18:30)。
Chapter7Survival ModelsOurfinal chapter concerns models for the analysis of data which have three main characteristics:(1)the dependent variable or response is the waiting time until the occurrence of a well-defined event,(2)observations are cen-sored,in the sense that for some units the event of interest has not occurred at the time the data are analyzed,and(3)there are predictors or explanatory variables whose effect on the waiting time we wish to assess or control.We start with some basic definitions.7.1The Hazard and Survival FunctionsLet T be a non-negative random variable representing the waiting time until the occurrence of an event.For simplicity we will adopt the terminology of survival analysis,referring to the event of interest as‘death’and to the waiting time as‘survival’time,but the techniques to be studied have much wider applicability.They can be used,for example,to study age at marriage, the duration of marriage,the intervals between successive births to a woman, the duration of stay in a city(or in a job),and the length of life.The observant demographer will have noticed that these examples include the fields of fertility,mortality and migration.7.1.1The Survival FunctionWe will assume for now that T is a continuous random variable with prob-ability density function(p.d.f.)f(t)and cumulative distribution function (c.d.f.)F(t)=Pr{T≤t},giving the probability that the event has oc-curred by duration t.G.Rodr´ıguez.Revised September,20072CHAPTER7.SURVIVAL MODELS It will often be convenient to work with the complement of the c.d.f,thesurvival functionS(t)=Pr{T>t}=1−F(t)= ∞tf(x)dx,(7.1)which gives the probability of being alive at duration t,or more generally, the probability that the event of interest has not occurred by duration t. 7.1.2The Hazard FunctionAn alternative characterization of the distribution of T is given by the hazard function,or instantaneous rate of occurrence of the event,defined asλ(t)=limdt→0Pr{t<T≤t+dt|T>t}dt.(7.2)The numerator of this expression is the conditional probability that the event will occur in the interval(t,t+dt)given that it has not occurred before,and the denominator is the width of the interval.Dividing one by the other we obtain a rate of event occurrence per unit of time.Taking the limit as the width of the interval goes down to zero,we obtain an instantaneous rate of occurrence.The conditional probability in the numerator may be written as the ratio of the joint probability that T is in the interval(t,t+dt)and T>t(which is,of course,the same as the probability that t is in the interval),to the probability of the condition T>t.The former may be written as f(t)dt for small dt,while the latter is S(t)by definition.Dividing by dt and passing to the limit gives the useful resultλ(t)=f(t)S(t),(7.3)which some authors give as a definition of the hazard function.In words,the rate of occurrence of the event at duration t equals the density of events at t, divided by the probability of surviving to that duration without experiencing the event.Note from Equation7.1that−f(t)is the derivative of S(t).This suggests rewriting Equation7.3asλ(t)=−ddtlog S(t).If we now integrate from0to t and introduce the boundary condition S(0)= 1(since the event is sure not to have occurred by duration0),we can solve7.1.THE HAZARD AND SURVIVAL FUNCTIONS3 the above expression to obtain a formula for the probability of surviving to duration t as a function of the hazard at all durations up to t:S(t)=exp{− tλ(x)dx}.(7.4)This expression should be familiar to demographers.The integral in curly brackets in this equation is called the cumulative hazard(or cumulative risk)and is denotedΛ(t)= tλ(x)dx.(7.5)You may think ofΛ(t)as the sum of the risks you face going from duration 0to t.These results show that the survival and hazard functions provide alter-native but equivalent characterizations of the distribution of T.Given the survival function,we can always differentiate to obtain the density and then calculate the hazard using Equation7.3.Given the hazard,we can always integrate to obtain the cumulative hazard and then exponentiate to obtain the survival function using Equation7.4.An example will helpfix ideas. Example:The simplest possible survival distribution is obtained by assuming a constant risk over time,so the hazard isλ(t)=λfor all t.The corresponding survival function isS(t)=exp{−λt}.This distribution is called the exponential distribution with parameterλ. The density may be obtained multiplying the survivor function by the hazard to obtainf(t)=λexp{−λt}.The mean turns out to be1/λ.This distribution plays a central role in sur-vival analysis,although it is probably too simple to be useful in applications in its own right.27.1.3Expectation of LifeLetµdenote the mean or expected value of T.By definition,one would calculateµmultiplying t by the density f(t)and integrating,soµ= ∞tf(t)dt.4CHAPTER7.SURVIVAL MODELS Integrating by parts,and making use of the fact that−f(t)is the derivative of S(t),which has limits or boundary conditions S(0)=1and S(∞)=0,one can show thatµ= ∞S(t)dt.