Forecasting
Forecasting China′s Stock Market Volatility Using
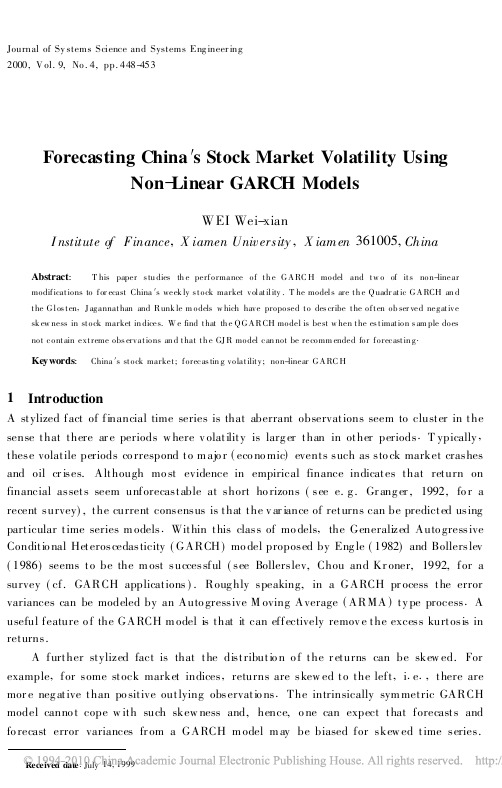
Journal of Sy stems Science and Systems Eng ineer ing2000,V ol.9,No.4,pp.448-453Forecasting China′s Stock Market Volatility UsingNon-Linear GARCH ModelsWEI Wei-xianI nstitute of Finance,X iamen University,X iamen361005,ChinaAbstract: T his paper s tu dies th e performance of th e GARC H model and tw o of its non-linearmodifications to for ecast China′s week ly s tock market volatility.T he models are th e Quadr atic GARCH an dthe Glos ten,J agannathan and Runk le m odels w hich have proposed to des cribe the often ob ser ved negativesk ew ness in stock market in dices.W e find that th e QGARCH model is best w hen the es timation s am ple doesnot contain extreme obs ervations an d that th e GJ R model can not be recomm ended for forecastin g.Keywords: China′s stock market;forecas tin g volatility;non-linear GARC H1 IntroductionA stylized fact of financial time series is that aberrant observations seem to cluster in the sense that there ar e periods w here v olatility is larg er than in other periods.T ypically, these volatile periods co rrespond to m ajo r(eco no mic)events such as sto ck market crashes and oil cr ises.Although mo st evidence in empirical finance indicates that return on financial assets seem unforecastable at short ho rizons(see e.g.Gr ang er,1992,fo r a recent survey),the current consensus is that the v ar iance of returns can be predicted using particular time series m odels.Within this class of mo dels,the Generalized Auto gressive Conditio nal Heteroscedasticity(GARCH)mo del proposed by Eng le(1982)and Bollerslev (1986)seems to be the m ost successful(see Bollerslev,Chou and Kr oner,1992,for a survey(cf.GARCH applications).Roug hly speaking,in a GARCH pr ocess the error variances can be modeled by an Auto gressive M oving Average(ARMA)ty pe process.A useful feature o f the GARCH m odel is that it can effectively remov e the excess kurtosis in returns.A further stylized fact is that the distributio n o f the r eturns can be skew ed.For example,for some stock market indices,returns are skew ed to the left,i.e.,there are mor e neg ative than po sitive outlying observatio ns.The intrinsically sym metric GARCH model canno t cope w ith such skew ness and,hence,o ne can expect that forecasts and fo recast error variances fr om a GARCH m odel m ay be biased for skew ed time series.Received date:July14,1999Recently ,a few modifications to the GARCH m odel have been proposed ,w hich ex plicitly take acco unt o f skew ed distributions .In this paper ,w e consider tw o such modifications ,Quadratic GA RCH model (QGARCH )proposed by Engle and Ng (1993)(see also Sentana (1995)for a recent discussion o f QGARCH mo dels ),and the advocated in Glosten,Jag annathan and Runkle,1992,to be abbr ev iated as GJR.An alter native model that can g enerate skew ed time series patterns is the so -called Exponential GARCH model (EGARCH )proposed by Nelso n (1990).Altho ug h w e have considered this mo del as a po ssibly useful candidate for our pur poses,it w as fo und that not to be very useful for repeated fo recasting exercises.Given the latter pur pose of r epeatedly specify ing and estimating GARCH m odels ,an obvious r equirement o f these m odels is that the estimation metho d is reasonably sim ple and that parameter co nv erg ence o ccurs reaso nably quick.Unfortunately ,it has been our ex perience w hile running the estim ation procedures that param eter estim ation o f the EGARCH m odel can be tedious .In fact ,in alm ost all cases ,no quick convergence was obtained.Only when w e w er e able to g enerate a particular set of starting -values w ith precision 8digits could w e obtain converg ence .The focus is the out -of-sample forecasting perform ance of these tw o non-linear mo dels relative to the standard GARCH m odel,and o n the perfo rmance of all three models relative to the simple random w alk forecasting schem e .We limit our analy sis to fo recasting volatility and not the mean of the tim e series.In the nex t sectio n we present the m odels used in o ur mo delling and for ecasting exercise .In the third section w e discuss China ′s sto ck market data w e use in