大数据外文翻译文献
大数据领域学术文章英语作文格式

大数据领域学术文章英语作文格式## Big Data: A Comprehensive Review.### Introduction.Big data refers to massive, complex, and rapidly generated datasets that are difficult to process using traditional data management tools. The advent of big data has revolutionized various industries, from healthcare to finance, transportation, and agriculture. In this paper, we present a comprehensive review of big data, including its characteristics, challenges, opportunities, and applications.### Characteristics of Big Data.Big data is often characterized by the following attributes:Volume: Big data datasets are massive, typicallyranging from terabytes to petabytes or even exabytes in size.Variety: Big data comes in various formats, including structured, semi-structured. and unstructured data.Velocity: Big data is generated rapidly and continuously, requiring real-time or near-real-time processing.Veracity: Big data quality can vary, and it isessential to address data cleansing and validation.### Challenges in Big Data Analytics.Big data analytics presents several challenges:Data storage and management: Storing and managing large and diverse datasets require efficient and scalable data storage solutions.Data processing: Traditional data processing tools areoften inadequate for handling big data, necessitating specialized big data processing techniques.Data analysis: Extracting meaningful insights from big data requires advanced analytics techniques and machine learning algorithms.Data security and privacy: Protecting big data from unauthorized access, breaches, and data loss is a significant challenge.### Opportunities of Big Data.Despite the challenges, big data presents numerous opportunities:Improved decision-making: Big data analytics enables data-driven decision-making, providing invaluable insights into customer behavior, market trends, and operational patterns.Predictive analytics: Big data allows for predictiveanalytics, identifying patterns and forecasting future events.Real-time analytics: Processing big data in near-real-time enables instant decision-making and rapid response to changing conditions.Innovation: Big data analytics drives innovation by fostering new products, services, and business models.### Applications of Big Data.Big data finds applications in numerous domains:Healthcare: Big data analytics helps improve patient diagnosis, treatment, and disease prevention.Finance: Big data is used for risk assessment, fraud detection, and personalized financial services.Transportation: Big data optimizes traffic flow, improves safety, and enhances the overall transportationsystem.Agriculture: Big data supports precision farming, crop yield prediction, and sustainable agriculture practices.Retail: Big data analytics enables personalized recommendations, customer segmentation, and supply chain optimization.### Conclusion.Big data has emerged as a transformative force in the modern world. Its vast volume, variety, velocity, and veracity present challenges but also offer unprecedented opportunities for data-driven decision-making, predictive analytics, real-time insights, and innovation. As the amount of data continues to grow exponentially, the role of big data analytics will only become more critical in shaping the future of various industries and sectors.### 中文回答:## 大数据,一个全面的评论。
大数据外文翻译参考文献综述
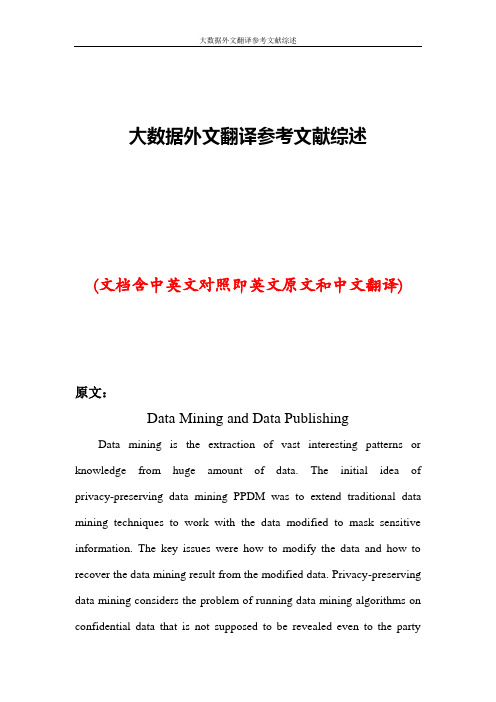
大数据外文翻译参考文献综述(文档含中英文对照即英文原文和中文翻译)原文:Data Mining and Data PublishingData mining is the extraction of vast interesting patterns or knowledge from huge amount of data. The initial idea of privacy-preserving data mining PPDM was to extend traditional data mining techniques to work with the data modified to mask sensitive information. The key issues were how to modify the data and how to recover the data mining result from the modified data. Privacy-preserving data mining considers the problem of running data mining algorithms on confidential data that is not supposed to be revealed even to the partyrunning the algorithm. In contrast, privacy-preserving data publishing (PPDP) may not necessarily be tied to a specific data mining task, and the data mining task may be unknown at the time of data publishing. PPDP studies how to transform raw data into a version that is immunized against privacy attacks but that still supports effective data mining tasks. Privacy-preserving for both data mining (PPDM) and data publishing (PPDP) has become increasingly popular because it allows sharing of privacy sensitive data for analysis purposes. One well studied approach is the k-anonymity model [1] which in turn led to other models such as confidence bounding, l-diversity, t-closeness, (α,k)-anonymity, etc. In particular, all known mechanisms try to minimize information loss and such an attempt provides a loophole for attacks. The aim of this paper is to present a survey for most of the common attacks techniques for anonymization-based PPDM & PPDP and explain their effects on Data Privacy.Although data mining is potentially useful, many data holders are reluctant to provide their data for data mining for the fear of violating individual privacy. In recent years, study has been made to ensure that the sensitive information of individuals cannot be identified easily.Anonymity Models, k-anonymization techniques have been the focus of intense research in the last few years. In order to ensure anonymization of data while at the same time minimizing the informationloss resulting from data modifications, everal extending models are proposed, which are discussed as follows.1.k-Anonymityk-anonymity is one of the most classic models, which technique that prevents joining attacks by generalizing and/or suppressing portions of the released microdata so that no individual can be uniquely distinguished from a group of size k. In the k-anonymous tables, a data set is k-anonymous (k ≥ 1) if each record in the data set is in- distinguishable from at least (k . 1) other records within the same data set. The larger the value of k, the better the privacy is protected. k-anonymity can ensure that individuals cannot be uniquely identified by linking attacks.2. Extending ModelsSince k-anonymity does not provide sufficient protection against attribute disclosure. The notion of l-diversity attempts to solve this problem by requiring that each equivalence class has at least l well-represented value for each sensitive attribute. The technology of l-diversity has some advantages than k-anonymity. Because k-anonymity dataset permits strong attacks due to lack of diversity in the sensitive attributes. In this model, an equivalence class is said to have l-diversity if there are at least l well-represented value for the sensitive attribute. Because there are semantic relationships among the attribute values, and different values have very different levels of sensitivity. Afteranonymization, in any equivalence class, the frequency (in fraction) of a sensitive value is no more than α.3. Related Research AreasSeveral polls show that the public has an in- creased sense of privacy loss. Since data mining is often a key component of information systems, homeland security systems, and monitoring and surveillance systems, it gives a wrong impression that data mining is a technique for privacy intrusion. This lack of trust has become an obstacle to the benefit of the technology. For example, the potentially beneficial data mining re- search project, Terrorism Information Awareness (TIA), was terminated by the US Congress due to its controversial procedures of collecting, sharing, and analyzing the trails left by individuals. Motivated by the privacy concerns on data mining tools, a research area called privacy-reserving data mining (PPDM) emerged in 2000. The initial idea of PPDM was to extend traditional data mining techniques to work with the data modified to mask sensitive information. The key issues were how to modify the data and how to recover the data mining result from the modified data. The solutions were often tightly coupled with the data mining algorithms under consideration. In contrast, privacy-preserving data publishing (PPDP) may not necessarily tie to a specific data mining task, and the data mining task is sometimes unknown at the time of data publishing. Furthermore, some PPDP solutions emphasize preserving the datatruthfulness at the record level, but PPDM solutions often do not preserve such property. PPDP Differs from PPDM in Several Major Ways as Follows :1) PPDP focuses on techniques for publishing data, not techniques for data mining. In fact, it is expected that standard data mining techniques are applied on the published data. In contrast, the data holder in PPDM needs to randomize the data in such a way that data mining results can be recovered from the randomized data. To do so, the data holder must understand the data mining tasks and algorithms involved. This level of involvement is not expected of the data holder in PPDP who usually is not an expert in data mining.2) Both randomization and encryption do not preserve the truthfulness of values at the record level; therefore, the released data are basically meaningless to the recipients. In such a case, the data holder in PPDM may consider releasing the data mining results rather than the scrambled data.3) PPDP primarily “anonymizes” the data by hiding the identity of record owners, whereas PPDM seeks to directly hide the sensitive data. Excellent surveys and books in randomization and cryptographic techniques for PPDM can be found in the existing literature. A family of research work called privacy-preserving distributed data mining (PPDDM) aims at performing some data mining task on a set of private databasesowned by different parties. It follows the principle of Secure Multiparty Computation (SMC), and prohibits any data sharing other than the final data mining result. Clifton et al. present a suite of SMC operations, like secure sum, secure set union, secure size of set intersection, and scalar product, that are useful for many data mining tasks. In contrast, PPDP does not perform the actual data mining task, but concerns with how to publish the data so that the anonymous data are useful for data mining. We can say that PPDP protects privacy at the data level while PPDDM protects privacy at the process level. They address different