Shape Filtering for False Positive Reduction at Computed Tomography Colonography
互联网中充斥着大量的虚假信息作文

互联网中充斥着大量的虚假信息作文英文回答:Internet is flooded with a large amount of false information, which has become a major concern in today's society. The proliferation of fake news, rumors, and misinformation has made it difficult for people to distinguish between what is true and what is not. This issue has serious consequences, as it can lead to misunderstandings, misjudgments, and even conflicts.First and foremost, the spread of false information on the internet has a negative impact on individuals. People may unknowingly believe and share false information, which can mislead others and create a distorted view of reality. For example, a false news article claiming that a certain food can cure a disease may lead people to rely on ineffective treatments, putting their health at risk.Furthermore, the spread of fake news can also havesignificant societal consequences. It can influence public opinion, shape political discourse, and even impactelection outcomes. For instance, during the 2016 US presidential election, there were numerous instances of fake news articles being shared on social media platforms, which influenced voters' perceptions and decisions.In addition, the proliferation of false information on the internet can also have economic implications. Companies and individuals may fall victim to scams and frauds based on false information. For example, a person may receive an email claiming that they have won a lottery and need to provide personal information to claim the prize. If they believe the false information and provide their personal details, they may become victims of identity theft or financial fraud.To address this issue, it is crucial for individuals to develop critical thinking skills and be able to evaluate the credibility of information they come across on the internet. Fact-checking websites and reliable sources should be consulted to verify the accuracy of informationbefore sharing or acting upon it. Additionally, socialmedia platforms and search engines should takeresponsibility in filtering out false information and promoting reliable sources.中文回答:互联网上充斥着大量的虚假信息,这已经成为当今社会的一个重大问题。
RED EYE FALSE POSITIVE FILTERING USING FACE LOCAT
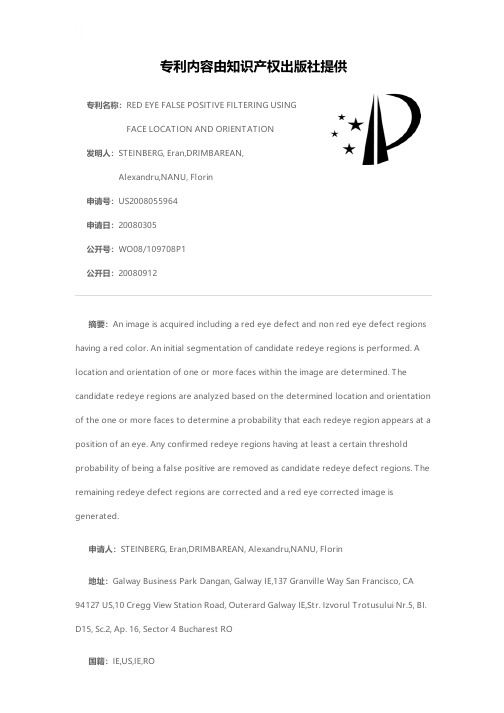
专利名称:RED EYE FALSE POSITIVE FILTERING USING FACE LOCATION AND ORIENTATION发明人:STEINBERG, Eran,DRIMBAREAN,Alexandru,NANU, Florin申请号:US2008055964申请日:20080305公开号:WO08/109708P1公开日:20080912专利内容由知识产权出版社提供摘要:An image is acquired including a red eye defect and non red eye defect regions having a red color. An initial segmentation of candidate redeye regions is performed. A location and orientation of one or more faces within the image are determined. The candidate redeye regions are analyzed based on the determined location and orientation of the one or more faces to determine a probability that each redeye region appears at a position of an eye. Any confirmed redeye regions having at least a certain threshold probability of being a false positive are removed as candidate redeye defect regions. The remaining redeye defect regions are corrected and a red eye corrected image is generated.申请人:STEINBERG, Eran,DRIMBAREAN, Alexandru,NANU, Florin地址:Galway Business Park Dangan, Galway IE,137 Granville Way San Francisco, CA 94127 US,10 Cregg View Station Road, Outerard Galway IE,Str. Izvorul Trotusului Nr.5, BI. D15, Sc.2, Ap. 16, Sector 4 Bucharest RO国籍:IE,US,IE,RO代理机构:SMITH, Andrew, V 更多信息请下载全文后查看。
AI专用词汇
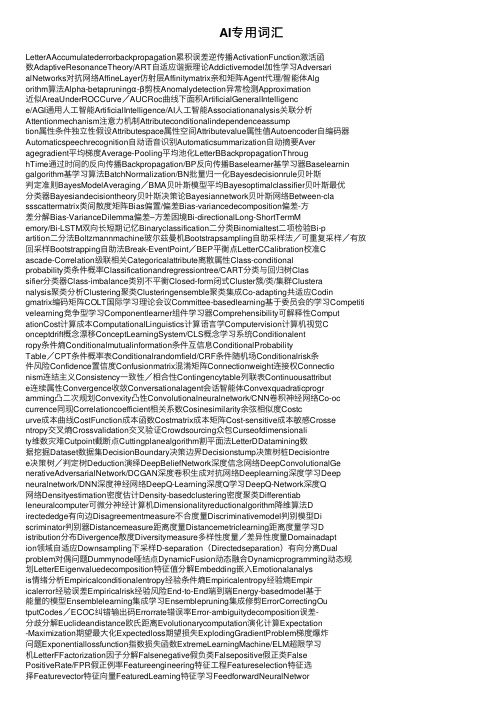
AI专⽤词汇LetterAAccumulatederrorbackpropagation累积误差逆传播ActivationFunction激活函数AdaptiveResonanceTheory/ART⾃适应谐振理论Addictivemodel加性学习Adversari alNetworks对抗⽹络AffineLayer仿射层Affinitymatrix亲和矩阵Agent代理/智能体Algorithm算法Alpha-betapruningα-β剪枝Anomalydetection异常检测Approximation近似AreaUnderROCCurve/AUCRoc曲线下⾯积ArtificialGeneralIntelligence/AGI通⽤⼈⼯智能ArtificialIntelligence/AI⼈⼯智能Associationanalysis关联分析Attentionmechanism注意⼒机制Attributeconditionalindependenceassumption属性条件独⽴性假设Attributespace属性空间Attributevalue属性值Autoencoder⾃编码器Automaticspeechrecognition⾃动语⾳识别Automaticsummarization⾃动摘要Aver agegradient平均梯度Average-Pooling平均池化LetterBBackpropagationThroughTime通过时间的反向传播Backpropagation/BP反向传播Baselearner基学习器Baselearnin galgorithm基学习算法BatchNormalization/BN批量归⼀化Bayesdecisionrule贝叶斯判定准则BayesModelAveraging/BMA贝叶斯模型平均Bayesoptimalclassifier贝叶斯最优分类器Bayesiandecisiontheory贝叶斯决策论Bayesiannetwork贝叶斯⽹络Between-cla ssscattermatrix类间散度矩阵Bias偏置/偏差Bias-variancedecomposition偏差-⽅差分解Bias-VarianceDilemma偏差–⽅差困境Bi-directionalLong-ShortTermMemory/Bi-LSTM双向长短期记忆Binaryclassification⼆分类Binomialtest⼆项检验Bi-partition⼆分法Boltzmannmachine玻尔兹曼机Bootstrapsampling⾃助采样法/可重复采样/有放回采样Bootstrapping⾃助法Break-EventPoint/BEP平衡点LetterCCalibration校准Cascade-Correlation级联相关Categoricalattribute离散属性Class-conditionalprobability类条件概率Classificationandregressiontree/CART分类与回归树Classifier分类器Class-imbalance类别不平衡Closed-form闭式Cluster簇/类/集群Clusteranalysis聚类分析Clustering聚类Clusteringensemble聚类集成Co-adapting共适应Codin