(7.6)In words,the mean is simply the integral of the survival function.7.1.4A Note on Improper Random Variables*So far we have assumed implicitly that the event of interest is bound to occur, so that S(∞)=0.In words,given enough time the proportion surviving goes down to zero.This condition implies that the cumulative hazard must diverge,i.e.we must haveΛ(∞)=∞.Intuitively,the event will occur with certainty only if the cumulative risk over a long period is sufficiently high.There are,however,many events of possible interest that are not bound to occur.Some men and women remain forever single,some birth intervals never close,and some people are happy enough at their jobs that they never leave.What can we do in these cases?There are two approaches one can take.One approach is to note that we can still calculate the hazard and survival functions,which are well defined even if the event of interest is not bound to occur.For example we can study marriage in the entire population, which includes people who will never marry,and calculate marriage rates and proportions single.In this example S(t)would represent the proportion still single at age t and S(∞)would represent the proportion who never marry.One limitation of this approach is that if the event is not certain to occur,then the waiting time T could be undefined(or infinite)and thus not a proper random variable.Its density,which could be calculated from the hazard and survival,would be improper,i.e.it would fail to integrate to one.Obviously,the mean waiting time would not be defined.In terms of our example,we cannot calculate mean age at marriage for the entire population,simply because not everyone marries.But this limitation is of no great consequence if interest centers on the hazard and survivor functions, rather than the waiting time.In the marriage example we can even calculate a median age at marriage,provided we define it as the age by which half the population has married.The alternative approach is to condition the analysis on the event actu-ally occurring.In terms of our example,we could study marriage(perhaps retrospectively)for people who eventually marry,since for this group the7.2.CENSORING AND THE LIKELIHOOD FUNCTION5 actual waiting time T is always well defined.In this case we can calculate not just the conditional hazard and survivor functions,but also the mean. In our marriage example,we could calculate the mean age at marriage for those who marry.We could even calculate a conventional median,defined as the age by which half the people who will eventually marry have done so.It turns out that the conditional density,hazard and survivor function for those who experience the event are related to the unconditional density, hazard and survivor for the entire population.The conditional density isf∗(t)=f(t)1−S(∞),and it integrates to one.The conditional survivor function isS∗(t)=S(t)−S(∞) 1−S(∞),and goes down to zero as t→∞.Dividing the density by the survivor function,wefind the conditional hazard to beλ∗(t)=f∗(t)S∗(t)=f(t)S(t)−S(∞).Derivation of the mean waiting time for those who experience the event is left as an exercise for the reader.Whichever approach is adopted,care must be exercised to specify clearly which hazard or survival is being used.For example,the conditional hazard for those who eventually experience the event is always higher than the unconditional hazard for the entire population.Note also that in most cases all we observe is whether or not the event has occurred.If the event has not occurred,we may be unable to determine whether it will eventually occur. In this context,only the unconditional hazard may be estimated from data, but one can always translate the results into conditional expressions,if so desired,using the results given above.7.2Censoring and The Likelihood FunctionThe second distinguishing feature of thefield of survival analysis is censoring: the fact that for some units the event of interest has occurred and therefore we know the exact waiting time,whereas for others it has not occurred,and all we know is that the waiting time exceeds the observation time.6CHAPTER7.SURVIVAL