privacy models and data mining scenarios. In the field of statistical disclosure control (SDC), the research works focus on privacy-preserving publishing methods for statistical tables. SDC focuses on three types of disclosures, namely identity disclosure, attribute disclosure, and inferential disclosure. Identity disclosure occurs if an adversary can identify a respondent from the published data. Revealing that an individual is a respondent of a data collection may or may not violate confidentiality requirements. Attribute disclosure occurs when confidential information about a respondent is revealed and can be attributed to the respondent. Attribute disclosure is the primary concern of most statistical agencies in deciding whether to publish tabular data. Inferential disclosure occurs when individual information can be inferred with high confidence from statistical information of the published data.Some other works of SDC focus on the study of the non-interactive query model, in which the data recipients can submit one query to the system. This type of non-interactive query model may not fully address the information needs of data recipients because, in some cases, it is very difficult for a data recipient to accurately construct a query for a data mining task in one shot. Consequently, there are a series of studies on the interactive query model, in which the data recipients, including adversaries, can submit a sequence of queries based on previously received query results. The database server is responsible to keep track of all queries of each user and determine whether or not the currently received query has violated the privacy requirement with respect to all previous queries. One limitation of any interactive privacy-preserving query system is that it can only answer a sublinear number of queries in total; otherwise, an adversary (or a group of corrupted data recipients) will be able to reconstruct all but 1 . o(1) fraction of the original data, which is a very strong violation of privacy. When the maximum number of queries is reached, the query service must be closed to avoid privacy leak. In the case of the non-interactive query model, the adversary can issue only one query and, therefore, the non-interactive query model cannot achieve the same degree of privacy defined by Introduction the interactive model. One may consider that privacy-reserving data publishing is a special case of the non-interactivequery model.This paper presents a survey for most of the common attacks techniques for anonymization-based PPDM & PPDP and explains their effects on Data Privacy. k-anonymity is used for security of respondents identity and decreases linking attack in the case of homogeneity attack a simple k-anonymity model fails and we need a concept which prevent from this attack solution is l-diversity. All tuples are arranged in well represented form and adversary will divert to l places or on l sensitive attributes. l-diversity limits in case of background knowledge attack because no one predicts knowledge level of an adversary. It is observe that using generalization and suppression we also apply these techniques on those attributes which doesn’t need th is extent of privacy and this leads to reduce the precision of publishing table. e-NSTAM (extended Sensitive Tuples Anonymity Method) is applied on sensitive tuples only and reduces information loss, this method also fails in the case of multiple sensitive tuples.Generalization with suppression is also the causes of data lose because suppression emphasize on not releasing values which are not suited for k factor. Future works in this front can include defining a new privacy measure along with l-diversity for multiple sensitive attribute and we will focus to generalize attributes without suppression using other techniques which are used to achieve k-anonymity because suppression leads to reduce the precision ofpublishing table.译文:数据挖掘和数据发布数据挖掘中提取出大量有趣的模式从大量的数据或知识。
大数据应用的英文作文
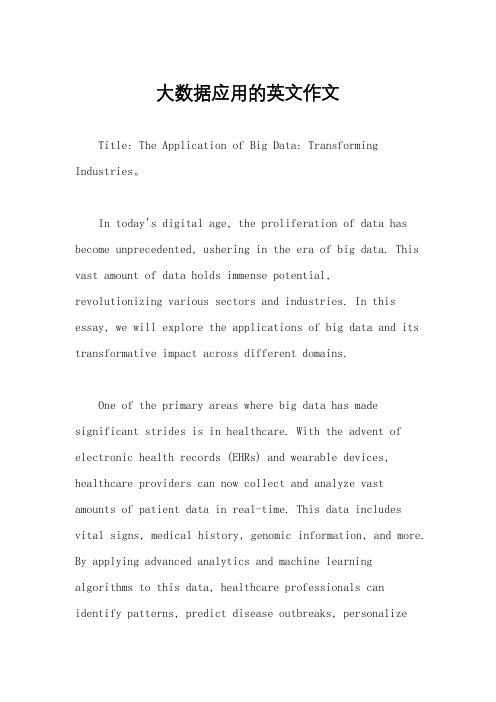
大数据应用的英文作文Title: The Application of Big Data: Transforming Industries。
In today's digital age, the proliferation of data has become unprecedented, ushering in the era of big data. This vast amount of data holds immense potential,revolutionizing various sectors and industries. In this essay, we will explore the applications of big data and its transformative impact across different domains.One of the primary areas where big data has made significant strides is in healthcare. With the advent of electronic health records (EHRs) and wearable devices, healthcare providers can now collect and analyze vast amounts of patient data in real-time. This data includesvital signs, medical history, genomic information, and more. By applying advanced analytics and machine learning algorithms to this data, healthcare professionals canidentify patterns, predict disease outbreaks, personalizetreatments, and improve overall patient care. For example, predictive analytics can help identify patients at risk of developing chronic conditions such as diabetes or heart disease, allowing for proactive interventions to prevent or mitigate these conditions.Another sector that has been transformed by big data is finance. In the financial industry, data-driven algorithms are used for risk assessment, fraud detection, algorithmic trading, and customer relationship management. By analyzing large volumes of financial transactions, market trends, and customer behavior, financial institutions can make more informed decisions, optimize investment strategies, and enhance the customer experience. For instance, banks employ machine learning algorithms to detect suspicious activities and prevent fraudulent transactions in real-time, safeguarding both the institution and its customers.Furthermore, big data has revolutionized the retail sector, empowering companies to gain deeper insights into consumer preferences, shopping behaviors, and market trends. Through the analysis of customer transactions, browsinghistory, social media interactions, and demographic data, retailers can personalize marketing campaigns, optimize pricing strategies, and enhance inventory management. For example, e-commerce platforms utilize recommendation systems powered by machine learning algorithms to suggest products based on past purchases and browsing behavior, thereby improving customer engagement and driving sales.The transportation industry is also undergoing a profound transformation fueled by big data. With the proliferation of GPS-enabled devices, sensors, andtelematics systems, transportation companies can collect vast amounts of data on vehicle performance, traffic patterns, weather conditions, and logistics operations. By leveraging this data, companies can optimize route planning, reduce fuel consumption, minimize delivery times, and enhance overall operational efficiency. For instance, ride-sharing platforms use predictive analytics to forecast demand, allocate drivers more effectively, and optimizeride routes, resulting in improved service quality and customer satisfaction.In addition to these sectors, big data is making significant strides in fields such as manufacturing, agriculture, energy, and government. In manufacturing, data analytics is used for predictive maintenance, quality control, and supply chain optimization. In agriculture, precision farming techniques enabled by big data help optimize crop yields, minimize resource usage, and mitigate environmental impact. In energy, smart grid technologies leverage big data analytics to optimize energy distribution, improve grid reliability, and promote energy efficiency. In government, big data is utilized for urban planning, public safety, healthcare management, and policy formulation.In conclusion, the application of big data is transforming industries across the globe, enabling organizations to make data-driven decisions, unlock new insights, and drive innovation. From healthcare and finance to retail and transportation, the impact of big data is profound and far-reaching. As we continue to harness the power of data analytics and machine learning, we can expect further advancements and breakthroughs that will shape the future of our society and economy.。
客户关系管理和大数据外文文献翻译
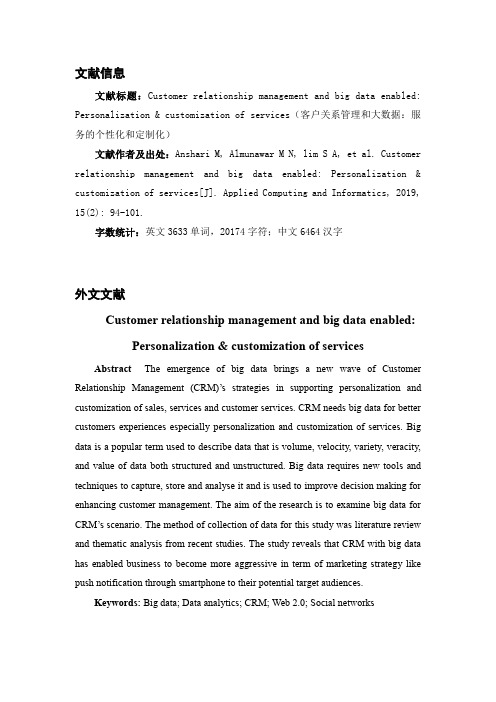
文献信息文献标题:Customer relationship management and big data enabled: Personalization & customization of services(客户关系管理和大数据:服务的个性化和定制化)文献作者及出处:Anshari M, Almunawar M N, lim S A, et al. Customer relationship management and big data enabled: Personalization & customization of services[J]. Applied Computing and Informatics, 2019, 15(2): 94-101.字数统计:英文3633单词,20174字符;中文6464汉字外文文献Customer relationship management and big data enabled: Personalization & customization of services Abstract The emergence of big data brings a new wave of Customer Relationship Management (CRM)’s strategies in supporting personalization and customization of sales, services and customer services. CRM needs big data for better customers experiences especially personalization and customization of services. Big data is a popular term used to describe data that is volume, velocity, variety, veracity, and value of data both structured and unstructured. Big data requires new tools and techniques to capture, store and analyse it and is used to improve decision making for enhancing customer management. The aim of the research is to examine big data for CRM’s scenario. The method of collection of data for this study was literature review and thematic analysis from recent studies. The study reveals that CRM with big data has enabled business to become more aggressive in term of marketing strategy like push notification through smartphone to their potential target audiences.Keywords: Big data; Data analytics; CRM; Web 2.0; Social networks1.IntroductionManaging good customer relationship in an organization refers to the concepts, tools, and strategies of customer relationship management (CRM). CRM as a tools with Web/Apps technology provides organizations ability to understand customers or potential customers its usual practices and thus deliver a particular activities that might convince them to make transactions and decisions. CRM has been discussed in many fields such as business, health care, science, and other service industries. The massive adoption of big data in