our empirical study.In the fo ur th sectio n w e discuss som e within-sam ple estimation results.In the fifth section we ev aluate the forecasting performance of the GARCH,QGA RCH and GJR models as w ell as the Random Walk model .In the final section w e present conclusions .2 GARCH ModelsConsider a stock market composite index I t and its return r t ,w hich w e co nstr uct as r t =ln(I t )-ln(I t -1).In this paper the index t denotes w eekly o bser vatio ns.Although hig h order mo dels are so metim es found to be useful,the do minant empirical m odel fo r r t is the auto -regression of order p w ith GARCH (1,1)disturbances (AR (p )-GARCH (1,1)),w hich can be expressed asp (B )r t = + t ,w ith p (B )=1- 1B -…- p Bp t ~N (0,h t )h t =!+∀ 2t -1+#h t -1(1)w here B is backward shift operator defined by B k x t =x t -k .The parameter reflects aco nstant term ,w hich in practice is typically estimated to be close or equal to zero.Further more ,p is usually 0or small ,sugg esting that there ar e usually no o pportunities to fo recast r t fro m its ow n past.It is assum ed that the solutions of the characteristic equation (z )=0lie o utside the unit circle and that !,∀,#>0and ∀+#<1(see Bollerslev ,1986).All o dd m oments oft in mo del (1)equal to zero ,and hence t and r t ar e symm etric 449W EI W ei -x ian Fo r ecasting China ′s St ock M ar ket V olatility U sing …450Jo ur nal of Systems Science and Sy st ems Eng ineering V ol.9N o.4 time series w ith fat tails.For sto ck market time series,how ever,r t m ay display significant neg ative skew ness.This em pirical stylized result seems to be due to the fact that stock market crashes o ccur m ore frequently and mo re quickly than stock mar ket boo ms,and that the absolute size of crashes is much larger.T w o simple classes of models that cope w ith skew ed returns are the Quadratic GARCH model proposed by Eng le and Ng(1993)and the so-called GJR m odel,advocated by Glo sten,Jag annathan and Runkle(1992).The QGARCH m odel differs from model(1)byh t=!+∀(t-1-∃)2+#h t-1(2) When∃takes a positive value,it is clear fro m model(2)that a negative t-1v alue has a larger impact o n h t.The GJR m odel is also similar to mo del(1),but now the h t process is given byh t=!+∀2t-1+%D t-12t-1+#h t-1(3) w here D t-1is a dummy variable which takes a value of1w hen t-1<0and value o f0w hen t-1 0.Sim ilar to the QGARCH mo del,w hen%>0,neg ative shocks w ill have a larg er impact on h t than positive shocks.Stationary and stability of these mo dels w er e discussed in relevant references.T he QGA RCH and GJR models can improv e upon the standard GARCH since they can cope w ith negative(or positive)skew ness,the latter depending on the sign o f the additional param eter.3 The Data and Research MethodThe data w e analyze in this paper ar e weekly o bser ved indices for Shang hai Stock Exchang e Composite(HSEC)and Shenzhen Stock Ex change Com po nent(ZSEC).The data span7year s,w ith the first o bser vatio n being w eek1in1992and the last observation being w eek52in1998.In o ur case,the w eekly o bser vations are taken as the values that are reco rded on Fridays′closing price.A summary of som e characteristics of the r t series is giv en in Table1.T he number of observation equals to355for all tw o stock m arkets.Sam ple statistics for the two stock returns(Table1)highlight the follow ing:(a)retur n display positive skew ness fo r all indices.The null hypothesis co efficient conforming to the norm al distribution value o f zero is alway s rejected at5percent.(b)Return display also ex cess kurtosis.In this case,the null hy pothesis o f coefficients confo rming to the no rmal value of thr ee is r ejected for all indices.T hus,mo st returns are leptokurtic,that is,their distribution hav e thicker (flatter)tails than nor mal distribution.