gmatrix编码矩阵COLT国际学习理论会议Committee-basedlearning基于委员会的学习Competiti velearning竞争型学习Componentlearner组件学习器Comprehensibility可解释性Comput ationCost计算成本ComputationalLinguistics计算语⾔学Computervision计算机视觉C onceptdrift概念漂移ConceptLearningSystem/CLS概念学习系统Conditionalentropy条件熵Conditionalmutualinformation条件互信息ConditionalProbabilityTable/CPT条件概率表Conditionalrandomfield/CRF条件随机场Conditionalrisk条件风险Confidence置信度Confusionmatrix混淆矩阵Connectionweight连接权Connectionism连结主义Consistency⼀致性/相合性Contingencytable列联表Continuousattribute连续属性Convergence收敛Conversationalagent会话智能体Convexquadraticprogramming凸⼆次规划Convexity凸性Convolutionalneuralnetwork/CNN卷积神经⽹络Co-oc currence同现Correlationcoefficient相关系数Cosinesimilarity余弦相似度Costcurve成本曲线CostFunction成本函数Costmatrix成本矩阵Cost-sensitive成本敏感Crosse ntropy交叉熵Crossvalidation交叉验证Crowdsourcing众包Curseofdimensionality维数灾难Cutpoint截断点Cuttingplanealgorithm割平⾯法LetterDDatamining数据挖掘Dataset数据集DecisionBoundary决策边界Decisionstump决策树桩Decisiontree决策树/判定树Deduction演绎DeepBeliefNetwork深度信念⽹络DeepConvolutionalGe nerativeAdversarialNetwork/DCGAN深度卷积⽣成对抗⽹络Deeplearning深度学习Deep neuralnetwork/DNN深度神经⽹络DeepQ-Learning深度Q学习DeepQ-Network深度Q⽹络Densityestimation密度估计Density-basedclustering密度聚类Differentiab leneuralcomputer可微分神经计算机Dimensionalityreductionalgorithm降维算法D irectededge有向边Disagreementmeasure不合度量Discriminativemodel判别模型Di scriminator判别器Distancemeasure距离度量Distancemetriclearning距离度量学习D istribution分布Divergence散度Diversitymeasure多样性度量/差异性度量Domainadaption领域⾃适应Downsampling下采样D-separation(Directedseparation)有向分离Dual problem对偶问题Dummynode哑结点DynamicFusion动态融合Dynamicprogramming动态规划LetterEEigenvaluedecomposition特征值分解Embedding嵌⼊Emotionalanalysis情绪分析Empiricalconditionalentropy经验条件熵Empiricalentropy经验熵Empiricalerror经验误差Empiricalrisk经验风险End-to-End端到端Energy-basedmodel基于能量的模型Ensemblelearning集成学习Ensemblepruning集成修剪ErrorCorrectingOu tputCodes/ECOC纠错输出码Errorrate错误率Error-ambiguitydecomposition误差-分歧分解Euclideandistance欧⽒距离Evolutionarycomputation演化计算Expectation-Maximization期望最⼤化Expectedloss期望损失ExplodingGradientProblem梯度爆炸问题Exponentiallossfunction指数损失函数ExtremeLearningMachine/ELM超限学习机LetterFFactorization因⼦分解Falsenegative假负类Falsepositive假正类False PositiveRate/FPR假正例率Featureengineering特征⼯程Featureselection特征选择Featurevector特征向量FeaturedLearning特征学习FeedforwardNeuralNetworks/FNN前馈神经⽹络Fine-tuning微调Flippingoutput翻转法Fluctuation震荡Forwards tagewisealgorithm前向分步算法Frequentist频率主义学派Full-rankmatrix满秩矩阵Func tionalneuron功能神经元LetterGGainratio增益率Gametheory博弈论Gaussianker nelfunction⾼斯核函数GaussianMixtureModel⾼斯混合模型GeneralProblemSolving通⽤问题求解Generalization泛化Generalizationerror泛化误差Generalizatione rrorbound泛化误差上界GeneralizedLagrangefunction⼴义拉格朗⽇函数Generalized linearmodel⼴义线性模型GeneralizedRayleighquotient⼴义瑞利商GenerativeAd versarialNetworks/GAN⽣成对抗⽹络GenerativeModel⽣成模型Generator⽣成器Genet icAlgorithm/GA遗传算法Gibbssampling吉布斯采样Giniindex基尼指数Globalminimum全局最⼩GlobalOptimization全局优化Gradientboosting梯度提升GradientDescent梯度下降Graphtheory图论Ground-truth真相/真实LetterHHardmargin硬间隔Hardvoting硬投票Harmonicmean调和平均Hessematrix海塞矩阵Hiddendynamicmodel隐动态模型H iddenlayer隐藏层HiddenMarkovModel/HMM隐马尔可夫模型Hierarchicalclustering层次聚类Hilbertspace希尔伯特空间Hingelossfunction合页损失函数Hold-out留出法Homo geneous同质Hybridcomputing混合计算Hyperparameter超参数Hypothesis假设Hypothe sistest假设验证LetterIICML国际机器学习会议Improvediterativescaling/IIS改进的迭代尺度法Incrementallearning增量学习Independentandidenticallydistributed/i.i.d.独⽴同分布IndependentComponentAnalysis/ICA独⽴成分分析Indicatorfunction指⽰函数Individuallearner个体学习器Induction归纳Inductivebias归纳偏好I nductivelearning归纳学习InductiveLogicProgramming/ILP归纳逻辑程序设计Infor mationentropy信息熵Informationgain信息增益Inputlayer输⼊层Insensitiveloss不敏感损失Inter-clustersimilarity簇间相似度InternationalConferencefor MachineLearning/ICML国际机器学习⼤会Intra-clustersimilarity簇内相似度Intrinsicvalue固有值IsometricMapping/Isomap等度量映射Isotonicregression等分回归It erativeDichotomiser迭代⼆分器LetterKKernelmethod核⽅法Kerneltrick核技巧K ernelizedLinearDiscriminantAnalysis/KLDA核线性判别分析K-foldcrossvalidationk折交叉验证/k倍交叉验证K-MeansClusteringK–均值聚类K-NearestNeighb oursAlgorithm/KNNK近邻算法Knowledgebase知识库KnowledgeRepresentation知识表征LetterLLabelspace标记空间Lagrangeduality拉格朗⽇对偶性Lagrangemultiplier拉格朗⽇乘⼦Laplacesmoothing拉普拉斯平滑Laplaciancorrection拉普拉斯修正Latent DirichletAllocation隐狄利克雷分布Latentsemanticanalysis潜在语义分析Latentvariable隐变量Lazylearning懒惰学习Learner学习器Learningbyanalogy类⽐学习Learn ingrate学习率LearningVectorQuantization/LVQ学习向量量化Leastsquaresre gressiontree最⼩⼆乘回归树Leave-One-Out/LOO留⼀法linearchainconditional randomfield线性链条件随机场LinearDiscriminantAnalysis/LDA线性判别分析Linearmodel线性模型LinearRegression线性回归Linkfunction联系函数LocalMarkovproperty局部马尔可夫性Localminimum局部最⼩Loglikelihood对数似然Logodds/logit对数⼏率Lo gisticRegressionLogistic回归Log-likelihood对数似然Log-linearregression对数线性回归Long-ShortTermMemory/LSTM长短期记忆Lossfunction损失函数LetterM Machinetranslation/MT机器翻译Macron-P宏查准率Macron-R宏查全率Majorityvoting绝对多数投票法Manifoldassumption流形假设Manifoldlearning流形学习Margintheory间隔理论Marginaldistribution边际分布Marginalindependence边际独⽴性Marginalization边际化MarkovChainMonteCarlo/MCMC马尔可夫链蒙特卡罗⽅法MarkovRandomField马尔可夫随机场Maximalclique最⼤团MaximumLikelihoodEstimation/MLE极⼤似然估计/极⼤似然法Maximummargin最⼤间隔Maximumweightedspanningtree最⼤带权⽣成树Max-P