any sectors has triggered assessment of frontend perspective especially managing customer relationship. It is pivotal to examine the role of big data within CRM strategies.Big data have quantum leap to a digital era where public generates a huge data in any sectors and industries. The amount of data are captured, collected, and processed by organization through digital sensors, communications, computation, and storage had captured information which was valuable to businesses, sciences, government, and society at large. A large amount of data streaming from smartphones, computers, parking meters, buses, trains, and supermarkets. Search engine companies collect enormous amount of data per day and share these data to useful information for others as well as their own used.Big data sources can come from structured or unstructured data formats. These data sources are gathered from multi channels like social networks, voice recording, image processing, video recording, open government data (OGD), and online customers’ activities. Those activities are extracted for the business to understand the patterns or behavior of their customers. Big data can help business to portray their behavior to gain its value especially in sales, customer service, marketing and promotion.Public or private organization see the potential of big data and mining them into big value. Many organizations have made huge investments to collect, integrate, analyse data, and use it to run business activities. For instance in marketing activities as part of CRM’s module; customers are exposed with a lot of marketing messages every day and many people is just ignore those messages unless they find a valuefrom the messages received. Email campaigning program are distributed to public or random customers about their new product so that customers might be interested to have one. Email campaigning may turn into disappointing situation because customers feel bombarded with the spam and lead to increase number of unsubscribes. Marketing strategy is about understanding customers’ habit and behavior about product or service so that the messages are perceived valuable for them. Unfortunately, many organizations may simplify marketing strategies by focusing a short term relationship with their customers with no path in attracting, retaining, and extending for long term relationship. Therefore, there is a need for personalization and customization of marketing that fits for each and every potential customer.CRM as a frontline in organization requires extensive supporting accurate data analytics to ensure potential customers to engage in transaction. Since customers make buying decisions every day and every decision depends on consideration of cost, benefits, and value. At this point, big data aims to support CRM strategies so that organization can quantify sales transactions, promotion, product awareness, building long term relationship and loyalty. Furthermore, the paper address the following question: How can big data in CRM will enhance CRM strategies in delivering personalization and customization of services for customer? The structure of this study is organized as follows. In the next section, a literature review of related work. Section 3 explains the methodology and results of our study. Section 4 presents a discussion of our findings. Recommendations for suggested future research directions are presented in Section 5, and Section 6 concludes the paper.2.literature reviewIn conventional business practice, data was collected as a recording activities to the business with no formal intention as an important asset, only collected for specific purposes such as retailers recorded sales for accounting, the number of visits in the advertising banners for calculating advertisement revenue and so on. Since many organizations either privates or publics have realized the value of data gathered as an asset, data no longer treated as its initial purpose. With the capabilities of processinghuge amount of data, it has created a new industry of data analytic services. For example IBM and Twitter involved partnership on data analytics for the purpose of selling analytical information to corporate clients in order to provide businesses a real-time conversations to make smarter decision. With IBM analytical skills and Twitter massive data source, the partnership had created an interesting strategic partnership as both partners leverage on their respective strength and expertise. Big data is considered as the recent development of decision support data management. Big data have big impact towards businesses ranging from CRM, ERP, and SCM. In the next section is discussed recent literatures on CRM and big data.2.1.Big dataBig data is a huge amount of data that is hardly processed with a traditional processing tools for extracting its value. It has an impact in various fields like business, healthcare, financial, security, communication, agriculture, and even traffic control. Big data creates opportunities for business that can use it for generating business value. The purpose is intended to gain value from volumes and a variety of data by allowing velocity of analysis. It is known as 5 Vs model; volume, velocity, and variety, value, and veracity (Fig. 1). V olume means processing massive data scale from any data type gathered. The explosive of data volumes improve a knowledge sharing and people awareness. Big data is a particularly massive volume with a large data sets, and those data cannot be analysed its content using traditional database tools, management, and processing. Velocity means real time data processing, specifically data collection and analysis. Velocity processes very large data in real-time processing. In addition, big data escalates its speed velocity surpassing that of old methods of computing. Variety is any types of data from various channels including structured and unstructured data like audio, video, image, location data for example Google Map, webpage, and text, as well as traditional structured data. Some of the semistructured data based can use Hadoop. It focuses on analysing volumes of data involved and mining the data and calculations involved in large amount of computing. Finally, veracity refers to data authenticity with the interest in the data source of Web log files, social media, enterprise content, transaction, data application. Date need a validpower of information to ensure its authenticity and safety.Fig. 1. Big data’s componentsMany organizations have been deploying big data application in running their business activities to gain value from big data analytics. Value is generated from big data processing that supports the right decision. Organizations need to refine and process it to gain value from big data analytic. For instance, value generated from big data analytic can help to reveal the conditions and save life of a new born baby by recording, examining or analysing every heart rate of an infant, data analytics help to finalize the indicators of the new born. One of the applications on the use of big data is to optimize machine or device performance. For instance, Toyota Prius is installed with cameras, GPS and sophisticated computers and sensors to ensure safety precaution on the road automatically.Big data also reduces the maintenance costs for instance, organizations deploy cloud computing approach where data are stored in the cloud. The emergence of cloud computing has enabled big data analytics to be cost efficient, easily accessed, and reliable. Cloud computing is robust, reliable and responsive when there are issues because it is responsible of cloud service provider. Since, service outrages are unacceptable at the business. Whenever data analytic goes down impacting marketingactivities are disrupted and customers have to question whether to trust such a system. Therefore reliability is competitive advantage of cloud computing in big data application.In addition, businesses have aggressively built their organization on big data capabilities. Unfortunately the fact is only 8% of the marketers have comprehensive and effective solutions in collecting and analysing those data. Evans Data Corporation conducted survey of big data and advanced analytics in organization (Fig. 2). Customer-cantered departments like as marketing, sales, and customer service are dominant users for 38.2% of all big data and advanced analytical apps. While, marketing department has the most common users (14.4%) of the data analytics, followed by IT (13.3%), and research for 13% (Columbus, 2015).Fig. 2. Big data analytics usage in organization. Sources: Evans Data Corporation2.2.Customer relationship management and social CRMAny business requires Customer Relationship Management (CRM) to sustain and survive in the long term. CRM is a tool and strategy for managing customers’ interaction using technology to automate business processes. CRM consists of sales, marketing, and customer service activities (Fig. 3). The aims are to find, attract newcustomers, nurture and retain them for future business. Business uses CRM in meeting customers’ expectations and aligning with the organization’s mission and objectives in order to bring about a sustainable performance and effective customer relationships.Fig. 3. CRM scope & moduleThe emergence of Web 2.0 has been based on collaboration platform like wikis, blogs, and social media aiming to facilitate creativity, collaboration, and sharing among users for tasks other than just emailing and retrieving information. The concept of a social network defines an organization as a system that contains objects such as people, groups, and other organizations linked together by a range of relationships. Web 2.0 is a tool that can be used to communicate a political agenda to the public via social networks. Users can gain access to the data on Web 2.0 enabled sites and exercise control over such data. Web 2.0 represents a revolution in how people communicate facilitating peer-to-peer collaboration and easy access to real-time communication. The rapid growth in Web 2.0 has impacted organization that cannot their customer relationship by using traditional CRM techniques. Social CRM is a recent approach and strategies to reveal patterns in customer management, behavior, or anything related to the multi channels customers’ interactions as expressed at Fig. 4. Social CRM makes more precise analysis possible based on people conversation in social media, and thus helps them to provide more accurate programs or activities leading to customers’ interests and preferences.Fig. 4. CRM 1.0 vs CRM 2.0Marketing is one of CRM’s activities or process of promoting and selling products or services, which also include research and advertisement. Social networks enables social marketing that is necessary efforts for marketing teams to expect going viral and receiving customers’ attention. ‘‘Marketing, is defined an the activity, set of institutions, and processes for creating, communicating, delivering, and exchanging offerings that have value for customers, clients, partners, and society at large.”