(c)T he hypothesis of(unco nditional)norm ality is alw ay s rejected by Jar que-Bera test,confo rming to the results based either on skewness or on kurto sis.The appro ach taken in this paper is as follow s.Since our main g oal is to evaluate the volatility fo recasting perfo rmance o f three GARCH m odels,we w ish to consider a reasonably larg e hold-out sample.We thus choo se o bserv ations fiv e years of o bser vatio ns to estim ate various mo del parameter s.Furthermor e,since it is no t a priori assumed that one model necessar ily dominates other m odels over the w hole sample,w e r epeat ourmodelling and fo recasting exercise for different sub -sample .We thus fit the mo dels to a sam ple o f five y ears ,g enerate a one -step -ahead fo recast ,delete the fir st observ ation from the sam ple and add the nex t one,and gener ate again a one-head-forecast.In order to evaluate possibly changing patterns over y ears,w e evaluate forecasting perform ance for the years 1997to 1998.This results in an ev aluation tw o tim es about 52for ecasts .Since w e w ish to m inimize the impact of outlying observations on fo recast evaluation,w e use the M edian o f Squar ed Erro r (M EDSE)instead of the usual M ean Squared Error (M SE).Table 1 Summary statistics of data on returns .The sample covers weeklyobservations for the years 1992to 1998Stock marketN M ean (×10-4)Variance (×10-4)Skew nes s Excess Kurtos is Jarque-Bera tes t for norm ality Sh angh ai35538.76082.792 4.602835.6273522545.67S henzhen 35531.55340.449 1.1802 6.072871251.85 Notes:T he week ly data concern Friday close obs ervations.T he n otation (×10-4)mean s that th e reported value sh ou ld be multiplied by 10-4.The Jar qu e -Ber a test for norm ality dis tr ibution as &22(2degrees of freedom ).Th e critical value for the null hypothesis of n ormal distrib ution is 5.99at the 5percent s ignificance level.Higher tes t values r eject the null hypothes is.4 Within Sample EstimationIn this section,w e present w ithin-sam ple estimation results to give an idea of the possible usefulness of non-linear GARCH models.T o save space w e consider the sample co vering the perio d 1992to 1996,which g ives an indicatio n o f the ty pical estim ated parameter values .In T able 2w e report the relevant parameter estim ates fo r the GARCH model (1).Next to the parameter estimates,we repor t the value of the AIC and the value of the LogLikehoo d (LnL ).We present these AIC and LnL values to com pare mo dels (1),(2),and (3).We further report the values o f Box -Pierce statistics fo r t /h 0.5t and 2t /h t in orderto check the em pirical validity of the models.It is clear fro m Table 2that the parameters ∀and #in the GARCH (1,1)m odel are usually sig nificant at the 5%level,and hence that the constant variance mo del can be r ejected ,at least w ithin sam ple .Fur thermor e ,for two cases the sum of the ∀and #parameters is close to unity.Ho w ever,for no index do w e observe that ∀+#>1.In T able 3,w e report som e estimation for the non -linear variants of the GARCH (1,1)m odel .We r eport the estim ates for the ∃parameter in the QGARCH m odel and the %param eter in the GJR m odel.Although the r esults should be interpr eted w ith care since not all no n-norm ality may have been r em oved,the t -ratios in T ables 3indicate that the ∃and %par am eters ar e significant at the 10%lev el for the two stock m arket indices .The po ssible usefulness o f non-linear GARCH mo del seems to be confir med by the LnL v alues,altho ug h the AIC values do no t sug gest a clear fav orite.U nr eported estim ates of ∃and %in other samples sho ws that these parameters are not alw ays relev ant .Hence ,the relev ance of these parameter s can depend on one or tw o observations being including in or 451W EI W ei -x ian Fo r ecasting China ′s St ock M ar ket V olatility U sing …deleted from the sample .Table 2 Estimation results f or GARCH models for 1992~1996Parameter estimates Diagnostics IndexP !(×10-2)∀#AIC LnL Q 1(10)Q 2(10)HSEC00.5150.4570.523-7.08441.2113.6910.32(2.05)(2.15)(3.30)ZS EC 10.3320.1910.678-5.76318.73 5.77 3.29(1.30)(1.30)(3.75) Notes :Th e model (1)is estimated ,t -ratio ar e given in parentheses .Estimation is carried using my own program w ritten in TS P international 4.4.T he d iagnos tics are the Akaike Information Cr iterion (AIC ),w hich will to be u sed to compare th ese models w ith thos e in T able 3,th e LogLik ehood (L nL )calculated for s imilar reas ons ,and Q 1(10)an d Q 2(10),w hich denote Box-Pierce tes t statis tics for th e s ignificance of res idual correlation u p to 10in the estimated s caledresiduals t /h 0.5tand the s qu ares of these residu