ooling最⼤池化Meansquarederror均⽅误差Meta-learner元学习器Metriclearning度量学习Micro-P微查准率Micro-R微查全率MinimalDescriptionLength/MDL最⼩描述长度Minim axgame极⼩极⼤博弈Misclassificationcost误分类成本Mixtureofexperts混合专家Momentum动量Moralgraph道德图/端正图Multi-classclassification多分类Multi-docum entsummarization多⽂档摘要Multi-layerfeedforwardneuralnetworks多层前馈神经⽹络MultilayerPerceptron/MLP多层感知器Multimodallearning多模态学习Multipl eDimensionalScaling多维缩放Multiplelinearregression多元线性回归Multi-re sponseLinearRegression/MLR多响应线性回归Mutualinformation互信息LetterN Naivebayes朴素贝叶斯NaiveBayesClassifier朴素贝叶斯分类器Namedentityrecognition命名实体识别Nashequilibrium纳什均衡Naturallanguagegeneration/NLG⾃然语⾔⽣成Naturallanguageprocessing⾃然语⾔处理Negativeclass负类Negativecorrelation负相关法NegativeLogLikelihood负对数似然NeighbourhoodComponentAnalysis/NCA近邻成分分析NeuralMachineTranslation神经机器翻译NeuralTuringMachine神经图灵机Newtonmethod⽜顿法NIPS国际神经信息处理系统会议NoFreeLunchTheorem /NFL没有免费的午餐定理Noise-contrastiveestimation噪⾳对⽐估计Nominalattribute列名属性Non-convexoptimization⾮凸优化Nonlinearmodel⾮线性模型Non-metricdistance⾮度量距离Non-negativematrixfactorization⾮负矩阵分解Non-ordinalattribute⽆序属性Non-SaturatingGame⾮饱和博弈Norm范数Normalization归⼀化Nuclearnorm核范数Numericalattribute数值属性LetterOObjectivefunction⽬标函数Obliquedecisiontree斜决策树Occam’srazor奥卡姆剃⼑Odds⼏率Off-Policy离策略Oneshotlearning⼀次性学习One-DependentEstimator/ODE独依赖估计On-Policy在策略Ordinalattribute有序属性Out-of-bagestimate包外估计Outputlayer输出层Outputsmearing输出调制法Overfitting过拟合/过配Oversampling过采样LetterPPairedt-test成对t检验Pairwise成对型PairwiseMarkovproperty成对马尔可夫性Parameter参数Parameterestimation参数估计Parametertuning调参Parsetree解析树ParticleSwarmOptimization/PSO粒⼦群优化算法Part-of-speechtagging词性标注Perceptron感知机Performanceme asure性能度量PlugandPlayGenerativeNetwork即插即⽤⽣成⽹络Pluralityvoting相对多数投票法Polaritydetection极性检测Polynomialkernelfunction多项式核函数Pooling池化Positiveclass正类Positivedefinitematrix正定矩阵Post-hoctest后续检验Post-pruning后剪枝potentialfunction势函数Precision查准率/准确率Prepruning预剪枝Principalcomponentanalysis/PCA主成分分析Principleofmultipleexplanations多释原则Prior先验ProbabilityGraphicalModel概率图模型ProximalGradientDescent/PGD近端梯度下降Pruning剪枝Pseudo-label伪标记LetterQQuantizedNeu ralNetwork量⼦化神经⽹络Quantumcomputer量⼦计算机QuantumComputing量⼦计算Quasi Newtonmethod拟⽜顿法LetterRRadialBasisFunction/RBF径向基函数RandomFo restAlgorithm随机森林算法Randomwalk随机漫步Recall查全率/召回率ReceiverOperatin gCharacteristic/ROC受试者⼯作特征RectifiedLinearUnit/ReLU线性修正单元Recurr entNeuralNetwork循环神经⽹络Recursiveneuralnetwork递归神经⽹络Referencemodel参考模型Regression回归Regularization正则化Reinforcementlearning/RL强化学习Representationlearning表征学习Representertheorem表⽰定理reproducingke rnelHilbertspace/RKHS再⽣核希尔伯特空间Re-sampling重采样法Rescaling再缩放Residu alMapping残差映射ResidualNetwork残差⽹络RestrictedBoltzmannMachine/RBM受限玻尔兹曼机RestrictedIsometryProperty/RIP限定等距性Re-weighting重赋权法Robu stness稳健性/鲁棒性Rootnode根结点RuleEngine规则引擎Rulelearning规则学习LetterS Saddlepoint鞍点Samplespace样本空间Sampling采样Scorefunction评分函数Self-Driving⾃动驾驶Self-OrganizingMap/SOM⾃组织映射Semi-naiveBayesclassifiers半朴素贝叶斯分类器Semi-SupervisedLearning半监督学习semi-SupervisedSupportVec torMachine半监督⽀持向量机Sentimentanalysis情感分析Separatinghyperplane分离超平⾯SigmoidfunctionSigmoid函数Similaritymeasure相似度度量Simulatedannealing模拟退⽕Simultaneouslocalizationandmapping同步定位与地图构建SingularV alueDecomposition奇异值分解Slackvariables松弛变量Smoothing平滑Softmargin软间隔Softmarginmaximization软间隔最⼤化Softvoting软投票Sparserepresentation稀疏表征Sparsity稀疏性Specialization特化SpectralClustering谱聚类SpeechRecognition语⾳识别Splittingvariable切分变量Squashingfunction挤压函数Stability-plasticitydilemma可塑性-稳定性困境Statisticallearning统计学习Statusfeaturefunction状态特征函Stochasticgradientdescent随机梯度下降Stratifiedsampling分层采样Structuralrisk结构风险Structuralriskminimization/SRM结构风险最⼩化S ubspace⼦空间Supervisedlearning监督学习/有导师学习supportvectorexpansion⽀持向量展式SupportVectorMachine/SVM⽀持向量机Surrogatloss替代损失Surrogatefunction替代函数Symboliclearning符号学习Symbolism符号主义Synset同义词集LetterTT-Di stributionStochasticNeighbourEmbedding/t-SNET–分布随机近邻嵌⼊Tensor张量TensorProcessingUnits/TPU张量处理单元Theleastsquaremethod最⼩⼆乘法Th reshold阈值Thresholdlogicunit阈值逻辑单元Threshold-moving阈值移动TimeStep时间步骤Tokenization标记化Trainingerror训练误差Traininginstance训练⽰例/训练例Tran sductivelearning直推学习Transferlearning迁移学习Treebank树库Tria-by-error试错法Truenegative真负类Truepositive真正类TruePositiveRate/TPR真正例率TuringMachine图灵机Twice-learning⼆次学习LetterUUnderfitting⽋拟合/⽋配Undersampling⽋采样Understandability可理解性Unequalcost⾮均等代价Unit-stepfunction单位阶跃函数Univariatedecisiontree单变量决策树Unsupervisedlearning⽆监督学习/⽆导师学习Unsupervisedlayer-wisetraining⽆监督逐层训练Upsampling上采样LetterVVanishingGradientProblem梯度消失问题Variationalinference变分推断VCTheoryVC维理论Versionspace版本空间Viterbialgorithm维特⽐算法VonNeumannarchitecture冯·诺伊曼架构LetterWWassersteinGAN/WGANWasserstein⽣成对抗⽹络Weaklearner弱学习器Weight权重Weightsharing权共享Weightedvoting加权投票法Within-classscattermatrix类内散度矩阵Wordembedding词嵌⼊Wordsensedisambiguation词义消歧LetterZZero-datalearning零数据学习Zero-shotlearning零次学习。
自适应搜索目标的快速彩色复合人脸检测方法
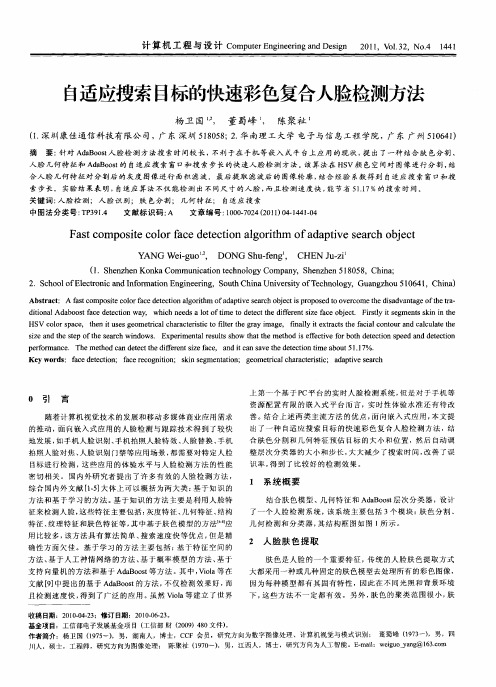
自适应搜索 目标的快速彩色复合人脸检测方法
杨 卫 国 , 董 蜀峰 陈聚祉 ,
0 引 言
随着 计 算 机 视 觉 技 术 的 发 展 和 移 动 多 媒 体 商 业 应 用 需 求
关 键 词 : 脸 检 测 ; 人 脸 识 别 ; 肤 色分 割 ; 几何 特 征 ; 自适 应 搜 索 人
中图法分 类号 :P 9. T 3 1 4
文 献标识码 : A
文章编 号 :0072 2 1) 414 —4 10 —04(0 1 0.4 1 0
F sc mp s ec lr a e eet nag rh o a t esac bet at o oi oo c tci loi m f d pi erhojc t f d o t a v