. Marketing should focus on building relationships and meanings. It also applies to sales and customer services where organizations use social networks as a tool to make sales as much as possible of handling customers’ complaint at social media. Since social networks is part of big data source, the next question, how big data will impact CRM strategies.Social media has empowered customers to make conversation and business organization may utilize an increasing amount of data through people conversations that is available to them for company’s benefits such as understanding customer preference, complaining items, people expectations. Web 2.0 platform allows customers to express their opinions. In the context of CRM, social networks provide a means of strengthening relationships between customers and service providers. Itmight be utilized to create long-term relationships between business organizations and their customers and public in general. Adopting social networks into CRM is known as Social CRM or a second generation of CRM (CRM 2.0) that empowers customers to express their opinions and expectations about product or services. Social CRM has become ‘a must’ strategies for any organization nowadays to understand their customers better. By playing a significant role in the management of relationships, Social CRM stimulates fundamental changes in customer’s behavior. Social CRM has an impact towards multi channels relationships in all areas either public or private sectors is no exception.3.MethodThe study investigates the factors that an organization considers to adopt big data. The objective of the study is to investigate recent big data adoption in an organization. The methods consisted of in-depth analysis of the latest research on big data in business organization. The data for this report was through literature review of articles ranging from 2010 to 2015. The reason for choosing this time period because of the velocity of big data, any older articles might have irrelevant information. Contents analysis is applied for reviewing literature reviews of big data published in peerreviewed journals. The review process then is clustered into a thematic. We enhance and integrate various possible solutions into proposed model. We chose only English-language articles published in peer-reviewed journals. After removing duplicates and articles beyond the scope of this study, these articles were reviewed to extract feature of CRM and big data capabilities at Fig. 5.Fig. 5. Big data and marketing4.DiscussionBusiness realizes that their most valuable assets are relationships with customers and all stakeholders. In fact, building personal and social relationships become important area in marketing. The importance of relationships as market based assets that contribute to customers’ value. With the amount of data increase, some business organizations use advanced powerful computers with a huge storage to process big data analytics and to increase their performance resulting in tremendous cost saving. Businesses manage structured and unstructured data sources such as social marketing, retail databases, recorded customer activity, logistics, and enterprise data to establish a quality level of CRM strategies by having the abilities or knowledge on how to recognize big data and its advantage. While, big data analytics is a process to reveal the variety of data types in big data itself. There are some CRM strategies that can happen through big data and big data analytics.Since big data can provide a pattern of customers’ information, businesses can predict and assume what are the needs of their customers nowadays. Fig. 5 indicates basic framework on how big data can contribute to generating CRM strategy. Big data had helped shaped many industries and changed the way businesses operatednowadays. Big companies definitely benefited from this shift especially companies such as technology giants such as Amazon and googles and would continue to serve these giants from the sheer volume of data they generated. Data Velocity showed how marketers could have access to real-time data, for example real time analytics of interactions on internet sites and also social media interactions.CRM with the big data influence, a new paradigm had been created to allow accessibility and availability of information which result in greater take up by big or small business alike. Big data offers pervasive knowledge acquisition in CRM activities. Big data will support long-term relationship through understanding customers’ life cycle and behavior in more comprehensive perspective. Customers voluntarily generate a huge amount of data daily by detailing their interest and preference about products or services to the public through various channels. Therefore, big data analytic can come up with a comprehensive views of customers so that organization can enhance service fitting with customer attention, engagement, participation, and personalization. The study introduces several fundamental concept of marketing with big data that are closely related to customer based CRM strategies in an organization by engaging customer life cycle.CRM with big data brings a promise of big transformation that can affect organization in delivering CRM strategies. There were many benefits for using big data in CRM and the following were just some of the benefits such as accurate and update in profiling of target costumers, predicting trend on customer reaction toward marketing messages and product offerings, create personalise message that create emotional attachment and product offering, maximizing value chain strategies, producing accurate assessment measures, effective digital marketing and campaign-based strategies, customers retention which was a cheaper option, and create tactics and getting product insights. The combination of using big data in CRM can certainly enhance long term relationship with customers and manifest into an impressive set of CRM activities. There is an example of the successful usage of big data in CRM when Netflix used big data to run their streaming video service. Instead of using traditional methods of data gathering, they were able to find out what theircustomers want and made measurable marketing decisions. Big data can perform better CRM strategies than any processes with double the speed.CRM with big data features becomes more aggressive in term of marketing strategy like push notification through smartphone to the potential target audiences. Web / Apps users who make comment, liking page, or comes back visiting Web or Apps are potential customers are targeted for pushed notification. Technically, there are many third parties for Apps or Web that can help business to set up push notification right to the users. For instance, there are also many plugin supports web push facilities in CMS based website. Notification can be set up auto generated or manual whenever new contents are available directed at customer convenience in the form of text message, link sharing, or smartphone notification offering promotion at nearby shop. CRM aims to quantify sales transactions, promotion, product awareness, while its strategies for building long term relationship and loyalty. Businesses cannot simplify marketing strategies only focusing a short term relationship with customers without any path in attracting, retaining, and extending for long term relationship.In addition, the organization can also create better customer personas by using the profile data as the backbone of creating accurately personifications for the customers. Also the organization will have data on what the customers’ needs and preferences and used this data to provide better content for the audience where the content is relevant and valuable to them. All these data can also provide valuable information for the management team to improve marketing budget management by ensuring business operational process stayed on budget with the help of data and to be more focused and targeted.5.ChallengesBig data in CRM has very much potential to offer, with its ability to collect and produce a big amounts of data, big data could really be the downfall as well without the proper expertise and tools to obtain and analysed them. Many challenges must be managed before these potential can be fully optimized. Firstly, it may occur when organizations are shortage in technical supports and expertise. Secondly, it is difficultto track customer behavior especially trailing customers moving from brand awareness to conversion. It challenges to connect the dot from online to offline channels such as when and where customer see or read about a product to finally purchasing the product. Thirdly, CRM with big data may need more user friendly data analytics tools in producing report especially when it comes to utilizing the data appropriately across the channels, especially when they do not understand the effectiveness of their efforts in the process. There is no one size fit all solution, staffs need to integrate big data into their strategies, especially products lines, and content offering and customer journey is unique. Until such tools is available many CRM staffs would continue to search for solutions to overcome this challenge. The last challenge refers to data authenticity with the interest in the data source of Web log files, social media, enterprise content, transaction, data application may need a valid power of information to ensure its authenticity and safety. For examples, all the post or tweets we post on social networks are observed by the one who manages the big data. Finally, there is a possibility that the research may lack of generalizability because it requires case study and primary data collection from the business organizations, this research will plan to reach a large number of participants in the future.6.ConclusionCRM is about understanding of human behavior and interests. Big data can be expected to improve customer relationship as it allows interactivity, multi-way communications, personalization, and customization. The recent developments of big data analytics have optimized process, growth, and generate aggressive marketing strategy and delivering value for each customer and potential customer. CRM with big data enabled engage customers in delivering affective CRM activities where marketing teams at the organizations tune the ideas into executable marketing program. Big data enhance CRM strategies by understanding better customers’ habits and behaviors so that business can deliver CRM be more personalized and customized for each and every customers. Finally, CRM with big data will make better tools andstrategies more personalized and customized to the customers because they understand well target audiences and intended message to send.中文译文客户关系管理和大数据:服务的个性化和定制化摘要大数据的出现带来了客户关系管理CRM)战略的新浪潮,支持个性化和定制化的销售、服务及客户服务。