als,res pectively.Th e AIC is calculated so that the model w ith the sm alles t AIC valu es is preferred.Table 3 Estimation results for non -linear GARCH models f or 1992~1996IndexQGARCH Diagnostics ∃(×10-1)AIC LnL %AIC LnL HSEC0.087(2.79)-7.05456.710.357(1.92)-7.00441.58 ZS EC 0.115(2.67)-5.77 481.960.204(1.85)-5.76480.40 Notes :T he QGARCH model is given by ∋(B )r t = + t , t ~N (0,h t ),and h t is given by equation (2).GJ R model by ∋(B )r t = + t , t ~N (0,h t ),and h t by equation (3),w her e D t -1is a du mmy variable w hich tak es a value of 1w hen t -1<0an d value of 0w hen t -1 0,and w here ∋(B )is an au toregressive polynominal of order p and r t is the w eekly observed retu rn ser ies.5 Out -of Sample ForecastingTo evaluate the ability o f non -linear v ar iants o f GARCH models to adequately forecast the volatility in financial time series,w e need a measure of the ′true v olatility ′.As is standard in related studies on forecasting volatility (see Day and Lew is,1992;Pag an and Schw er t,1990),w e use the measure w t ,defined byw t =(r t -r -)2(4)w here r -is the averag e return o ver the last fiv e y ears of w eekly observations .A s a measure of the one-step-ahead fo recast err or u t +1,w e use u t +1=w t +1-h t +1(5)w here ht +1is generated using the h t equations in (1),(2),and (3)w ith the estimates for the parameters !,∀,#,∃and %substituted .To com pare the for ecasts fro m the GARCH models w ith a naive no -change fo recast ,w e also evaluate the forecast erro rs fo r the Rando m W alk (RW)m odel,fo r w hich forecasts are giv en by ht +1=w t .No tice that w e calculate o ne -step -ahead forecasts ,i .e .,w e use the estimated models to forecast fo r t +1w hile the m odels are calibrated for fiv e years of observations until and including t .In T able 4w e repor t the M EDSE for the y ears 1997and 1998.The results indicate that the QGARCH m odel is pr efer red in 2of the 4cases,and that the RW,GARCH,and 452Jo ur nal of Systems Science and Sy st ems Eng ineering V ol .9N o .4 GJR models are preferred in 1,1,0cases ,respectively .That is ,the QGARCH model outperforms the o ther models in 2of the 4cases .The results for the GJR m odel sugg est that it is not a useful too l for fo recasting China ′s stock market volatility.Table 4 Out -of -sample f orecasting performance of GARCH ,QGARCH ,GJR andrandom walk models f or the volatility of stock indicesIn dexM odel Forecasting period 19971998HSEC GARCH1.7852.858QGARCH0.812 1.461GJ R4.52362.54RW1.047 3.278ZSE C GARCH0.7570.919QGARCH0.655 1.024GJ R2.1458.874RW 0.6450.983 Notes:As a m easure of ′true volatility ′w e u se (r t -r -)2,w here r -is th e average retu rn over t -1,t -2,…,t -five years.T he models are estimated for s am ples for five years,w h ere in each new s am ple the first obs ervation is deleted an d the n ew observation is included ,and on e -step ah ead for ecasts are gen erated for 1997an d 1998.Each cell contain s the M edian of S quar ed Errors (×10-7).6 ConclusionsWe apply non-linear GARCH models to for ecasting China ′s stock market volatility for the first time.For ecasting r esults for China ′s tw o weekly stock market indices show that the QGARCH m odel can significantly im pro ve on the linear GARCH m odel and the random w alk mo del .T he GJR model ,o n the o ther hand ,canno t be recom mended .References :[1] Bollerslev T.Generalized autogr ess ive conditional heteros kedasticity.J ournal of Econ ometrics,1986,31:307~327.[2] Bollers lev T,Ch ou R,Kroner K.ARCH modellin g in finance:a review of the theory and emp irical evidence.Journal of Econ ometrics ,1992,52:5~59.[3] Day T ,Lew is C .Stock m ark et volatility and th e information content of s tock index options .Journal ofEconometrics ,1992,52:267~287.[4] En gle R F.Autogressive conditional heteros kedasticity w ith estimates of the variance of UK inflation.Econometrica,1982,50:987~1008.[5] Engle R F ,Ng V .M easur ing and tes tin g the impact of new s on volatility .J ournal of Finance ,1993,48:1749~1778.[6] Glosten L,Jagann athan R,Runk le D.On the relation betw een the expected valu e and th e volatility of nominalexces s return on stock s.Journ al of Finance,1992,46:1779~1801.[7] Granger C W J.For ecasting stock m ark et prices :Les sons for forecasters.International J ournal of Forecas tin g,1992,8:3~13.[8] Nels on D .Conditional h eteroskedas ticity in ass et returns :a new app roach .Econometrica ,1990,59:347~370.[9] Pagan A R,S chw ert G W.Alternative m od els for conditional s tock market volatility.J ournal of Econometrics ,1990,45:267~290.[10] Sentana E.Quadr atic ARCH m od els.Review of Econom ic Studies,1995,62:639~661.453W EI W ei -x ian Fo r ecasting China ′s St ock M ar ket V olatility U sing …。