Y ANG e— u , DON G h —e g, CHE J — i W i o g S u fn N u z
ห้องสมุดไป่ตู้
【 .S e z e n aCo 1 h n h nKo k mmu iaintc n lg mp n ,S e z e 0 8 hn ; nc t h oo yCo a y h n h n5 5 ,C ia o e 1 8
p ro ma c . Th t o al e e t ed fe e t ief c 。 a di c n s v ed t c in t b u 1 % . e r ne f eme h dc l d t c i r n z e h t s a n a a et ee t mea o t 1 t h o i 5 .7
刘萍萍翻译
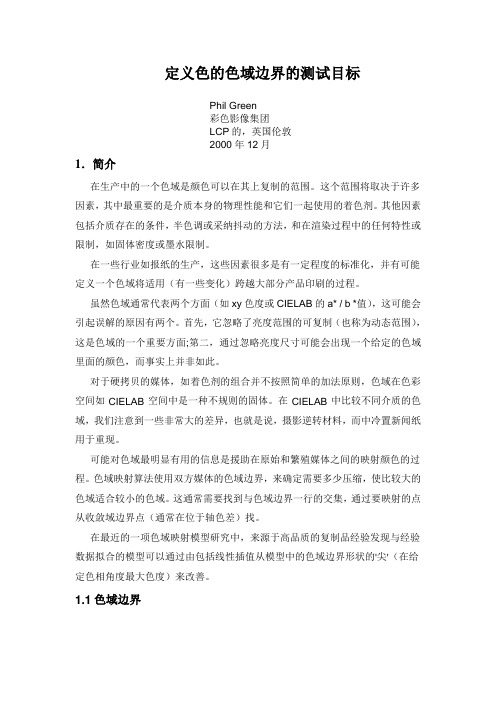
定义色的色域边界的测试目标Phil Green彩色影像集团LCP的,英国伦敦2000年12月1.简介在生产中的一个色域是颜色可以在其上复制的范围。
这个范围将取决于许多因素,其中最重要的是介质本身的物理性能和它们一起使用的着色剂。
其他因素包括介质存在的条件,半色调或采纳抖动的方法,和在渲染过程中的任何特性或限制,如固体密度或墨水限制。
在一些行业如报纸的生产,这些因素很多是有一定程度的标准化,并有可能定义一个色域将适用(有一些变化)跨越大部分产品印刷的过程。
虽然色域通常代表两个方面(如xy色度或CIELAB的a* / b *值),这可能会引起误解的原因有两个。
首先,它忽略了亮度范围的可复制(也称为动态范围),这是色域的一个重要方面;第二,通过忽略亮度尺寸可能会出现一个给定的色域里面的颜色,而事实上并非如此。
对于硬拷贝的媒体,如着色剂的组合并不按照简单的加法原则,色域在色彩空间如CIELAB空间中是一种不规则的固体。
在CIELAB中比较不同介质的色域,我们注意到一些非常大的差异,也就是说,摄影逆转材料,而中冷置新闻纸用于重现。
可能对色域最明显有用的信息是援助在原始和繁殖媒体之间的映射颜色的过程。
色域映射算法使用双方媒体的色域边界,来确定需要多少压缩,使比较大的色域适合较小的色域。
这通常需要找到与色域边界一行的交集,通过要映射的点从收敛域边界点(通常在位于轴色差)找。
在最近的一项色域映射模型研究中,来源于高品质的复制品经验发现与经验数据拟合的模型可以通过由包括线性插值从模型中的色域边界形状的'尖'(在给定色相角度最大色度)来改善。
1.1色域边界计算色域边界的许多方法被描述在[1,2,3,4,5,6]。
这些方法的主要特点由Morovic[6进行了总结]。
不同的媒体和图像的色域也已在最近文件[7,8]中进行了比较。
在着色剂空间,色域边界可以被视为一个立方体因为在顶点它有固体着色剂和他们的第二组合的面孔。
纹理物体缺陷的视觉检测算法研究--优秀毕业论文
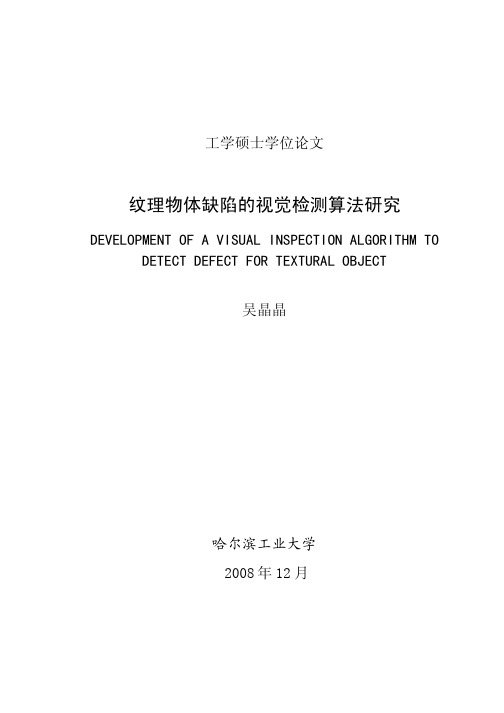
摘 要
在竞争激烈的工业自动化生产过程中,机器视觉对产品质量的把关起着举足 轻重的作用,机器视觉在缺陷检测技术方面的应用也逐渐普遍起来。与常规的检 测技术相比,自动化的视觉检测系统更加经济、快捷、高效与 安全。纹理物体在 工业生产中广泛存在,像用于半导体装配和封装底板和发光二极管,现代 化电子 系统中的印制电路板,以及纺织行业中的布匹和织物等都可认为是含有纹理特征 的物体。本论文主要致力于纹理物体的缺陷检测技术研究,为纹理物体的自动化 检测提供高效而可靠的检测算法。 纹理是描述图像内容的重要特征,纹理分析也已经被成功的应用与纹理分割 和纹理分类当中。本研究提出了一种基于纹理分析技术和参考比较方式的缺陷检 测算法。这种算法能容忍物体变形引起的图像配准误差,对纹理的影响也具有鲁 棒性。本算法旨在为检测出的缺陷区域提供丰富而重要的物理意义,如缺陷区域 的大小、形状、亮度对比度及空间分布等。同时,在参考图像可行的情况下,本 算法可用于同质纹理物体和非同质纹理物体的检测,对非纹理物体 的检测也可取 得不错的效果。 在整个检测过程中,我们采用了可调控金字塔的纹理分析和重构技术。与传 统的小波纹理分析技术不同,我们在小波域中加入处理物体变形和纹理影响的容 忍度控制算法,来实现容忍物体变形和对纹理影响鲁棒的目的。最后可调控金字 塔的重构保证了缺陷区域物理意义恢复的准确性。实验阶段,我们检测了一系列 具有实际应用价值的图像。实验结果表明 本文提出的纹理物体缺陷检测算法具有 高效性和易于实现性。 关键字: 缺陷检测;纹理;物体变形;可调控金字塔;重构
Keywords: defect detection, texture, object distortion, steerable pyramid, reconstruction
II
语义分割的pr曲线
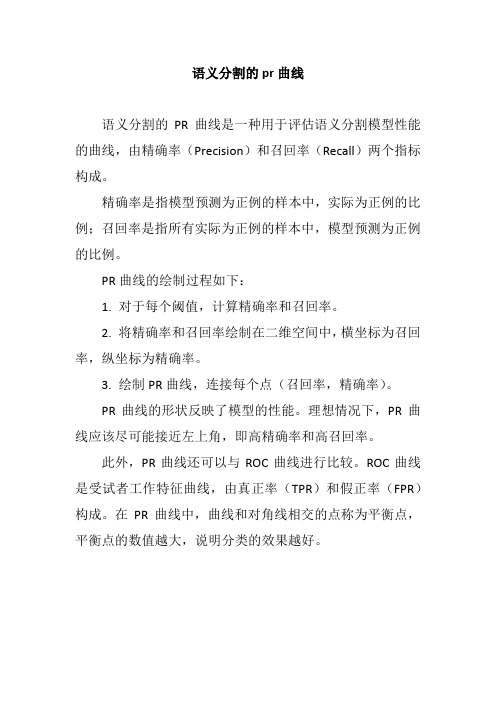
语义分割的pr曲线
语义分割的PR曲线是一种用于评估语义分割模型性能的曲线,由精确率(Precision)和召回率(Recall)两个指标构成。
精确率是指模型预测为正例的样本中,实际为正例的比例;召回率是指所有实际为正例的样本中,模型预测为正例的比例。
PR曲线的绘制过程如下:
1. 对于每个阈值,计算精确率和召回率。
2. 将精确率和召回率绘制在二维空间中,横坐标为召回率,纵坐标为精确率。
3. 绘制PR曲线,连接每个点(召回率,精确率)。
PR曲线的形状反映了模型的性能。
理想情况下,PR曲线应该尽可能接近左上角,即高精确率和高召回率。
此外,PR曲线还可以与ROC曲线进行比较。
ROC曲线是受试者工作特征曲线,由真正率(TPR)和假正率(FPR)构成。
在PR曲线中,曲线和对角线相交的点称为平衡点,平衡点的数值越大,说明分类的效果越好。
tracepro学习经验和知识点

• 2.先在薄膜堆层编辑器中定义几层薄膜,保 存后可嵌入到你定义的一个表面属性,然 后应用属性到表面
定义表面光源
• Flux和irradiance是不连续光源 • Blackbody和graybody是连续光源 • 不连续波段和连续波段的选择是针对
Tracepro中光线颜色的介绍 2023最新整理收集 do something
• 当只有单色光作用时 • 1.红色表示光线衰减至原来的100%~66%; • 2.绿色表示光线衰减至原来的66%~33%; • 3.蓝色表示光线衰减至原来的33%~0%。 • 但建立多色光源时,光线颜色不再表示能
量,而是表示不同颜色的光
重点采样的设置
• 1.设置面光源 • 2.在该面光源的apply properties中选取
importance sampling选项卡,在该选项卡 中增加重点采样面
重点采样面设置
重点采样
• 手动重点采样和自动重点采样 • 自动设置重点采样可以用于已经定义了
prescription的光学系统 • 注: prescription为属性选项卡,它规定了
3.irradiance map 看到的是通过2中选出的光线携带的能 量,所以在各个中间的面上,irradiance map得到的结果 里,总能量上都是一致的。 作小模型验证过
4exit surface 上出射的能量是如何计算的? 从现有的文献看一开始并不知道是多少,是方向的计算得 来得,具体的计算方法,待详。
理论或测量得到数据制成光源文件
• 按照上一ppt页的制作方法保存为txt格式后, 删除其值,输入实测值,特别注意文件头
- 1、下载文档前请自行甄别文档内容的完整性,平台不提供额外的编辑、内容补充、找答案等附加服务。
- 2、"仅部分预览"的文档,不可在线预览部分如存在完整性等问题,可反馈申请退款(可完整预览的文档不适用该条件!)。
- 3、如文档侵犯您的权益,请联系客服反馈,我们会尽快为您处理(人工客服工作时间:9:00-18:30)。
Shape Filtering for False Positive Reduction at Computed Tomography Colonography Abhilash A.Miranda,Tarik A.Chowdhury,Ovidiu Ghita,Paul F.Whelan Vision Systems Group,Dublin City University,IrelandAbstract.In this paper,we treat the problem of reducing the false pos-itives(FP)in the automatic detection of colorectal polyps at ComputerAided Detection in Computed Tomography Colonography(CAD-CTC)as a shape-filtering task.From the extracted candidate surface,we obtaina reliable shape distribution function and analyse it in the Fourier domainand use the resulting spectral data to classify the candidate surface asbelonging to a polyp or a non-polyp class.The developed shapefilteringscheme is computationally efficient(takes approximately2seconds perdataset to detect the polyps from the colonic surface)and offers robustpolyp detection with an overall false positive rate of5.44per dataset ata sensitivity of100%for polyps greater than10mm when it was appliedto standard and low dose CT data.1IntroductionAccording to the World Health Report[1],colorectal cancer caused approxi-mately622,000deaths in2002.It is understood that the risk of colon cancer could be reduced considerably if growths in the colon called polyps are removed before they become cancerous.CAD-CTC is being developed as a reliable technique for early detection of pre-malignant polyps useful in mass screening of risky individuals.It involves the analysis of the images of the colon obtained using a Computed Tomography(CT) examination of the abdominal puter vision is used for the automatic detection of potential polyps by analyzing the three-dimensional model of the colon obtained by combining the