大数据文献综述英文版

The development and tendency of Big DataAbstract: "Big Data" is the most popular IT word after the "Internet of things" and "Cloud computing". From the source, development, status quo and tendency of big data, we can understand every aspect of it. Big data is one of the most important technologies around the world and every country has their own way to develop the technology.Key words: big data; IT; technology1 The source of big dataDespite the famous futurist Toffler propose the conception of “Big Data” in 1980, for a long time, because the primary stage is still in the development of IT industry and uses of information sources, “Big Data” is not get enough attention by the people in that age[1].2 The development of big dataUntil the financial crisis in 2008 force the IBM ( multi-national corporation of IT industry) proposing conception of “Smart City”and vigorously promote Internet of Things and Cloud computing so that information data has been in a massive growth meanwhile the need for the technology is very urgent. Under this condition, some American data processing companies have focused on developing large-scale concurrent processing system, then the “Big Data”technology become available sooner and Hadoop mass data concurrent processing system has received wide attention. Since 2010, IT giants have proposed their products in big data area. Big companies such as EMC、HP、IBM、Microsoft all purchase other manufacturer relating to big data in order to achieve technical integration[1]. Based on this, we can learn how important the big data strategy is. Development of big data thanks to some big IT companies such as Google、Amazon、China mobile、Alibaba and so on, because they need a optimization way to store and analysis data. Besides, there are also demands of health systems、geographic space remote sensing and digital media[2].3 The status quo of big dataNowadays America is in the lead of big data technology and market application. USA federal government announced a “Big Data’s research and development” plan in March,2012, which involved six federal government department the National Science Foundation, Health Research Institute, Department of Energy, Department of Defense, Advanced Research Projects Agency and Geological Survey in order to improve the ability to extract information and viewpoint of big data[1]. Thus, it can speed science and engineering discovery up, and it is a major move to push some research institutions making innovations.The federal government put big data development into a strategy place, which hasa big impact on every country. At present, many big European institutions is still at the primary stage to use big data and seriously lack technology about big data. Most improvements and technology of big data are come from America. Therefore, there are kind of challenges of Europe to keep in step with the development of big data. But, in the financial service industry especially investment banking in London is one of the earliest industries in Europe. The experiment and technology of big data is as good as the giant institution of America. And, the investment of big data has been maintained promising efforts. January 2013, British government announced 1.89 million pound will be invested in big data and calculation of energy saving technology in earth observation and health care[3].Japanese government timely takes the challenge of big data strategy. July 2013, Japan’s communications ministry proposed a synthesize strategy called “Energy ICT of Japan” which focused on big data application. June 2013, the abe cabinet formally announced the new IT strategy----“The announcement of creating the most advanced IT country”. This announcement comprehensively expounded that Japanese new IT national strategy is with the core of developing opening public data and big data in 2013 to 2020[4].Big data has also drawn attention of China government.《Guiding opinions of the State Council on promoting the healthy and orderly development of the Internet of things》promote to quicken the core technology including sensor network、intelligent terminal、big data processing、intelligent analysis and service integration. December 2012, the national development and reform commission add data analysis software into special guide, in the beginning of 2013 ministry of science and technology announced that big data research is one of the most important content of “973 program”[1]. This program requests that we need to research the expression, measure and semantic understanding of multi-source heterogeneous data, research modeling theory and computational model, promote hardware and software system architecture by energy optimal distributed storage and processing, analysis the relationship of complexity、calculability and treatment efficiency[1]. Above all, we can provide theory evidence for setting up scientific system of big data.4 The tendency of big data4.1 See the future by big dataIn the beginning of 2008, Alibaba found that the whole number of sellers were on a slippery slope by mining analyzing user-behavior data meanwhile the procurement to Europe and America was also glide. They accurately predicting the trend of world economic trade unfold half year earlier so they avoid the financial crisis[2]. Document [3] cite an example which turned out can predict a cholera one year earlier by mining and analysis the data of storm, drought and other natural disaster[3].4.2 Great changes and business opportunitiesWith the approval of big data values, giants of every industry all spend more money in big data industry. Then great changes and business opportunity comes[4].In hardware industry, big data are facing the challenges of manage, storage and real-time analysis. Big data will have an important impact of chip and storage industry,besides, some new industry will be created because of big data[4].In software and service area, the urgent demand of fast data processing will bring great boom to data mining and business intelligence industry.The hidden value of big data can create a lot of new companies, new products, new technology and new projects[2].4.3 Development direction of big dataThe storage technology of big data is relational database at primary. But due to the canonical design, friendly query language, efficient ability dealing with online affair, Big data dominate the market a long term. However, its strict design pattern, it ensures consistency to give up function, its poor expansibility these problems are exposed in big data analysis. Then, NoSQL data storage model and Bigtable propsed by Google start to be in fashion[5].Big data analysis technology which uses MapReduce technological frame proposed by Google is used to deal with large scale concurrent batch transaction. Using file system to store unstructured data is not lost function but also win the expansilility. Later, there are big data analysis platform like HA VEn proposed by HP and Fusion Insight proposed by Huawei . Beyond doubt, this situation will be continued, new technology and measures will come out such as next generation data warehouse, Hadoop distribute and so on[6].ConclusionThis paper we analysis the development and tendency of big data. Based on this, we know that the big data is still at a primary stage, there are too many problems need to deal with. But the commercial value and market value of big data are the direction of development to information age.忽略此处..[1] Li Chunwei, Development report of China’s E-Commerce enterprises, Beijing , 2013,pp.268-270[2] Li Fen, Zhu Zhixiang, Liu Shenghui, The development status and the problems of large data, Journal of Xi’an University of Posts and Telecommunications, 18 volume, pp. 102-103,sep.2013 [3] Kira Radinsky, Eric Horivtz, Mining the Web to Predict Future Events[C]//Proceedings of the 6th ACM International Conference on Web Search and Data Mining, WSDM 2013: New York: Association for Computing Machinery,2013,pp.255-264[4] Chapman A, Allen M D, Blaustein B. It’s About the Data: Provenance as a Toll for Assessing Data Fitness[C]//Proc of the 4th USENIX Workshop on the Theory and Practice of Provenance, Berkely, CA: USENIX Association, 2012:8[5] Li Ruiqin, Zheng Janguo, Big data Research: Status quo, Problems and Tendency[J],Network Application,Shanghai,1994,pp.107-108[6] Meng Xiaofeng, Wang Huiju, Du Xiaoyong, Big Daya Analysis: Competition and Survival of RDBMS and ManReduce[J], Journal of software, 2012,23(1): 32-45。
大数据挖掘外文翻译文献
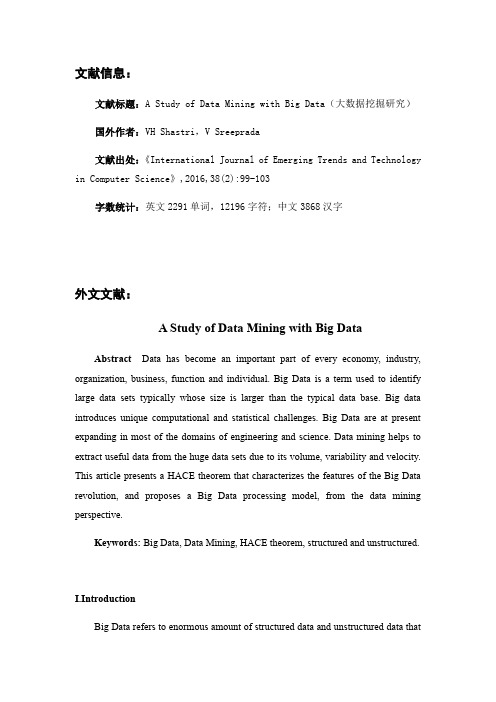