Forecasting

ForecastingWhy forecast?Features Common to all Forecasts∙Conditions in the past will continue in the future∙Rarely perfect∙Forecasts for groups tend to be more accurate than forecasts for individuals ∙Forecast accuracy declines as time horizon increasesElements of a Good Forecast∙Timely∙Accurate∙Reliable (should work consistently)∙Forecast expressed in meaningful units∙Communicated in writing∙Simple to understand and useSteps in Forecasting Process∙Determine purpose of the forecast∙Establish a time horizon∙Select forecasting technique∙Gather and analyze the appropriate data∙Prepare the forecast∙Monitor the forecastTypes of Forecasts∙Qualitativeo Judgment and opiniono Sales forceo Consumer surveyso Delphi technique∙Quantitativeo Regression and Correlation (associative)o Time seriesForecasts Based on Time Series Data∙What is Time Series?∙Components (behavior) of Time Series datao Trendo Cycleo Seasonalo Irregularo Random variationsNaïve MethodsNaïve Forecast – uses a single previous value of a time series as the basis of a forecast.Techniques for Averaging∙What is the purpose of averaging?∙Common Averaging Techniqueso Moving Averageso Exponential smoothingMoving AverageExponential SmoothingTechniques for TrendLinear Trend Equationline the of slope at of value pe riod time for fore cast from pe riods time of numbe r spe cifie d =====b ty a ty t t where t t 0:Curvilinear Trend Equationline the of slope at of value pe riod time for fore cast from pe riods time of numbe r spe cifie d =====b ty a ty t t where t t 0:Techniques for Seasonality∙ What is seasonality?∙ What are seasonal relatives or indexes?∙ How seasonal indexes are used:o Deseasonalizing datao Seasonalizing data∙ How indexes are computed (see Example 7 on page 109)Accuracy and Control of ForecastsMeasures of Accuracyo Mean Absolute Deviation (MAD)o Mean Squared Error (MSE)o Mean Absolute Percentage Error (MAPE) Forecast Control Measureo Tracking SignalMean Absolute Deviation (MAD)Mean Squared Error (or Deviation) (MSE)Mean Square Percentage Error (MAPE)Tracking SignalProblems:2 – Plot, Linear, MA, exponential Smoothing5 – Applying a linear trend to forecast15 – Computing seasonal relatives17 – Using indexes to deseasonalize values26 – Using MAD, MSE to measure forecast accuracyProblem 2 (110)National Mixer Inc., sells can openers. Monthly sales for a seven-month period were as follows:(a) Plot the monthly data on a sheet of graph paper.(b) Forecast September sales volume using each of the following:(1) A linear trend equation(2) A five-month moving average(3) Exponential smoothing with a smoothing constant equal to 0.20, assuming March forecast of19(000)(4) The Naïve Approach(5) A weighted average using 0.60 for August, 0.30 for July, and 0.10 for June(c) Which method seems least appropriate? Why?(d) What does use of the term sales rather than demand presume?EXCEL SOLUTION(a) Plot of the monthly dataHow to superimpose a trend line on the graph∙Click on the graph created above (note that when you do this an item called CHART will appear on the Excel menu bar)∙Click on Chart > Add Trend Line∙Click on the most appropriate Trend Regression Type∙Click OK(b) Forecast September sales volume using:(1) Linear Trend Equation∙Create a column for time period (t) codes (see column B)∙Click Tools > Data Analysis > Regression∙Fill in the appropriate information in the boxes in the Regression box that appearsCoded time periodSales dataCoded time period(2) Five-month moving average(3) Exponential Smoothing with a smoothing constant of 0.20, assuming March forecast of 19(000)∙Enter the smoothing factor in D1∙Enter “19” in D5 as forecast for March∙Create the exponential smoothing formula in D6, then copy it onto D7 to D11(4) The Naïve Approach(5) A weighted average using 0.60 for August, 0.30 for July, and 0.10 for JuneProblem 5 (110)A cosmetics manufactur er’s marketing department has developed a linear trend equation that can be used to predict annual sales of its popular Hand & Foot Cream.y t =80 + 15 twhere: y t = Annual sales (000 bottles) t0 = 1990(a) Are the annual sales increasing or decreasing? By how much?