two-dimensional axial images obtained from the CT examination.Many methods developed for CAD-CTC suffer from severe false-positive(FP)findings owing to the confusion in correctly distinguishing polyps from other colonic shapes such as folds,residual materials,etc.In this paper,we propose a novel method to accurately discriminate the polyp shapes from other colonic shapes(folds)by analysing a reliable shape distrib-ution function(SDF)in the frequency domain.After a detailed analysis of the SDF in the frequency domain,we developed a novel technique that addresses the polyp−non-polyp classification as a shapefiltering problem.In order to evaluate the validity of the proposed technique we have analysed its performance when applied to a large number of synthetic(scanned with standard and low radiation dose)and real CT datasets(scanned at a standard radiation dose).This paper is organised as follows.In section3,the method designed to extract the candidate surfaces from the CT data is briefly explained.In section Corresponding Author:abhilash@eeng.dcu.ie24,we introduce the SDF of an extracted colonic surface and discuss its properties. The next two sections discuss the properties of the polyp and non-polyp SDFs and explain the motivation for analysing the SDF in the frequency domain in terms of its power spectral density.In section5.1,we detail the implementation of the novel shapefiltering scheme developed for polyp−non-polyp classification. In the concluding section,we present the results obtained on the large number of real patient datasets on which the shapefiltering technique was tested.2Materials and MethodIn order to perform bowel cleansing,the patients were instructed to follow a low-residue diet for48hours,followed by the ingestion of clearfluids for24 hours.Before the examination,the colon is gently insufflated with room air at the maximum level tolerated by the patient and the CT data acquisition was performed on a Siemens-Somatom4-slice multi-detector spiral CT scanner by the radiologists at the Mater Hospital,Dublin,Ireland.The scanning parame-ters in use are120kVp,100mAs tube current,2.5mm collimation,3mm slice thickness,1.5mm reconstruction interval,and0.5gantry rotation.The scanning is performed in a single breath-hold and the acquisition time is in the interval of20to30seconds depending on the body-size of the patient.The scanning is performedfirst with the patient in the supine position and then repeated with the patient in the prone position.Typically the number of slices varies from200 to350and the total size of the volumetric CT data is approximately150MB. 3Candidate surface extractionThe candidate surface extraction method consists of two main stages.In thefirst stage,the colonic wall is identified in the CT data based on the high contrast between the gas insufflated regions and the colon tissue.In this way,the gaseous region can be successfully identified by using a simple region growing algorithm where the colonic wall is defined by the voxels that are adjacent to the colon tissue.The threshold value for region growing algorithm was set to−800HU[2] to ensure that only the voxels inside the colon(gasfilled volume)are segmented. The second stage of the algorithm attempts to identify the convex surfaces from the colon wall by analysing the intersections of the normal vectors which are calculated using the Zucker-Hummel operator[3].The normal intersections are recorded for a number of Hough points that are uniformly distributed from2.5 mm to10mm in the normal direction for each voxel of the colon wall.The normal intersections are recorded into a3D histogram and the candidate surfaces are generated by the Hough points that have more than5intersections in the3D histogram.In order to eliminate the voxels associated with spurious non-convex surfaces an additional convexity test is performed[2],[4].During the candidate surface processing,the centre-point and the radius of each candidate cluster is calculated byfinding the sum of weighted Gaussian distances d G for each point of the surface(full details about the implementation of this algorithm can be found in[2]).3 4Shape Distribution FunctionRobust polyp detection is very difficult to achieve since the polyps and other convex colonic structures such as folds have a large number of shapes and sizes. Typically the polyps can be assumed to have a spherical shape where the nominal model for folds is cylindrical.Based on this geometrical characterization,many researchers attempted to perform the polyp/fold classification using geometrical features that are extracted from candidate surfaces[5][6][7].Unfortunately very often the polyp and fold surfaces present very subtle shape differences as illus-trated in Fig.1and as a result the geometrical approaches return high sensitivity in polyp detection but at the expense of an increased number of false positives.Fig.1.Images illustrating some examples of the extracted surfaces for polyps(first row)and folds(second row).In the next section we detail the