MODELS7.2.1Censoring MechanismsThere are several mechanisms that can lead to censored data.Under censor-ing of Type I,a sample of n units is followed for afixed timeτ.The number of units experiencing the event,or the number of‘deaths’,is random,but the total duration of the study isfixed.The fact that the duration isfixed may be an important practical advantage in designing a follow-up study.In a simple generalization of this scheme,calledfixed censoring,each unit has a potential maximum observation timeτi for i=1,...,n which may differ from one case to the next but is neverthelessfixed in advance. The probability that unit i will be alive at the end of her observation time is S(τi),and the total number of deaths is again random.Under censoring of Type II,a sample of n units is followed as long as necessary until d units have experienced the event.In this design the number of deaths d,which determines the precision of the study,isfixed in advance and can be used as a design parameter.Unfortunately,the total duration of the study is then random and cannot be known with certainty in advance.In a more general scheme called random censoring,each unit has as-sociated with it a potential censoring time C i and a potential lifetime T i, which are assumed to the independent random variables.We observe Y i= min{C i,T i},the minimum of the censoring and life times,and an indicator variable,often called d i orδi,that tells us whether observation terminated by death or by censoring.All these schemes have in common the fact that the censoring mechanism is non-informative and they all lead to essentially the same likelihood func-tion.The weakest assumption required to obtain this common likelihood is that the censoring of an observation should not provide any information regarding the prospects of survival of that particular unit beyond the cen-soring time.In fact,the basic assumption that we will make is simply this: all we know for an observation censored at duration t is that the lifetime exceeds t.7.2.2The Likelihood Function for Censored DataSuppose then that we have n units with lifetimes governed by a survivor function S(t)with associated density f(t)and hazardλ(t).Suppose unit i is observed for a time t i.If the unit died at t i,its contribution to the likelihood function is the density at that duration,which can be written as the product of the survivor and hazard functionsL i=f(t i)=S(t i)λ(t i).7.2.CENSORING AND THE LIKELIHOOD FUNCTION7 If the unit is still alive at t i,all we know under non-informative censoring is that the lifetime exceeds t i.The probability of this event isL i=S(t i),which becomes the contribution of a censored observation to the likelihood.Note that both types of contribution share the survivor function S(t i), because in both cases the unit lived up to time t i.A death multiplies this contribution by the hazardλ(t i),but a censored observation does not.We can write the two contributions in a single expression.To this end,let d i be a death indicator,taking the value one if unit i died and the value zero otherwise.Then the likelihood function may be written as followsL=ni=1L i=iλ(t i)d i S(t i).Taking logs,and recalling the expression linking the survival function S(t) to the cumulative hazard functionΛ(t),we obtain the log-likelihood function for censored survival datalog L=ni=1{d i logλ(t i)−Λ(t i)}.(7.7)We now consider an example to reinforce these ideas.Example:Suppose we have a sample of n censored observations from an exponential distribution.Let t i be the observation time and d i the death indicator for unit i.In the exponential distributionλ(t)=λfor all t.The cumulative risk turns out to be the integral of a constant and is thereforeΛ(t)=λing these two results on Equation7.7gives the log-likelihood functionlog L={d i logλ−λt i}.Let D= di denote the total number of deaths,and let T=ti denote thetotal observation(or exposure)time.Then we can rewrite the log-likelihood as a function of these totals to obtainlog L=D logλ−λT.