文献信息:文献标题:A Study of Data Mining with Big Data(大数据挖掘研究)国外作者:VH Shastri,V Sreeprada文献出处:《International Journal of Emerging Trends and Technology in Computer Science》,2016,38(2):99-103字数统计:英文2291单词,12196字符;中文3868汉字外文文献:A Study of Data Mining with Big DataAbstract Data has become an important part of every economy, industry, organization, business, function and individual. Big Data is a term used to identify large data sets typically whose size is larger than the typical data base. Big data introduces unique computational and statistical challenges. Big Data are at present expanding in most of the domains of engineering and science. Data mining helps to extract useful data from the huge data sets due to its volume, variability and velocity. This article presents a HACE theorem that characterizes the features of the Big Data revolution, and proposes a Big Data processing model, from the data mining perspective.Keywords: Big Data, Data Mining, HACE theorem, structured and unstructured.I.IntroductionBig Data refers to enormous amount of structured data and unstructured data thatoverflow the organization. If this data is properly used, it can lead to meaningful information. Big data includes a large number of data which requires a lot of processing in real time. It provides a room to discover new values, to understand in-depth knowledge from hidden values and provide a space to manage the data effectively. A database is an organized collection of logically related data which can be easily managed, updated and accessed. Data mining is a process discovering interesting knowledge such as associations, patterns, changes, anomalies and significant structures from large amount of data stored in the databases or other repositories.Big Data includes 3 V’s as its characteristics. They are volume, velocity and variety. V olume means the amount of data generated every second. The data is in state of rest. It is also known for its scale characteristics. Velocity is the speed with which the data is generated. It should have high speed data. The data generated from social media is an example. Variety means different types of data can be taken such as audio, video or documents. It can be numerals, images, time series, arrays etc.Data Mining analyses the data from different perspectives and summarizing it into useful information that can be used for business solutions and predicting the future trends. Data mining (DM), also called Knowledge Discovery in Databases (KDD) or Knowledge Discovery and Data Mining, is the process of searching large volumes of data automatically for patterns such as association rules. It applies many computational techniques from statistics, information retrieval, machine learning and pattern recognition. Data mining extract only required patterns from the database in a short time span. Based on the type of patterns to be mined, data mining tasks can be classified into summarization, classification, clustering, association and trends analysis.Big Data is expanding in all domains including science and engineering fields including physical, biological and biomedical sciences.II.BIG DATA with DATA MININGGenerally big data refers to a collection of large volumes of data and these data are generated from various sources like internet, social-media, business organization, sensors etc. We can extract some useful information with the help of Data Mining. It is a technique for discovering patterns as well as descriptive, understandable, models from a large scale of data.V olume is the size of the data which is larger than petabytes and terabytes. The scale and rise of size makes it difficult to store and analyse using traditional tools. Big Data should be used to mine large amounts of data within the predefined period of time. Traditional database systems were designed to address small amounts of data which were structured and consistent, whereas Big Data includes wide variety of data such as geospatial data, audio, video, unstructured text and so on.Big Data mining refers to the activity of going through big data sets to look for relevant information. To process large volumes of data from different sources quickly, Hadoop is used. Hadoop is a free, Java-based programming framework that supports the processing of large data sets in a distributed computing environment. Its distributed supports fast data transfer rates among nodes and allows the system to continue operating uninterrupted at times of node failure. It runs Map Reduce for distributed data processing and is works with structured and unstructured data.III.BIG DATA characteristics- HACE THEOREM.We have large volume of heterogeneous data. There exists a complex relationship among the data. We need to discover useful information from this voluminous data.Let us imagine a scenario in which the blind people are asked to draw elephant. The information collected by each blind people may think the trunk as wall, leg as tree, body as wall and tail as rope. The blind men can exchange information with each other.Figure1: Blind men and the giant elephantSome of the characteristics that include are:i.Vast data with heterogeneous and diverse sources: One of the fundamental characteristics of big data is the large volume of data represented by heterogeneous and diverse dimensions. For example in the biomedical world, a single human being is represented as name, age, gender, family history etc., For X-ray and CT scan images and videos are used. Heterogeneity refers to the different types of representations of same individual and diverse refers to the variety of features to represent single information.ii.Autonomous with distributed and de-centralized control: the sources are autonomous, i.e., automatically generated; it generates information without any centralized control. We can compare it with World Wide Web (WWW) where each server provides a certain amount of information without depending on other servers.plex and evolving relationships: As the size of the data becomes infinitely large, the relationship that exists is also large. In early stages, when data is small, there is no complexity in relationships among the data. Data generated from social media and other sources have complex relationships.IV.TOOLS:OPEN SOURCE REVOLUTIONLarge companies such as Facebook, Yahoo, Twitter, LinkedIn benefit and contribute work on open source projects. In Big Data Mining, there are many open source initiatives. The most popular of them are:Apache Mahout:Scalable machine learning and data mining open source software based mainly in Hadoop. It has implementations of a wide range of machine learning and data mining algorithms: clustering, classification, collaborative filtering and frequent patternmining.R: open source programming language and software environment designed for statistical computing and visualization. R was designed by Ross Ihaka and Robert Gentleman at the University of Auckland, New Zealand beginning in 1993 and is used for statistical analysis of very large data sets.MOA: Stream data mining open source software to perform data mining in real time. It has implementations of classification, regression; clustering and frequent item set mining and frequent graph mining. It started as a project of the Machine Learning group of University of Waikato, New Zealand, famous for the WEKA software. The streams framework provides an environment for defining and running stream processes using simple XML based definitions and is able to use MOA, Android and Storm.SAMOA: It is a new upcoming software project for distributed stream mining that will combine S4 and Storm with MOA.Vow pal Wabbit: open source project started at Yahoo! Research and continuing at Microsoft Research to design a fast, scalable, useful learning algorithm. VW is able to learn from terafeature datasets. It can exceed the throughput of any single machine networkinterface when doing linear learning, via parallel learning.V.DATA MINING for BIG DATAData mining is the process by which data is analysed coming from different sources discovers useful information. Data Mining contains several algorithms which fall into 4 categories. They are:1.Association Rule2.Clustering3.Classification4.RegressionAssociation is used to search relationship between variables. It is applied in searching for frequently visited items. In short it establishes relationship among objects. Clustering discovers groups and structures in the data.Classification deals with associating an unknown structure to a known structure. Regression finds a function to model the data.The different data mining algorithms are:Table 1. Classification of AlgorithmsData Mining algorithms can be converted into big map reduce algorithm based on parallel computing basis.Table 2. Differences between Data Mining and Big DataVI.Challenges in BIG DATAMeeting the challenges with BIG Data is difficult. The volume is increasing every day. The velocity is increasing by the internet connected devices. The variety is also expanding and the organizations’ capability to capture and process the data is limited.The following are the challenges in area of Big Data when it is handled:1.Data capture and storage2.Data transmission3.Data curation4.Data analysis5.Data visualizationAccording to, challenges of big data mining are divided into 3 tiers.The first tier is the setup of data mining algorithms. The second tier includesrmation sharing and Data Privacy.2.Domain and Application Knowledge.The third one includes local learning and model fusion for multiple information sources.3.Mining from sparse, uncertain and incomplete data.4.Mining complex and dynamic data.Figure 2: Phases of Big Data ChallengesGenerally mining of data from different data sources is tedious as size of data is larger. Big data is stored at different places and collecting those data will be a tedious task and applying basic data mining algorithms will be an obstacle for it. Next we need to consider the privacy of data. The third case is mining algorithms. When we are applying data mining algorithms to these subsets of data the result may not be that much accurate.VII.Forecast of the futureThere are some challenges that researchers and practitioners will have to deal during the next years:Analytics Architecture:It is not clear yet how an optimal architecture of analytics systems should be to deal with historic data and with real-time data at the same time. An interesting proposal is the Lambda architecture of Nathan Marz. The Lambda Architecture solves the problem of computing arbitrary functions on arbitrary data in real time by decomposing the problem into three layers: the batch layer, theserving layer, and the speed layer. It combines in the same system Hadoop for the batch layer, and Storm for the speed layer. The properties of the system are: robust and fault tolerant, scalable, general, and