shape distribution function and describe its properties that make it suitable for robust polyp−non-polyp classification.4.1Definition and PropertiesFor a given candidate colonic surface,the computation of the shape distribution function that we use in this paper can be explained as follows.Given the coordi-nates of the n voxels defining the candidate surface U and the Gaussian center v of the voxels[4]associated with the candidate surface,wefind the n−length array w which lists the Euclidean distances between each of the n surface voxels and the Gaussian center v of the candidate surface U.We call the histogram x of the entries in w as the shape distribution function(SDF)of the candidate colonic surface U,which is a distribution function of the relative distances of the surface voxels of the candidate colonic surface.The SDF x is simple in definition and computationally efficient.Also,x is robust to characterise the shape of the candidate surface when the voxel data is sparse or affected by noise.Since x is invariant to rotation and translation of the surface,it has a very important advantage that it is dependent only on the colonic shape and independent of the colonic orientation.The SDF used here is similar to the shape distribution function D1used for object recognition[8].Another important benefit in using the SDF is the significant reduction in the dimensionality that is achieved by transforming3D data(U)to a1D time-series(x)without losing interesting shape cues.This dimensionality reduction, as discussed later,reduces the complexity of the analysis thus making it suitable for real-time operation.45Analysis of the SDFs using the AutocorrelationFunctionsSome of the observed general characteristics and behaviours of the 3−neighbour averaged samples SDFs of polyps and non-polyps illustrated in Fig.2are as follows.The SDF of a polyp is,in general,a smooth curve having a single andDistance Bins N u m b e ro f V o x e l s Distance Bins Fig.2.SDFs of polyps and non-polyps surfaces from real-datasets shows significant differences in smoothness and maxima characteristics.global maxima.Conversely,a non-polyp SDF is noisy and might have single or multiple maxima,which might be similar to that of polyps,but whose location or variance near the neighbourhood of the maxima are random.We now briefly describe the observed characteristics of the SDF and evaluate the need to analyse the autocorrelation functions of the SDF for obtaining the information that differentiates the polyp surfaces from non-polyp surfaces.Although the SDFs for polyp and fold surfaces depicted in Fig.2offer the primary discrimination between these surfaces,there is no robust directly recog-nisable shape for the SDF curve for any one of the classes.As a result,we cannot apply straight-forward SDF shape matching technique to classify polyps from non-polyps.In addition,since there are multiple maxima with unpredictable variances in their neighbourhoods for a non-polyp SDF,techniques based on probability density function fitting or variance-based feature extraction will be less efficient in finding optimal patterns to classify non-polyp surfaces correctly.Since the polyp and non-polyp SDFs are generated by unknown probability distributions and appear to be corrupted by noise,there is an uncertainty in the location and variance of the maxima in the SDFs.Hence,it is necessary to compare each of the SDF time-series x with a delayed version of itself and others.This can be done by analysing the autocorrelation functions R xx of the SDFs.Moreover,in order to study the effects of noisy frequency components on the variance (power)of the SDF,it is necessary to see the contribution of the vari-ous frequency components of x to the total variance.According to the Wiener-Khinchin theorem,the power spectral density S xx of a signal x is the Fourier transform of R xx .In practice,for computational purposes,the S xx is calculated using Fast Fourier Transform methods.55.1Power Spectral Density of SDFsIn order to understand the distribution of the variance of the SDFs in the fre-quency domain,we analyse the power spectral density of the SDFs on which we make certain interesting observations which help us to design a robust shape filtering scheme as explained in this section.Let x =x 0,...,x M −1be the time-series corresponding to an SDF.The samples of its discrete Fourier transform is given by X l = M −1k =0x k e −i 2πkl M so that the power spectral density of SDF is given by S xx l =X l X ∗l ,the product of X l and its conjugate and normalised in accordance with the desired resolution of X l .We used a 128-point FFT to compute the power spectral density S xx of the SDFs and we noticed that S xx of the fold surfaces attenuate much quicker than those associated with polyps.Our experiments indicate that the frequency f dB at which S xx reaches 12.5%of its power at DC frequency offers a robust discrimination between the polyp and fold surfaces as illustrated in the Fig.3.Polyp Power Spectral Density S