(b) Predict annual sales for the year 2006 using the equationProblem 15 (113)Obtain estimates of daily relatives for the number of customers at a restaurant for the evening meal, given the following data. (Hint: Use a seven-day moving average)Excel Solution∙Type a 7-day average formula in E6 ( =average(C3:c9) )∙In F6, type the formula =C6/E6∙Copy the formulas in E6 and F6 onto cells E7 to E27∙Compute the average ratio for Day 1 (see formula in E12)∙Copy and paste the formula in E12 onto E13 to E18 to complete the indexes for Days 2 to 7Problem 17 (113) – Using indexes to deseasonalize valuesNew car sales for a dealer in Cook County, Illinois, for the past year are shown in the following table, along with monthly (seasonal) relatives, which are supplied to the dealer by the regional distributor.(a) Plot the data. Does there seem to be a trend?(b) Deseasonalize car sales(c) Plot the deseasonalized data on the same graph as the original data. Comment on the two graphs.Excel Solution(a) Plot of original data (seasonalized car sales)(b) Deseasonalized Car Sales(c) Graph of seasonalized car sales versus deseasonalized car salesProblem 26 (115) – Using MAD, MSE, and MAPE to measure forecast accuracyTwo different forecasting techniques (F1 and F2) were used to forecast demand for cases of bottled water. Actual demand and the two sets of forecasts are as follows:(a) Compute MAD for each set of forecasts. Given your results, which forecast appears to be the mostaccurate? Explain.(b) Compute MSE for each set of forecasts. Given your results, which forecast appears to be the mostaccurate? Explain.(c) In practice, either MAD or MSE would be employed to compute forecast errors. What factors might leadyou to choose one rather than the other?(d) Compute MAPE for each data set. Which forecast appears to be more accurate?Excel Solution。
pytorch-forecasting deepar 用法-概述说明以及解释
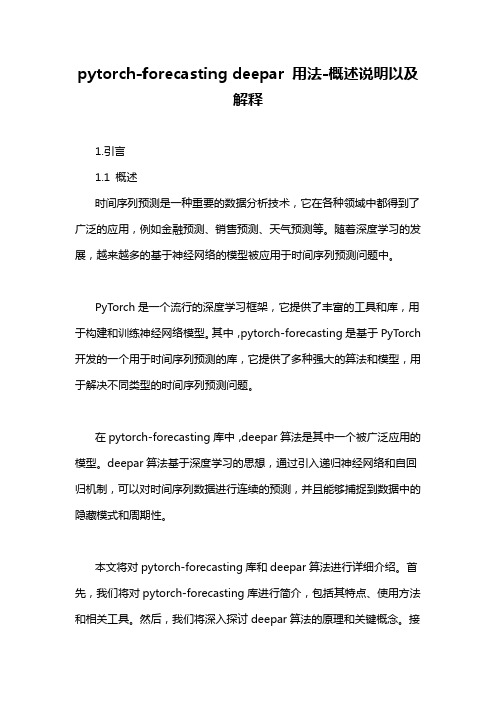
pytorch-forecasting deepar 用法-概述说明以及解释1.引言1.1 概述时间序列预测是一种重要的数据分析技术,它在各种领域中都得到了广泛的应用,例如金融预测、销售预测、天气预测等。
随着深度学习的发展,越来越多的基于神经网络的模型被应用于时间序列预测问题中。
PyTorch是一个流行的深度学习框架,它提供了丰富的工具和库,用于构建和训练神经网络模型。
其中,pytorch-forecasting是基于PyTorch 开发的一个用于时间序列预测的库,它提供了多种强大的算法和模型,用于解决不同类型的时间序列预测问题。
在pytorch-forecasting库中,deepar算法是其中一个被广泛应用的模型。
deepar算法基于深度学习的思想,通过引入递归神经网络和自回归机制,可以对时间序列数据进行连续的预测,并且能够捕捉到数据中的隐藏模式和周期性。
本文将对pytorch-forecasting库和deepar算法进行详细介绍。
首先,我们将对pytorch-forecasting库进行简介,包括其特点、使用方法和相关工具。
然后,我们将深入探讨deepar算法的原理和关键概念。
接着,我们将介绍deepar算法在时间序列预测中的应用场景,并分析其优势和适用性。
最后,我们将具体讲解deepar算法的使用方法,包括数据准备、模型构建、训练和预测等步骤。
通过本文的学习,读者将能够了解pytorch-forecasting库和deepar 算法的基本原理和使用方法,并且掌握如何应用这些技术解决实际的时间序列预测问题。
此外,我们还将对pytorch-forecasting的deepar算法进行评价,并提出可能的改进方向,以期进一步提升其预测性能和应用范围。
1.2 文章结构本文将详细介绍pytorch-forecasting deepar 的用法和应用。
文章分为三大部分:引言、正文和结论。
在引言部分,我们将首先概述本文要探讨的主题,并介绍pytorch-forecasting deepar 的背景和意义。
P190阅读理解43Forecasting Methods天气预报的方法
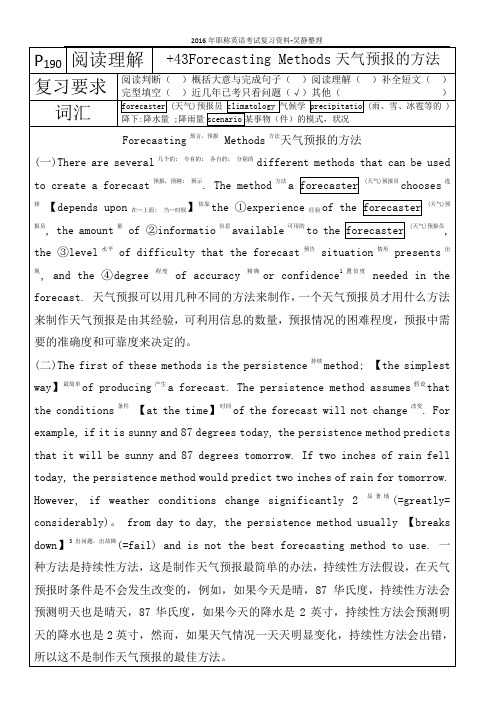
预示
. The method 方法 a forecaster (天气)预报员 chooses 选择
经验
】依靠 the ①experience
of the forecaster (天气)预报员,
the amount 量 of ②informatio 信息 available 可用的 to the forecaster (天气)预报员, the ③ level 水平 of difficulty that the forecast 预告 situation 情形 presents 出现, and the ④degree
Forecasting 预言,预报 Methods 方法天气预报的方法 (一)There are several 几个的;
专有的; 各自的; 分别的
different methods that can be used
to create a forecast 预报,预测; 【depends upon
在…上面; 当…时候
2016 年职称英语考试复习资料-吴静整理
P190 阅读理解 +43Forecasting Methods 天气预报的方法 阅读判断( )概括大意与完成句子( )阅读理解( )补全短文( ) 复习要求 完型填空( )近几年已考只看问题(√)其他( ) forecaster (天气)预报员 climatology 气候学 precipitatio (雨、雪、冰雹等的 ) 词汇 降下:降水量 ;降雨量 scenario 某事物(件)的模式,状况
weather in this forecast will behave the same as it did in the past. The analog method is difficult to use because it is virtually to find a predict analog. Various 各种各样的;
天气预报英语介绍
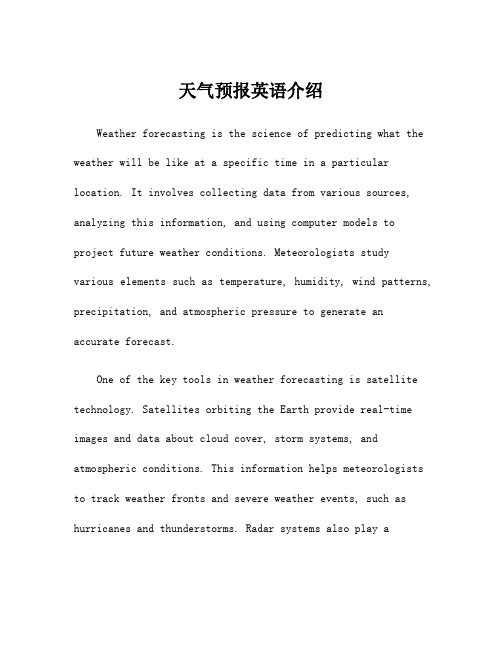