extensible, allows ad hoc queries, minimal maintenance, and debuggable.Statistical significance: It is important to achieve significant statistical results, and not be fooled by randomness. As Efron explains in his book about Large Scale Inference, it is easy to go wrong with huge data sets and thousands of questions to answer at once.Distributed mining: Many data mining techniques are not trivial to paralyze. To have distributed versions of some methods, a lot of research is needed with practical and theoretical analysis to provide new methods.Time evolving data: Data may be evolving over time, so it is important that the Big Data mining techniques should be able to adapt and in some cases to detect change first. For example, the data stream mining field has very powerful techniques for this task.Compression: Dealing with Big Data, the quantity of space needed to store it is very relevant. There are two main approaches: compression where we don’t loose anything, or sampling where we choose what is thedata that is more representative. Using compression, we may take more time and less space, so we can consider it as a transformation from time to space. Using sampling, we are loosing information, but the gains inspace may be in orders of magnitude. For example Feldman et al use core sets to reduce the complexity of Big Data problems. Core sets are small sets that provably approximate the original data for a given problem. Using merge- reduce the small sets can then be used for solving hard machine learning problems in parallel.Visualization: A main task of Big Data analysis is how to visualize the results. As the data is so big, it is very difficult to find user-friendly visualizations. New techniques, and frameworks to tell and show stories will be needed, as for examplethe photographs, infographics and essays in the beautiful book ”The Human Face of Big Data”.Hidden Big Data: Large quantities of useful data are getting lost since new data is largely untagged and unstructured data. The 2012 IDC studyon Big Data explains that in 2012, 23% (643 exabytes) of the digital universe would be useful for Big Data if tagged and analyzed. However, currently only 3% of the potentially useful data is tagged, and even less is analyzed.VIII.CONCLUSIONThe amounts of data is growing exponentially due to social networking sites, search and retrieval engines, media sharing sites, stock trading sites, news sources and so on. Big Data is becoming the new area for scientific data research and for business applications.Data mining techniques can be applied on big data to acquire some useful information from large datasets. They can be used together to acquire some useful picture from the data.Big Data analysis tools like Map Reduce over Hadoop and HDFS helps organization.中文译文:大数据挖掘研究摘要数据已经成为各个经济、行业、组织、企业、职能和个人的重要组成部分。
大数据应用的参考文献
大数据应用的参考文献以下是关于大数据应用的一些参考文献:1. "Big Data: A Revolution That Will Transform How We Live, Work, and Think" by Viktor Mayer-Schönberger and Kenneth Cukier2. "Hadoop: The Definitive Guide" by Tom White3. "Big Data: A Primer" by Eric Siegel4. "Data Science for Business" by Foster Provost and Tom Fawcett5. "Big Data Analytics: Turning Big Data into Big Money" by Frank J. Ohlhorst6. "The Big Data-Driven Business: How to Use Big Data to Win Customers, Beat Competitors, and Boost Profits" by Russell Glass and Sean Callahan7. "Data-Driven: Creating a Data Culture" by Hilary Mason and DJ Patil8. "Big Data at Work: Dispelling the Myths, Uncovering the Opportunities" by Thomas H. Davenport9. "The Human Face of Big Data" by Rick Smolan and Jennifer Erwitt10. "Big Data: Techniques and Technologies in Geoinformatics"edited by Hassan A. Karimi and Abdulrahman Y. Zekri这些文献包括了关于大数据的定义、技术、应用案例以及商业价值等方面的内容,可以作为深入了解和研究大数据应用的参考资源。
数据分析外文文献+翻译
数据分析外文文献+翻译文献1:《数据分析在企业决策中的应用》该文献探讨了数据分析在企业决策中的重要性和应用。
研究发现,通过数据分析可以获取准确的商业情报,帮助企业更好地理解市场趋势和消费者需求。
通过对大量数据的分析,企业可以发现隐藏的模式和关联,从而制定出更具竞争力的产品和服务策略。
数据分析还可以提供决策支持,帮助企业在不确定的环境下做出明智的决策。
因此,数据分析已成为现代企业成功的关键要素之一。
文献2:《机器研究在数据分析中的应用》该文献探讨了机器研究在数据分析中的应用。
研究发现,机器研究可以帮助企业更高效地分析大量的数据,并从中发现有价值的信息。
机器研究算法可以自动研究和改进,从而帮助企业发现数据中的模式和趋势。
通过机器研究的应用,企业可以更准确地预测市场需求、优化业务流程,并制定更具策略性的决策。
因此,机器研究在数据分析中的应用正逐渐受到企业的关注和采用。
文献3:《数据可视化在数据分析中的应用》该文献探讨了数据可视化在数据分析中的重要性和应用。
研究发现,通过数据可视化可以更直观地呈现复杂的数据关系和趋势。
可视化可以帮助企业更好地理解数据,发现数据中的模式和规律。
数据可视化还可以帮助企业进行数据交互和决策共享,提升决策的效率和准确性。
因此,数据可视化在数据分析中扮演着非常重要的角色。
翻译文献1标题: The Application of Data Analysis in Business Decision-making The Application of Data Analysis in Business Decision-making文献2标题: The Application of Machine Learning in Data Analysis The Application of Machine Learning in Data Analysis文献3标题: The Application of Data Visualization in Data Analysis The Application of Data Visualization in Data Analysis翻译摘要:本文献研究了数据分析在企业决策中的应用,以及机器研究和数据可视化在数据分析中的作用。
大数据英文版
大数据英文版Big Data: Revolutionizing the Way We Analyze and Utilize InformationIntroduction:In this era of digital transformation, the rapid growth of data has become a defining characteristic of our society. Big data refers to the massive volume, velocity, and variety of information that is generated from various sources such as social media, sensors, and online transactions. The ability to effectively analyze and utilize this data has revolutionized industries and transformed the way we make decisions. This article explores the impact of big data, its applications, challenges, and the future prospects of this emerging field.1. The Impact of Big Data:Big data has had a profound impact on various sectors, including business, healthcare, finance, and education. By harnessing the power of data analytics, organizations can gain valuable insights, make informed decisions, and improve their operational efficiency. For instance, retailers can analyze customer purchasing patterns to personalize marketing campaigns and enhance customer satisfaction. In the healthcare sector, big data analytics can be used to predict disease outbreaks, improve patient care, and optimize resource allocation.2. Applications of Big Data:2.1 Business Intelligence:Big data analytics enables organizations to gain a competitive edge by extracting actionable insights from vast amounts of structured and unstructured data. Companies can analyze customer behavior, market trends, and competitor strategies to make data-driven decisions and drive innovation. Moreover, big data analytics can help optimize supply chain management, detect fraud, and improve customer relationship management.2.2 Healthcare:Big data has the potential to revolutionize healthcare by enabling personalized medicine, improving patient outcomes, and reducing costs. By analyzing electronic health records, genomic data, and real-time patient monitoring, healthcare providers can identify patterns, predict diseases, and develop targeted treatment plans. Additionally, big data analytics can enhance clinical research, facilitate drug discovery, and improve healthcare delivery.2.3 Finance:The finance industry heavily relies on big data analytics to detect fraudulent activities, assess creditworthiness, and optimize investment strategies. By analyzing large volumes of financial data, including market trends, customer transactions, and social media sentiment, financial institutions can make more accurate risk assessments and improve their decision-making processes. Furthermore, big data analytics can help identify potential market opportunities and enhance regulatory compliance.2.4 Education:Big data analytics is transforming the education sector by providing insights into student performance, learning patterns, and personalized learning experiences. By analyzing student data, educators can identify at-risk students, tailor instructional approaches, and develop targeted interventions. Moreover, big data analytics can facilitate adaptive learning platforms, improve curriculum design, and enable lifelong learning.3. Challenges of Big Data:While big data offers immense opportunities, it also presents several challenges that need to be addressed:3.1 Data Privacy and Security:The vast amount of data collected raises concerns about privacy and security. Organizations must ensure that data is stored securely, and appropriate measures aretaken to protect sensitive information. Additionally, regulations and policies need to be in place to safeguard individuals' privacy rights.3.2 Data Quality and Integration:Big data comes from various sources and in different formats, making it challenging to ensure data quality and integrate disparate datasets. Data cleansing and integration techniques are essential to ensure accurate and reliable analysis.3.3 Scalability and Infrastructure:The sheer volume and velocity of big data require robust infrastructure and scalable systems to store, process, and analyze the data in a timely manner. Organizations need to invest in advanced technologies and tools to handle the growing demands of big data analytics.4. Future Prospects of Big Data:The future of big data looks promising, with ongoing advancements in technology and increased adoption across industries. The emergence of artificial intelligence and machine learning algorithms will further enhance the capabilities of big data analytics. Additionally, the integration of big data with the Internet of Things (IoT) will generate new opportunities for data-driven decision-making and predictive analytics.Conclusion:Big data has revolutionized the way we analyze and utilize information, enabling organizations to gain valuable insights, make data-driven decisions, and drive innovation. Its applications span across various sectors, including business, healthcare, finance, and education. However, challenges such as data privacy, quality, and infrastructure need to be addressed to fully harness the potential of big data. With ongoing advancements and increased adoption, big data is set to play a pivotal role in shaping the future of industries and society as a whole.。
信息技术发展趋势研究论文中英文外文翻译文献
信息技术发展趋势研究论文中英文外文翻译文献本文旨在通过翻译介绍几篇关于信息技术发展趋势的外文文献,以帮助读者更全面、深入地了解该领域的研究进展。
以下是几篇相关文献的简要介绍:1. 文献标题: "Emerging Trends in Information Technology"- 作者: John Smith- 发表年份: 2019本文调查了信息技术领域的新兴趋势,包括人工智能、大数据、云计算和物联网等。
通过对相关案例的分析,研究人员得出了一些关于这些趋势的结论,并探讨了它们对企业和社会的潜在影响。
2. 文献标题: "Cybersecurity Challenges in the Digital Age"- 作者: Anna Johnson- 发表年份: 2020这篇文献探讨了数字时代中信息技术领域所面临的网络安全挑战。
通过分析日益复杂的网络威胁和攻击方式,研究人员提出了一些应对策略,并讨论了如何提高组织和个人的网络安全防护能力。