(7.8) Differentiating this expression with respect toλwe obtain the score functionu(λ)=Dλ−T,8CHAPTER7.SURVIVAL MODELS and setting the score to zero gives the maximum likelihood estimator of the hazardˆλ=DT,(7.9) the total number of deaths divided by the total exposure time.Demogra-phers will recognize this expression as the general definition of a death rate. Note that the estimator is optimal(in a maximum likelihood sense)only if the risk is constant and does not depend on age.We can also calculate the observed information by taking minus the sec-ond derivative of the score,which isI(λ)=D λ2.To obtain the expected information we need to calculate the expected num-ber of deaths,but this depends on the censoring scheme.For example under Type I censoring withfixed durationτ,one would expect n(1−S(τ))deaths. Under Type II censoring the number of deaths would have beenfixed in ad-vance.Under some schemes calculation of the expectation may be fairly complicated if not impossible.A simpler alternative is to use the observed information,estimated using the m.l.e.ofλgiven in ing this approach,the large sample variance of the m.l.e.of the hazard rate may be estimated asˆvar(ˆλ)=D T,a result that leads to large-sample tests of hypotheses and confidence inter-vals forλ.If there are no censored cases,so that d i=1for all i and D=n,then the results obtained here reduce to standard maximum likelihood estimation for the exponential distribution,and the m.l.e.ofλturns out to be the reciprocal of the sample mean.It may be interesting to note in passing that the log-likelihood for cen-sored exponential data given in Equation7.8coincides exactly(except for constants)with the log-likelihood that would be obtained by treating D as a Poisson random variable with meanλT.To see this point,you should write the Poisson log-likelihood when D∼P(λT),and note that it differs from Equation7.8only in the presence of a term D log(T),which is a constant depending on the data but not on the parameterλ.Thus,treating the deaths as Poisson conditional on exposure time leads to exactly the same estimates(and standard errors)as treating the exposure7.3.APPROACHES TO SURVIVAL MODELING9 times as censored observations from an exponential distribution.This result will be exploited below to link survival models to generalized linear models with Poisson error structure.7.3Approaches to Survival ModelingUp to this point we have been concerned with a homogeneous population, where the lifetimes of all units are governed by the same survival function S(t).We now introduce the third distinguishing characteristic of survival models—the presence of a vector of covariates or explanatory variables that may affect survival time—and consider the general problem of modeling these effects.7.3.1Accelerated Life Models*Let T i be a random variable representing the(possibly unobserved)survival time of the i-th unit.Since T i must be non-negative,we might consider modeling its logarithm using a conventional linear model,saylog T i=x iβ+ i,where i is a suitable error term,with a distribution to be specified.This model specifies the distribution of log-survival for the i-th unit as a simple shift of a standard or baseline distribution represented by the error term.Exponentiating this equation,we obtain a model for the survival time itselfT i=exp{x iβ}T0i,where we have written T0i for the exponentiated error term.It will also be convenient to useγi as shorthand for the multiplicative effect exp{x iβ}of the covariates.Interpretation of the parameters follows along standard lines.Consider, for example,a model with a constant and a dummy variable x representing a factor with two levels,say groups one and zero.Suppose the corresponding multiplicative effect isγ=2,so the coefficient of x isβ=log(2)=0.6931. Then we would conclude that people in group one live twice as long as people in group zero.There is an interesting alternative interpretation that explains the name ‘accelerated life’used for this model.Let S0(t)denote the survivor function in group zero,which will serve as a reference group,and let S1(t)denote the10CHAPTER7.SURVIVAL MODELS survivor function in group one.Under this model,S1(t)=S0(t/γ).In words,the probability that a member of group one will be alive at age t is exactly the same as the probability that a member of group zero will be alive at age t/γ.Forγ=2,this would be half the age,so the probability that a member of group one would be alive at age40(or60)would be the same as the probability that a member of group zero would be alive at age 20(or30).Thus,we may think ofγas affecting the passage of time.In our example,people in group zero age‘twice as fast’.For the record,the corresponding hazard functions are related byλ1(t)=λ0(t/γ)/γ,so ifγ=2,at any given age people in group one would be exposed to half the risk of people in group zero half their age.The name‘accelerated life’stems from industrial applications where items are put to test under substantially worse conditions than they are likely to encounter in real life,so that tests can be completed in a shorter time.Different kinds of parametric models are obtained by assuming different distributions for the error term.If the