天气预报英语介绍Weather forecasting is the science of predicting what the weather will be like at a specific time in a particular location. It involves collecting data from various sources, analyzing this information, and using computer models to project future weather conditions. Meteorologists studyvarious elements such as temperature, humidity, wind patterns, precipitation, and atmospheric pressure to generate an accurate forecast.One of the key tools in weather forecasting is satellite technology. Satellites orbiting the Earth provide real-time images and data about cloud cover, storm systems, and atmospheric conditions. This information helps meteorologists to track weather fronts and severe weather events, such as hurricanes and thunderstorms. Radar systems also play acrucial role, enabling forecasters to observe precipitationin real-time and provide warnings for severe weather.Meteorological data is typically collected from a variety of sources, including weather stations, buoys in oceans, and aircraft. These observations are gathered in a centralized location and analyzed using sophisticated computer algorithms. Prediction models simulate weather behavior based on current conditions and historical data, allowing forecasters to make educated predictions about the weather.Weather forecasts are usually communicated throughvarious platforms, including television, radio, the internet, and mobile applications. They typically include information about temperature, chances of precipitation, wind speed, and potential severe weather conditions. Most forecasts covershort-term periods, such as daily or weekly forecasts, but some models can predict weather conditions several weeks in advance.One of the challenges in weather forecasting is the inherent unpredictability of the atmosphere. Weather systems are complex and can change rapidly, making precise long-term predictions difficult. Forecasters often use a range of forecasts to provide a more comprehensive view, emphasizing the possibility rather than certainty of certain conditions.Advancements in technology have significantly improved the accuracy of weather forecasts over the years. With the introduction of supercomputers and sophisticated modeling techniques, meteorologists can analyze vast amounts of data more efficiently, enhancing the reliability of predictions. Despite this progress, it is essential for the public to understand that forecasts are not infallible, and sudden changes in the weather can occur.Overall, weather forecasting is an essential service that impacts daily life, from agriculture to transportation, and even emergency management during natural disasters. As ourunderstanding of meteorology continues to evolve, so too will the methods and accuracy of weather forecasting, helping individuals and communities better prepare for the ever-changing weather.。
forecasting
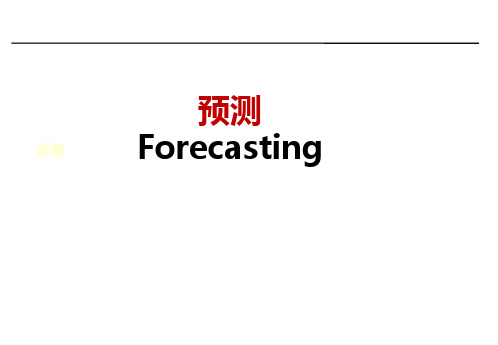
Y轴截距
斜率
Y = a + bX
因变量(反应变量) 自变量(解释变量)
线性回归模型 (Linear Regression)
Y
回归方程 Y = a + bX
因变量
X 自变量
预测总是不准确的 Forecast is always wrong!
预测的结果很少能够与实际完全相符; 大多数预测方法假设系统在一段时间内保持稳定;
产品需求数量
第1年
第2年
第3年
第4年
移动平均法(Moving Average)
MA 是一列算术平均值 主要用于数据序列不存在趋势或趋势不明显的情形
通常用于平滑数据波动, 能够反映数据在一段时间内的总体走 势
计算公式: 前n期需求总和 / n
加权移动平均(Weighted Moving Average)
定量预测
用于市场形势稳定或存在大 量历史数据的情形
– 新产品
– 新技术 主要利用决策者的直觉及经 验进行预测 – 如预测商品网络销量
– 已有产品
– 已存在的技术 主要利用数量方法进行预测
– 如预测彩色电视机的销量
定性方法(Qualitative Methods)
各部门主管集体讨论法
时间序列模型
因果模型
移动平均
指数平滑
趋势外推
线性回归
时间序列预测(Time Series)
时间序列即一列均匀分布的数据点 – 数据点可通过观察多个时间周期的反应变量获得 预测仅依赖于过去值 – 过去和当前发挥作用的因素变量在未来将继续施加影响
时间序列预测(Time Series)
周期 (Cycle) 趋势季节 (Season)
专业英语气象科技英语第三课 气象预报

Serve as 充当,作为
P1①National Meteorological Services perform a variety of activities in order to provide weather forecasts. ②The principal ones are data collection, the preparation of basic analyses and prognostic charts of short-and long-term forecasts for the public as well as special services for aviation, shipping , agricultural and other commercial and industrial users, and the issuance of severe weather warnings.
stations for severe weather . ②Under the World
Weather Watch (WWW) program, synoptic reports are made at some 4,000 land stations and by 7,000
ships. ③There are about 700 stations making
P7: During the first half of the century, short-range forecasts were based on synoptic principles, empirical rules and extrapolation of pressure changes.