3. 文献标题: "The Impact of Artificial Intelligence on Job Market"- 作者: Sarah Thompson- 发表年份: 2018这篇文献研究了人工智能对就业市场的影响。
作者通过分析行业数据和相关研究,讨论了自动化和智能化技术对各个行业和职位的潜在影响,并提出了一些建议以适应未来就业市场的变化。
以上是对几篇外文文献的简要介绍,它们涵盖了信息技术发展趋势的不同方面。
读者可以根据需求进一步查阅这些文献,以获得更深入的了解和研究。
- 1、下载文档前请自行甄别文档内容的完整性,平台不提供额外的编辑、内容补充、找答案等附加服务。
- 2、"仅部分预览"的文档,不可在线预览部分如存在完整性等问题,可反馈申请退款(可完整预览的文档不适用该条件!)。
- 3、如文档侵犯您的权益,请联系客服反馈,我们会尽快为您处理(人工客服工作时间:9:00-18:30)。
大数据外文翻译文献(文档含中英文对照即英文原文和中文翻译)原文:What is Data Mining?Many people treat data mining as a synonym for another popularly used term, “Knowledge Discovery in Databases”, or KDD. Alternatively, others view data mining as simply an essential step in the process of knowledge discovery in databases. Knowledge discovery consists of an iterative sequence of the following steps:· data cleaning: to remove noise or irrelevant data,· data integration: where multiple data sources may be combined,·data selection : where data relevant to the analysis task are retrieved from the database,·data transformation : where data are transformed or consolidated into forms appropriate for mining by performing summary or aggregation operations, for instance,·data mining: an essential process where intelligent methods are applied in order to extract data patterns,·pattern evaluation: to identify the truly interesting patterns representing knowledge based on some interestingness measures, and ·knowledge presentation: where visualization and knowledge representation techniques are used to present the mined knowledge to the user .The data mining step may interact with the user or a knowledge base. The interesting patterns are presented to the user, and may be stored as new knowledge in the knowledge base. Note that according to this view, data mining is only one step in the entire process, albeit an essential one since it uncovers hidden patterns for evaluation.We agree that data mining is a knowledge discovery process. However, in industry, in media, and in the database research milieu, the term “data mining” is becoming more popular than the longer term of “knowledge discovery in databases”. Therefore, in this book, we choose to use the term “data mining”. We adop t a broad view of data mining functionality: data mining is the process of discovering interestingknowledge from large amounts of data stored either in databases, data warehouses, or other information repositories.Based on this view, the architecture of a typical data mining system may have the following major components:1. Database, data warehouse, or other information repository. This is one or a set of databases, data warehouses, spread sheets, or other kinds of information repositories. Data cleaning and data integration techniques may be performed on the data.2. Database or data warehouse server. The database or data warehouse server is responsible for fetching the relevant data, based on the user’s data mining request.3. Knowledge base. This is the domain knowledge that is used to guide the search, or evaluate the interestingness of resulting patterns. Such knowledge can include concept hierarchies, used to organize attributes or attribute values into different levels of abstraction. Knowledge such as user beliefs, which can be used to assess a pattern’s interestingness based on its unexpectedness, may also be included. Other examples of domain knowledge are additional interestingness constraints or thresholds, and metadata (e.g., describing data from multiple heterogeneous sources).4. Data mining engine. This is essential to the data mining system and ideally consists of a set of functional modules for tasks such ascharacterization, association analysis, classification, evolution and deviation analysis.5. Pattern evaluation module. This component typically employs interestingness measures and interacts with the data mining modules so as to focus the search towards interesting patterns. It may access interestingness thresholds stored in the knowledge base. Alternatively, the pattern evaluation module may be integrated with the mining module, depending on the implementation of the data mining method used. For efficient data mining, it is highly recommended to push the evaluation of pattern interestingness as deep as possible into the mining process so as to confine the search to only the interesting patterns.6. Graphical user interface. This module communicates between users and the data mining system, allowing the user to interact with the system by specifying a data mining query or task, providing information to help focus the search, and performing exploratory data mining based on the intermediate data mining results. In addition, this component allows the user to browse database and data warehouse schemas or data structures, evaluate mined patterns, and visualize the patterns in different forms.From a data warehouse perspective, data mining can be viewed as an advanced stage of on-1ine analytical processing (OLAP). However, data mining goes far beyond the narrow scope of summarization-styleanalytical processing of data warehouse systems by incorporating more advanced techniques for data understanding.While there may be many “data mining systems” on the market, not all of them can perform true data mining. A data analysis system that does not handle large amounts of data can at most be categorized as a machine learning system, a statistical data analysis tool, or an experimental system prototype. A system that can only perform data or information retrieval, including finding aggregate values, or that performs deductive query answering in large databases should be more appropriately categorized as either a database system, an information retrieval system, or a deductive database system.Data mining involves an integration of techniques from mult1ple disciplines such as database technology, statistics, machine learning, high performance computing, pattern recognition, neural networks, data visualization, information retrieval, image and signal processing, and spatial data analysis. We adopt a database perspective in our presentation of data mining in this book. That is, emphasis is placed on efficient and scalable data mining techniques for large databases. By performing data mining, interesting knowledge, regularities, or high-level information can be extracted from databases and viewed or browsed from different angles. The discovered knowledge can be applied to decision making, process control, information management, query processing, and so on. Therefore,data mining is considered as one of the most important frontiers in database systems and one of the most promising, new database applications in the information industry.A classification of data mining systemsData mining is an interdisciplinary field, the confluence of a set of disciplines, including database systems, statistics, machine learning, visualization, and information science. Moreover, depending on the data mining approach used, techniques from other disciplines may be applied, such as neural networks, fuzzy and or rough set theory, knowledge representation, inductive logic programming, or high performance computing. Depending on the kinds of data to be mined or on the given data mining application, the data mining system may also integrate techniques from spatial data analysis, Information retrieval, pattern recognition, image analysis, signal processing, computer graphics, Web technology, economics, or psychology.Because of the diversity of disciplines contributing to data mining, data mining research is expected to generate a large variety of data mining systems. Therefore, it is necessary to provide a clear classification of data mining systems. Such a classification may help potential users distinguish data mining systems and identify those that best match their needs. Data mining systems can be categorized according to various criteria, as follows.1) Classification according to the kinds of databases mined.A data mining system can be classified according to the kinds of databases mined. Database systems themselves can be classified according to different criteria (such as data models, or the types of data or applications involved), each of which may require its own data mining technique. Data mining systems can therefore be classified accordingly.For instance, if classifying according to data models, we may have a relational, transactional, object-oriented, object-relational, or data warehouse mining system. If classifying according to the special types of data handled, we may have a spatial, time -series, text, or multimedia data mining system , or a World-Wide Web mining system . Other system types include heterogeneous data mining systems, and legacy data mining systems.2) Classification according to the kinds of knowledge mined.Data mining systems can be categorized according to the kinds of knowledge they mine, i.e., based on data mining functionalities, such as characterization, discrimination, association, classification, clustering, trend and evolution analysis, deviation analysis , similarity analysis, etc.A comprehensive data mining system usually provides multiple and/or integrated data mining functionalities.Moreover, data mining systems can also be distinguished based on the granularity or levels of abstraction of the knowledge mined, includinggeneralized knowledge(at a high level of abstraction), primitive-level knowledge(at a raw data level), or knowledge at multiple levels (considering several levels of abstraction). An advanced data mining system should facilitate the discovery of knowledge at multiple levels of abstraction.3) Classification according to the kinds of techniques utilized.Data mining systems can also be categorized according to the underlying data mining techniques employed. These techniques can be described according to the degree of user interaction involved (e.g., autonomous systems, interactive exploratory systems, query-driven systems), or the methods of data analysis employed(e.g., database-oriented or data warehouse-oriented techniques, machine learning, statistics, visualization, pattern recognition, neural networks, and so on ) .A sophisticated data mining system will often adopt multiple data mining techniques or work out an effective, integrated technique which combines the merits of a few individual approaches.什么是数据挖掘?许多人把数据挖掘视为另一个常用的术语—数据库中的知识发现或KDD的同义词。