Frequency in Hz S x x i n (n u m o f v o x el s /H z ) 2Non−polyp Power Spectral Density S Frequency in Hz Fig.3.S xx for the Shape Distribution Functions used in Fig.2shows faster attenuation for Non-polyps than PolypsThus the classification criterion can be defined as follows:an SDF belongs to the polyp class if its f dB is higher than an experimentally selected threshold frequency f th .The threshold-based rejection rule mentioned above works very well for small and medium sized polyps but it rejects very large polyps whose SDFs are not very different from those associated with folds.Based on observations on an ex-perimental set of samples,we find that for certain large polyps f dB lies in an uncertain classification region f min <f th <f max .References [4]and [2]have shown that the sum of the weighted Gaussian distances d G of the voxels that define the candidate surface offers robust discrimination between the surfaces associated with large polyps and those derived from folds.Based on the ex-perimentation with various sizes of polyps having a weighted sum of Gaussian distances,d 1and d 2,corresponding to f min and f max ,we devised a techniqueto compute adaptively the classification threshold frequency f th according to the following linear function:f th =f max +(d G −d 2)f min −f max d 1−d 2.In this way,a SDF belongs to a polyp surface if its f dB is higher than the computed thresholdfrequency f th ,otherwise it is classed as a fold surface.66Experiments and ResultsIn this section we discuss the tests that we carried out using the shapefiltering scheme and present the results obtained which we compare with other established CAD-CTC techniques.The shapefiltering CAD-CTC technique was tested on61real datasets ob-tained as part of the clinical study in conjunction with the Mater Hospital, Dublin,Ireland.The shapefiltering technique was also tested on custom-built phantom datasets[9]which contain a large number of synthetic structures which replicate accurately the real polyp shapes encountered in clinical studies.One of the main concerns associated with CTC as a mass screening tech-nique for colorectal polyp detection is the patient exposure to ionising radiation.A reduction in the radiation dose will increase the level of noise in the CT data and our aim is to fully evaluate the impact of the radiation dose on the perfor-mance of our CAD-CTC system.In this regard,we have used in our experiments a synthetic phantom that have47synthetic polyps that have different shapes (sessile,pedunculated andflat)and sizes(3to18mm).In our experiments we used the phantom CT data that has been obtained for the following radiation doses:100mAs,60mAs,20mAs,and13mAs.The results of the shapefiltering CAD-CTC for the Phantom Data[9]are summarised in Tables1to4.The ex-perimental results indicate that the radiation dose does not have a significant impact on the performance of our polyp detection method since the sensitivity for clinical relevant polyps(larger than10mm)is not affected.A small reduction in sensitivity for polyps in the range5-10mm has been noticed when the data has been scanned with20mAs radiation dose.Table5shows the performance of the shapefiltering technique when applied to61real patient datasets.These results were validated by our clinical part-ners from the Mater Hospital,Dublin,Ireland and indicate that the technique achieved100%sensitivity for detection of polyps larger than>10mm which are the most important features to be examined in clinical studies.The experimen-tal data also show that there is a high true positive rate for polyps with sizes ranging between5mm-10mm where a sensitivity of81.25%is achieved in spite of some polyps having very complex shapes.The false positives returned by our shapefiltering method stands at an average of5.44per dataset and compares well with the techniques that will be examined in the next section.The method detailed in this paper has not been developed to primarily detect theflat polyps since these polyps have many geometrical characteristics that are similar with those of the folds.High sensitivity forflat polyps detection cannot be achieved by using methods that attempt to discriminate the polyp and fold surfaces based on global shape models and theflat polyps should be approached as a distinct category of polyps.The average time for surface extraction per dataset for processing each vol-ume of data is approximately80seconds on a Pentium-IV3-GHz processor machine with1GB memory.The computation of the power spectral density S xx and the shape-filtering process takes in average2seconds per dataset when the algorithm was executed on the same machine.7 Table1:Phantom Data(100mAs)Polyp TypeTotalPolypsTruePositivesSensitivity%≥10mm1414100 [5,10)mm1919100 <5mm5480Flat9222.22 Total473983.97 Phantom Dataset(100mAs):FP=1Table2:Phantom Data(60mAs)PolypTypeTotalPolypsTruePositivesSensitivity%≥10mm1414100 [5,10)mm1919100<5mm5480Flat9333.33Total474085.11Phantom Dataset(60mAs):FP=1Table3:Phantom Data(20mAs)Polyp