IBM SPSS Forecasting 28使用手册说明书
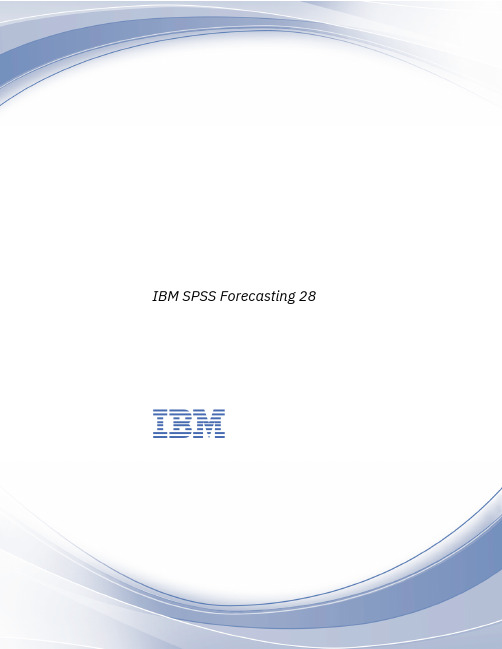
时间序列建模器............................................................................................................................................ 3 为专家建模器指定选项............................................................................................................................ 4 自定义的指数平滑法模型........................................................................................................................ 5 自定义的 ARIMA 模型............................................................................................................................. 6 输出(O).................................................................................................................................................... 7 保存模型预测和模型指定项.................................................................................................................... 9 选项....................................................................................................................................................... 10 TSMODEL 命令的附加功能................................................................................................................... 10
- 1、下载文档前请自行甄别文档内容的完整性,平台不提供额外的编辑、内容补充、找答案等附加服务。
- 2、"仅部分预览"的文档,不可在线预览部分如存在完整性等问题,可反馈申请退款(可完整预览的文档不适用该条件!)。
- 3、如文档侵犯您的权益,请联系客服反馈,我们会尽快为您处理(人工客服工作时间:9:00-18:30)。
“Prediction is very difficult, especially if it's about the future.”
Nils Bohr
Objectives
• Give the fundamental rules of forecasting
• Calculate a forecast using a moving average, weighted moving average, and exponential smoothing • Calculate the accuracy of a forecast
Actual demand (past sales) Predicted demand
What’s Forecasting All About?
From the March 10, 2006 WSJ:
Ahead of the Oscars, an economics professor, at the request of Weekend Journal, processed data about this year's films nominated for best picture through his statistical model and predicted with 97.4% certainty that "Brokeback Mountain" would win. Oops. Last year, the professor tuned his model until it correctly predicted 18 of the previous 20 best-picture awards; then it predicted that "The Aviator" would win; "Million Dollar Baby" won instead.
In the simple moving average models the forecast value is At + At-1 + … + At-n Ft+1 = n
t
is the current period.
Ft+1 is the forecast for next period n is the forecasting horizon (how far back we look),
• Cyclical elements
• Autocorrelation • Random variation
Some Important Questions
• What is the purpose of the forecast? • Which systems will use the forecast? • How important is the past in estimating the future? Answers will help determine time horizons, techniques, and level of detail for the forecast.
Quantitative forecasting methods
Time Series: models that predict future demand based on past history trends Causal Relationship: models that use statistical techniques to establish relationships between various items and demand Simulation: models that can incorporate some randomness and non-linear effects
Example: Mercedes E-class vs. M-class Sales
Month
Jan Feb Mar Apr
E-class Sales
23,345 22,034 21,453 24,897
M-class Sales
-
May
Jun Jul
23,561
22,684 ?
?
Question: Can we predict the new model M-class sales based on the data in the the table?
• The moving average model uses the last t periods in order to predict demand in period t+1. • There can be two types of moving average models: simple moving average and weighted moving average
• The moving average model assumption is that the most accurate prediction of future demand is a simple (linear) combination of past demand.
Time series: simple moving average
5-month MA forecast 3-month MA forecast
A
is the actual sales figure from each period.
Example: forecasting sales at Kroger
Kroger sells (among other stuff) bottled spring water
Month Jan Feb Mar Apr
Types of forecasting methods
Qualitative methods Rely on subjective opinions from one or more experts.
Quantitative methods Rely on data and analytical techniques.
What is forecasting?
Forecasting is a tool used for predicting future demand based on past demand information.
Why is forecasting important?
Demand for products and services is usually uncertain. Forecasting can be used for…
How should we pick our forecasting model?
1. Data availability
2. Time horizon for the forecast
3. Required accuracy 4. Required Resources
Time Series: Moving average
What is forecasting all about?
Demand for Mercedes E Class
We try to predict the future by looking back at the past
Jan Feb Mar Apr May Jun Jul Aug
Time
Predicted demand looking back six months
Bottles 1,325 1,353 1,305 1,275
May
Jun Jul
1,210
1,195 ?
What will the sales be for July?
What if we use a 3-month simple moving average?
FJul =
AJun + AMay + AApr 3
Qualitative forecasting methods
Grass Roots: deriving future demand by asking the person closest to the customer. Market Research: trying to identify customer habits; new product ideas. Panel Consensus: deriving future estimations from the synergy of a panel of experts in the area. Historical Analogy: identifying another similar market. Delphi Method: similar to the panel consensus but with concealed identities.
Key issues in forecasting
1. A forecast is only as good as the information included in the forecast (past data)
2. History is not a perfect predictor of the future (i.e.: there is no such thing as a perfect forecast)
REMEMBER: Forecasting is based on the assumption that the past predicts the future! When forecasting, think carefully whether or not the past is strongly related to what you expect to see in the future…