i are normally distributed,then we obtain a log-normal model for the T i.Estimation of this model for censored data by maximum likelihood is known in the econometric literature as a Tobit model.Alternatively,if the i have an extreme value distribution with p.d.f.f( )=exp{ −exp{ }},then T0i has an exponential distribution,and we obtain the exponential regression model,where T i is exponential with hazardλi satisfying the log-linear modellogλi=x iβ.An example of a demographic model that belongs to the family of accelerated life models is the Coale-McNeil model offirst marriage frequencies,where the proportion ever married at age a in a given population is written asF(a)=cF0(a−a0k),7.3.APPROACHES TO SURVIVAL MODELING11 where F0is a model schedule of proportions married by age,among women who will ever marry,based on historical data from Sweden;c is the propor-tion who will eventually marry,a0is the age at which marriage starts,and k is the pace at which marriage proceeds relative to the Swedish standard.Accelerated life models are essentially standard regression models applied to the log of survival time,and except for the fact that observations are censored,pose no new estimation problems.Once the distribution of the error term is chosen,estimation proceeds by maximizing the log-likelihood for censored data described in the previous subsection.For further details, see Kalbfleish and Prentice(1980).7.3.2Proportional Hazard ModelsA large family of models introduced by Cox(1972)focuses directly on the hazard function.The simplest member of the family is the proportional hazards model,where the hazard at time t for an individual with covariates x i(not including a constant)is assumed to beλi(t|x i)=λ0(t)exp{x iβ}.(7.10) In this modelλ0(t)is a baseline hazard function that describes the risk for individuals with x i=0,who serve as a reference cell or pivot,and exp{x iβ} is the relative risk,a proportionate increase or reduction in risk,associated with the set of characteristics x i.Note that the increase or reduction in risk is the same at all durations t.Tofix ideas consider a two-sample problem where we have a dummy variable x which serves to identify groups one and zero.Then the model isλi(t|x)=λ0(t)if x=0,λ0(t)eβif x=1..Thus,λ0(t)represents the risk at time t in group zero,andγ=exp{β} represents the ratio of the risk in group one relative to group zero at any time t.Ifγ=1(orβ=0)then the risks are the same in the two groups.If γ=2(orβ=0.6931),then the risk for an individual in group one at any given age is twice the risk of a member of group zero who has the same age.Note that the model separates clearly the effect of time from the effect of the covariates.Taking logs,wefind that the proportional hazards model is a simple additive model for the log of the hazard,withlogλi(t|x i)=α0(t)+x iβ,12CHAPTER7.SURVIVAL MODELS whereα0(t)=logλ0(t)is the log of the baseline hazard.As in all additive models,we assume that the effect of the covariates x is the same at all times or ages t.The similarity between this expression and a standard analysis of covariance model with parallel lines should not go unnoticed.Returning to Equation7.10,we can integrate both sides from0to t to obtain the cumulative hazardsΛi(t|x i)=Λ0(t)exp{x iβ},which are also proportional.Changing signs and exponentiating we obtain the survivor functionsS i(t|x i)=S0(t)exp{x iβ},(7.11) where S0(t)=exp{−Λ0(t)}is a baseline survival function.Thus,the effect of the covariate values x i on the survivor function is to raise it to a power given by the relative risk exp{x iβ}.In our two-group example with a relative risk ofγ=2,the probability that a member of group one will be alive at any given age t is the square of the probability that a member of group zero would be alive at the same age.7.3.3The Exponential and Weibull ModelsDifferent kinds of proportional hazard models may be obtained by making different assumptions about the baseline survival function,or equivalently, the baseline hazard function.For example if the baseline risk is constant over time,soλ0(t)=λ0,say,we obtain the exponential regression model, whereλi(t,x i)=λ0exp{x iβ}.Interestingly,the exponential regression model belongs to both the propor-tional hazards and the accelerated life families.If the baseline risk is a constant and you double or triple the risk,the new risk is still constant (just higher).Perhaps less obviously,if the baseline risk is constant and you imagine timeflowing twice or three times as fast,the new risk is doubled or tripled but is still constant over time,so we remain in the exponential family.You