TypeTotalPolypsTruePositivesSensitivity%≥10mm1414100 [5,10)mm191789.47 <5mm5360Flat9222.22 Total473676.60 Phantom Dataset(20mAs):FP=0Table4:Phantom Data(13mAs)PolypTypeTotalPolypsTruePositivesSensitivity%≥10mm141285.71 [5,10)mm191894.74<5mm5360Flat9111.11Total473472.34Phantom Dataset(13mAs):FP=1Table5:Real Datasets(100mAs)Polyp TypeTotalPolypsTruePositivesSensitivity%≥10mm1010100[5,10)mm322681.25<5mm1046259.62Mass11763.64Flat2150Total15910666.89FP/Real Dataset=5.446.1Comparison with Established TechniquesIn order to evaluate the validity of our shapefiltering CAD-CTC we compare its performance with the results in polyp detection obtained by other relevant documented techniques.Though they were not tested on the same datasets,the reported sensitivity and false positive per dataset(FP)should be indicative of their relative robustness in polyp detection.For polyps larger than10mm,the CAD-CTC techniques evaluated in this section present the following results.The method developed by Summers et al.[5]which uses surface curvature information gives a sensitivity of29%−100% with a varying FP rate(6−20)depending on the scanning parameters.The method proposed by Vining et al.[6]based on surface extraction and curvature analysis recorded a73%sensitivity with a very inconsistent FP rate in the range 9−90.Yoshida et al.[7]used various shape indices(cup,rut,saddle,ridge,cap) and curvedness values on small volumes of interest in conjunction with fuzzy clustering.They report a sensitivity of89%with an FP of2.0which increased by a factor of1.5when sensitivity was increased to100%.For polyps larger than5mm,the technique developed by Kiss et al.[4]based on surface normal distribution and spherefitting achieves a sensitivity of90% with a FP of2.82.It is useful to note that this performance is obtained when they applied their method to high resolution CT data(0.8mm reconstruction interval).We have evaluated the performance of our shapefiltering technique on CT data with a lower resolution(1.5mm reconstruction interval)where the problems caused by the partial volume effects are more pronounced.The SDF based shapefiltering technique that we presented in this paper, achieved100%sensitivity for polyps greater than10mm and81.25%sensitivity8for polyp sizes between5−10mm with an overall FP of5.44.The performance in polyp detection of our polyp detection technique compares well with the performance returned by the CAD-CTC systems evaluated in this section.7ConclusionWe have designed and implemented a simple,stable and robust CAD-CTC tech-nique which attains better results than other published CAD-CTC techniques. Currently,the false positive rate stands at an average of5.44FPs per dataset that can be further reduced byfine tuning the technique to increase the detection rate forflat polyps.For this category of polyps,the developed shapefiltering technique achieved the lowest sensitivity.By taking the3D shape recognition into the Fourier domain analysis,we were able to simplify the problem with significant reductions in processing time.We envisage further extensions to the shape-distribution based shape recognition technique to further improve the per-formance in polyp detection for small and medium polyps. AcknowledgmentsWe acknowledge the contributions of our clinical partners in this project:Dr. Helen Fenlon(Dept.of Radiology)and Dr.Padraic MacMathuna(Gastroin-testinal Unit)of the Mater Hospital,Dublin.This work was supported under an Investigator Programme Grant(02/IN1/1056)by Science Foundation Ireland. References1.World Health Organisation:The World Health Report2004.WHO(2004)2.Chowdhury,T.,Ghita,O.,Whelan,P.:A Statistical approach for Robust PolypDetection in CT Colonography.Proceedings of the International Conference of the IEEE Engineering in Medicine and Biology Society27(2005)3.Zucker,S.W.,Hummel,R.A.:A Three-Dimensional Edge Operator.IEEE Trans-actions on Pattern Analysis and Machine Intelligence3(3)(1981)324–3314.Kiss,G.,Cleynenbreugel,J.,Thomeer,M.,Suetens,P.,Marchal,G.:ComputerAided Diagnosis for Virtual Colonography.MICCAI(2001)621–6285.Summers,R.,Johnson,C.,Pusanik,L.,Malley,J.,Youssef,A.,Reed,J.:Auto-mated Polyp Detection at CT Colonography:Feasibility Assessment in a Human Population.Radiology216(2000)284–2906.Vining,D.,Hunt,G.,Ahn,D.,Steltes,D.,Helmer,P.:Computer-Assisted Detectionof Colon Polyps and Masses.Radiology219(2001)51–597.Yoshida,H.,Masutani,Y.,MacEneaney,P.,Rubin,D.,Dachman,A.:Computer-ized Detection of Colonic Polyps at CT Colonography on the Basis of Volumetric Features:Pilot Study.Radiology222(2002)327–3368.Osada,R.,Funkhouser,T.,Dobkin,D.:Matching3D Models with Shape Dis-tributions.International Conference on Shape Modeling and Applications(2001) 154–1669.Chowdhury,T.,Sadleir,R.,Whelan,P.,Moss,A.,Varden,J.,Short,M.,Fenlon,H.,MacMathuna,P.:The Impact of Radiation Dose on Imaged Polyp Characteristics at CT Colonography:Experiments with a Synthetic Phantom.Technical report, Association of Physical Scientists in Medicine,Annual Scientific Meeting,Dublin (2004)。