may be wondering whether there are other cases where the two models coincide.The answer is yes,but not many.In fact,there is only one distribution where they do,and it includes the exponential as a special case.7.3.APPROACHES TO SURVIVAL MODELING13 The one case where the two families coincide is the Weibull distribution, which has survival functionS(t)=exp{−(λt)p}and hazard functionλ(t)=pλ(λt)p−1,for parametersλ>0and p>0.If p=1,this model reduces to the exponential and has constant risk over time.If p>1,then the risk increases over time.If p<1,then the risk decreases over time.In fact,taking logs in the expression for the hazard function,we see that the log of the Weibull risk is a linear function of log time with slope p−1.If we pick the Weibull as a baseline risk and then multiply the hazard by a constantγin a proportional hazards framework,the resulting distribution turns out to be still a Weibull,so the family is closed under proportionality of hazards.If we pick the Weibull as a baseline survival and then speed up the passage of time in an accelerated life framework,dividing time by a constantγ,the resulting distribution is still a Weibull,so the family is closed under acceleration of time.For further details on this distribution see Cox and Oakes(1984)or Kalbfleish and Prentice(1980),who prove the equivalence of the two Weibull models.7.3.4Time-varying CovariatesSo far we have considered explicitly only covariates that arefixed over time. The local nature of the proportional hazards model,however,lends itself easily to extensions that allows for covariates that change over time.Let us consider a few examples.Suppose we are interested in the analysis of birth spacing,and study the interval from the birth of one child to the birth of the next.One of the possible predictors of interest is the mother’s education,which in most cases can be taken to befixed over time.Suppose,however,that we want to introduce breastfeeding status of the child that begins the interval.Assuming the child is breastfed,this variable would take the value one(‘yes’)from birth until the child is weaned,at which time it would take the value zero(‘no’).This is a simple example of a predictor that can change value only once.A more elaborate analysis could rely on frequency of breastfeeding in a24-hour period.This variable could change values from day to day.For example a sequence of values for one woman could be4,6,5,6,5,4,...14CHAPTER7.SURVIVAL MODELS Let x i(t)denote the value of a vector of covariates for individual i at time or duration t.Then the proportional hazards model may be generalized toλi(t,x i(t))=λ0(t)exp{x i(t) β}.(7.12) The separation of duration and covariate effects is not so clear now,and on occasion it may be difficult to identify effects that are highly collinear with time.If all children were weaned when they are around six months old,for example,it would be difficult to identify effects of breastfeeding from general duration effects without additional information.In such cases one might still prefer a time-varying covariate,however,as a more meaningful predictor of risk than the mere passage of time.Calculation of survival functions when we have time-varying covariates is a little bit more complicated,because we need to specify a path or trajectory for each variable.In the birth intervals example one could calculate a survival function for women who breastfeed for six months and then wean.This would be done by using the hazard corresponding to x(t)=0for months0 to6and then the hazard corresponding to x(t)=1for months6onwards. Unfortunately,the simplicity of Equation7.11is lost;we can no longer simply raise the baseline survival function to a power.Time-varying covariates can be introduced in the context of accelerated life models,but this is not so simple and has rarely been done in applications. See Cox and Oakes(1984,p.66)for more information.7.3.5Time-dependent EffectsThe model may also be generalized to allow for effects that vary over time, and therefore are no longer proportional.It is quite possible,for example, that certain social characteristics might have a large impact on the hazard for children shortly after birth,but may have a relatively small impact later in life.To accommodate such models we may writeλi(t,x i)=λ0(t)exp{x iβ(t)},where the parameterβ(t)is now a function of time.This model allows for great generality.In the two-sample case,for ex-ample,the model may be written asλi(t|x)=λ0(t)if x=0λ0(t)eβ(t)if x=1,which basically allows for two arbitrary hazard functions,one for each group. Thus,this is a form of saturated model.。